Deep Learning-Based Building Attribute Estimation from Google Street View Images for Flood Risk Assessment Using Feature Fusion and Task Relation Encoding
JOURNAL OF COMPUTING IN CIVIL ENGINEERING(2022)
摘要
Floods are the most common and damaging natural disaster worldwide in terms of both economic losses and human casualties. Currently, policymakers rely on data collected through labor-intensive and, consequently, expensive street-level surveys to assess flood risks. We propose a laborless and financially feasible alternative: a framework that can effectively and efficiently collect building attribute data without manual street surveys. By utilizing deep learning, the proposed framework analyzes Google Street View (GSV) images to estimate multiple attributes of buildings simultaneously-including foundation height, foundation type, building type, and number of stories-that are necessary for assessing flood risks. The proposed framework achieves a 0.177-m mean absolute error (MAE) for foundation height estimation and classification F1 scores of 77.96% for foundation type, 83.12% for building type, and 94.60% for building stories, and requires less than five days to predict the attributes of 0.8 million buildings in coastal Louisiana.
更多查看译文
关键词
Deep learning, Object recognition, Feature fusion, Multitask learning, Flood risk assessment
AI 理解论文
溯源树
样例
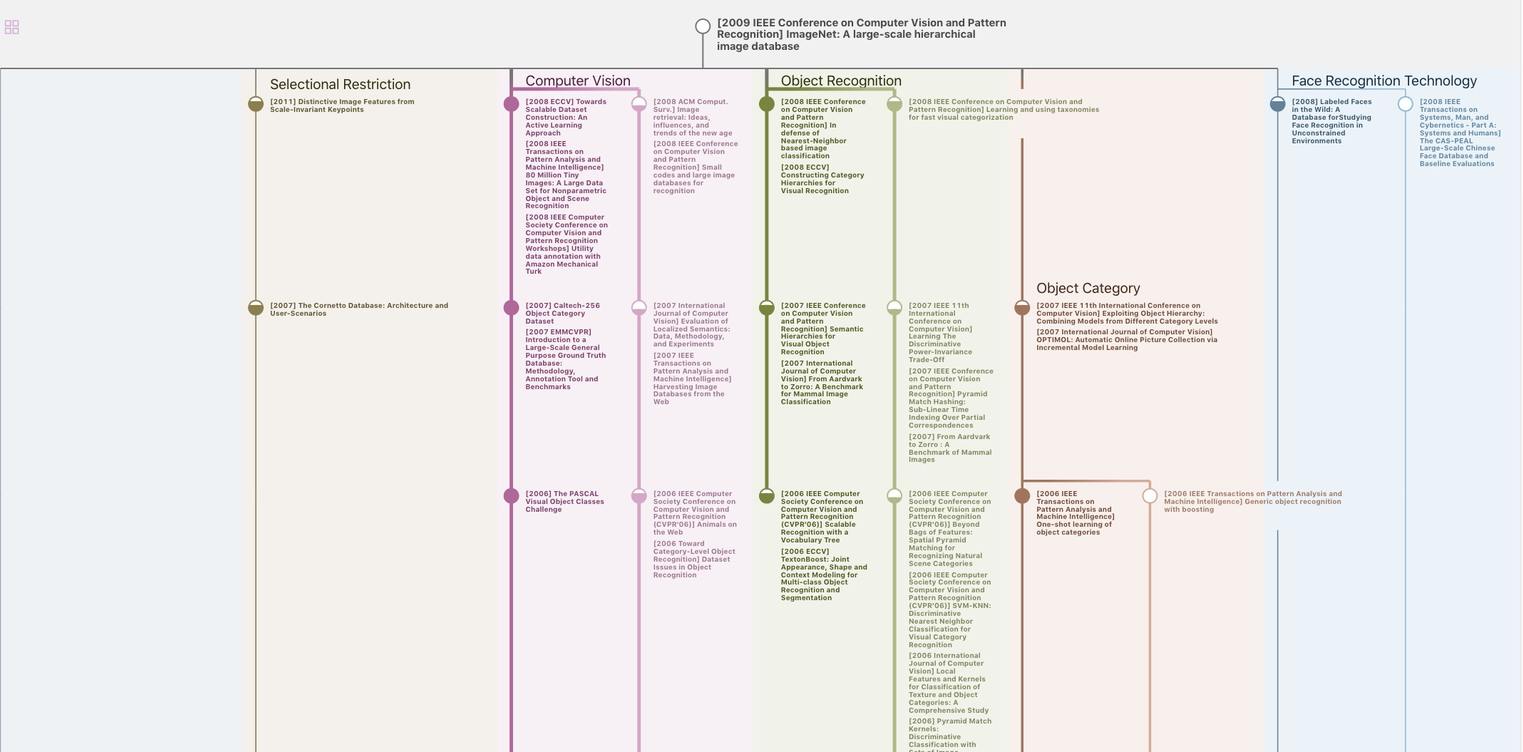
生成溯源树,研究论文发展脉络
Chat Paper
正在生成论文摘要