Application of Wavelet Characteristics and GMDH Neural Networks for Precise Estimation of Oil Product Types and Volume Fractions
SYMMETRY-BASEL(2022)
摘要
Given that one of the most critical operations in the oil and gas industry is to instantly determine the volume and type of product passing through the pipelines, in this research, a detection system for monitoring oil pipelines is proposed. The proposed system works in such a way that the radiation from the dual-energy source which symmetrically emits radiation, was received by the NaI detector after passing through the shield window and test pipeline. In the test pipe, four petroleum products-ethylene glycol, crude oil, gasoil, and gasoline-were simulated in pairs in different volume fractions. A total of 118 simulations were performed, and their signals were categorized. Then, feature extraction operations were started to reduce the volume of data, increase accuracy, increase the learning speed of the neural network, and better interpret the data. Wavelet features were extracted from the recorded signal and used as GMDH neural network input. The signals of each test were divided into details and approximation sections and characteristics with the names STD of A3, D3, D2 and were extracted. This described structure is modelled in the Monte Carlo N Particle code (MCNP). In fact, precise estimation of oil product types and volume fractions were done using a combination of symmetrical source and asymmetrical neural network. Four GMDH neural networks were trained to estimate the volumetric ratio of each product, and the maximum RMSE was 0.63. In addition to this high accuracy, the low implementation and computational cost compared to previous detection methods are among the advantages of present investigation, which increases its application in the oil industry.
更多查看译文
关键词
volume fraction, feature extraction, GMDH neural network, dual-energy gamma source
AI 理解论文
溯源树
样例
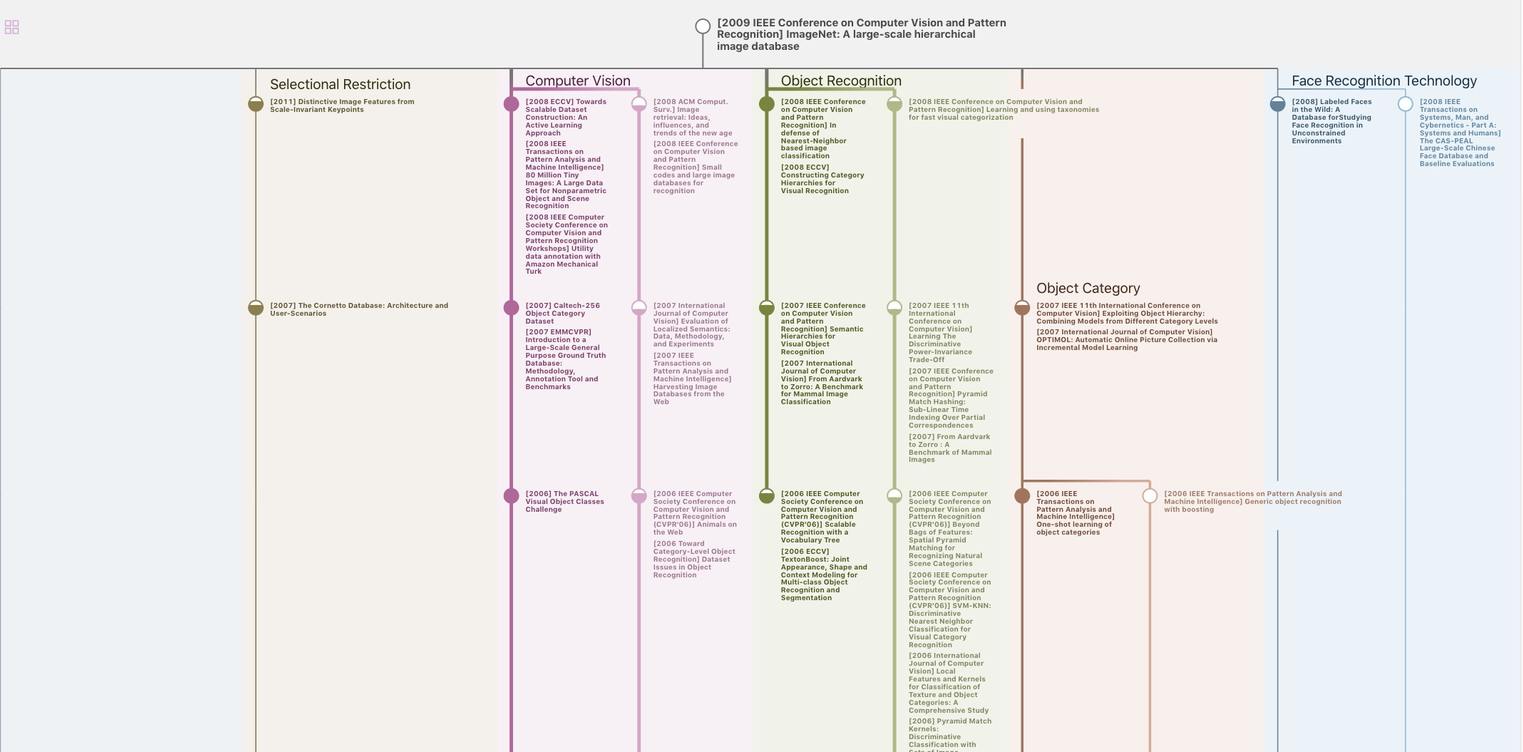
生成溯源树,研究论文发展脉络
Chat Paper
正在生成论文摘要