Correlation between wearable inertial sensor data and standardised Parkinson’s disease axial impairment measures using machine learning
2022 IEEE 21st Mediterranean Electrotechnical Conference (MELECON)(2022)
摘要
Wearable sensors represent a valuable means for monitoring motion signs and symptoms of Parkinson’s disease (PD). In this paper, we explore the potential of a single inertial sensor to yield information correlated to the patient’s subjective perception of axial motion impairment during daily activities. This latter is expressed using as a relevant metric the sum of MDS-UPDRS items 2.11-2.13. Methods: thirty-one patients with PD were enrolled in this study, and asked to perform a timed-up-and-go test while wearing an inertial sensor on their thigh. Several time- and frequency-domain features were extracted from the inertial signals. They were fed to a random forest regression model for the prediction of the axial impairment metric. The model was optimized using 10-fold cross-validation and performance were assessed using leave-one-subject-out test. Results: Pearson correlation coefficient with the addressed metric of 0.76 (0.86) and mean absolute error of 1.70 (1.52) were obtained in patients under (not under) dopaminergic therapy. Moreover, moderate to strong correlations were found between the predicted score and some important disease progression, axial impairment, and motor performance metrics. Conclusion: a single wearable inertial sensor may be used for assessing motor disabilities of patients with PD.
更多查看译文
关键词
Parkinson’s disease,UPDRS,wearable inertial sensors,gait,prediction,machine learning,random forest
AI 理解论文
溯源树
样例
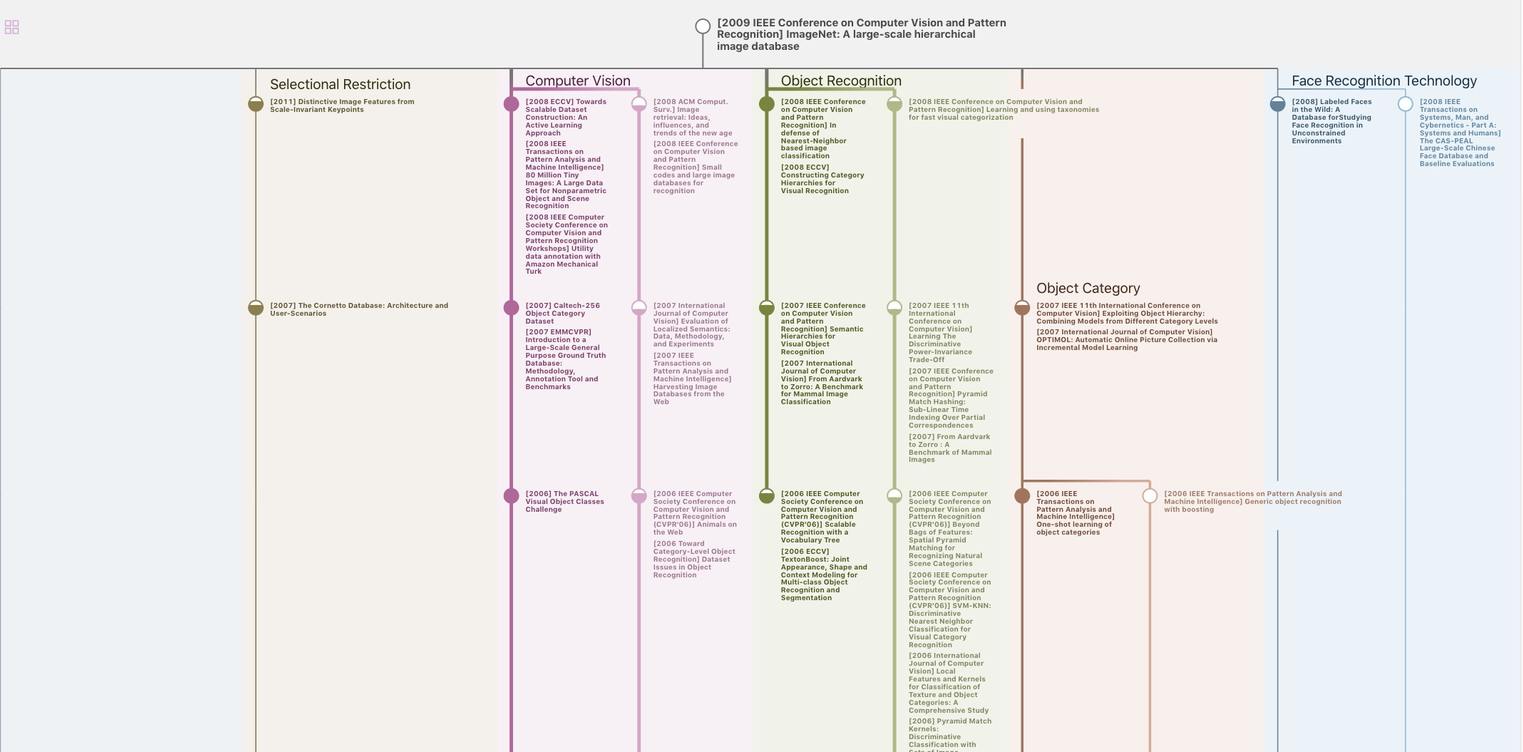
生成溯源树,研究论文发展脉络
Chat Paper
正在生成论文摘要