Optimized Sequence Dataset Generation for a Two-Stage Pipelined Microcontroller Instruction-Level Electromagnetic Information Leakage Analysis
IEEE ACCESS(2022)
摘要
With the prevalence of the Internet of Things (IoT) and microcontrollers (MCUs), the security issues of IoT and MCUs have become increasingly important. Side-channel analysis (SCA) is a major threat to such problems. One of the non-invasive SCAs is through electromagnetic information leakage (EM-leak) analysis. The author has developed a machine-instruction-level EM-leak analysis by neural network (NN) model. The NN model analysis needs a large dataset for training and validation. And the dataset should be complete and sufficient. The dataset sufficiency can be achieved by acquiring more data from the already proposed EM-leak measurement platform. However, the completeness issue becomes a challenge due to the pipelined architecture in the target MCU. In this paper, the completeness issue of a NN model dataset for the EM-leak analysis is addressed. Experiments show that the proposed algorithm can find an optimal solution. The contributions of this paper include: it successfully reduces a complex and practical SCA problem into a two-stage pipelined MCU individual EM-leak analysis sequence (2-EMAseq) description, proves that the 2-EMAseq problem has at least one optimal solution, uses this proof to develop an algorithm, and uses this algorithm to find a complete dataset is the target two-stage pipelined MCU dataset for NN model training/validation. Currently, the algorithm can only generate the optimal EM-dataset for two-stage pipelined MCUs. However, there are many MCUs with 4 similar to 8 pipeline stages. Whether there is an optimal solution when the stages are more than two is still an open question. And it needs to find proofs or derive heuristics in the future for such MCUs.
更多查看译文
关键词
EM information leakage, NN dataset generation, directed graph Eulerian trail
AI 理解论文
溯源树
样例
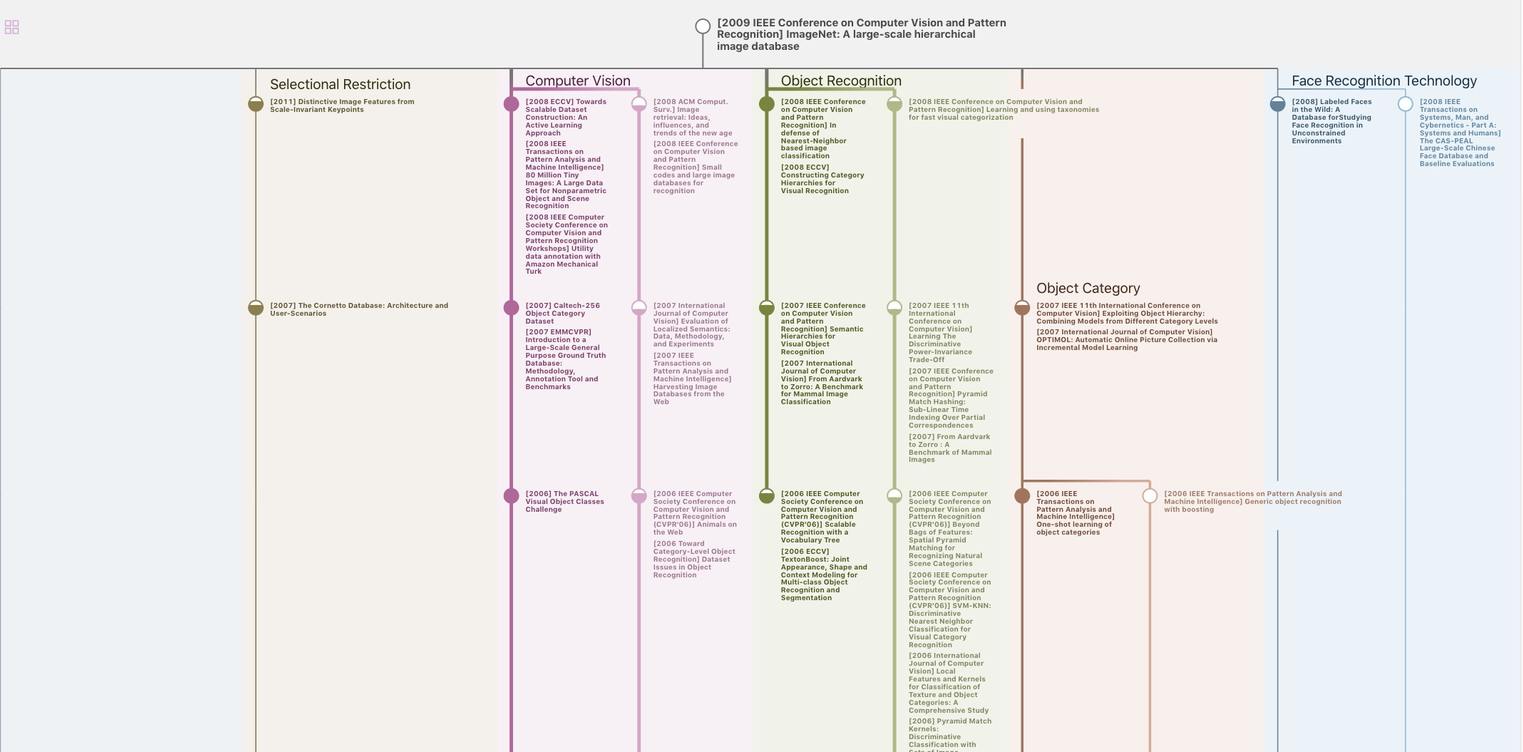
生成溯源树,研究论文发展脉络
Chat Paper
正在生成论文摘要