Degradation Trend Prediction of Hydropower Units Based on a Comprehensive Deterioration Index and LSTM
ENERGIES(2022)
摘要
Deterioration trend prediction of hydropower units helps to detect abnormal conditions of hydropower units and can prevent early failures. The reliability and accuracy of the prediction results are crucial to ensure the safe operation of the units and promote the stable operation of the power system. In this paper, the long short-term neural network (LSTM) is introduced, a comprehensive deterioration index (CDI) trend prediction model based on the time-frequency domain is proposed, and the prediction accuracy of the situation trend of hydropower units is improved. Firstly, the time-domain health model (THM) is constructed with back-propagation neural network (BPNN) and condition parameters of active power, guide vane opening and blade opening and the time-domain indicators. Subsequently, a frequency-domain health model (FHM) is established based on ensemble empirical mode decomposition (EEMD), approximate entropy (ApEn), and k-means clustering algorithm. Later, the time-domain degradation index (TDI) is developed according to THM, the frequency-domain degradation index (FDI) is constructed according to FHM, and the CDI is calculated as a weighted sum by TDI and FDI. Finally, the prediction model of LSTM is proposed based on the CDI to achieve degradation trend prediction. In order to validate the effectiveness of the CDI and the accuracy of the prediction model, the vibration waveform dataset of a hydropower plant in China is taken as a case study and compared with four different prediction models. The results demonstrate that the proposed model outperforms other comparison models in terms of predicting accuracy and stability.
更多查看译文
关键词
hydropower units, degradation trend prediction, comprehensive deterioration index, long and short-term neural network, ensemble empirical mode decomposition, approximate entropy
AI 理解论文
溯源树
样例
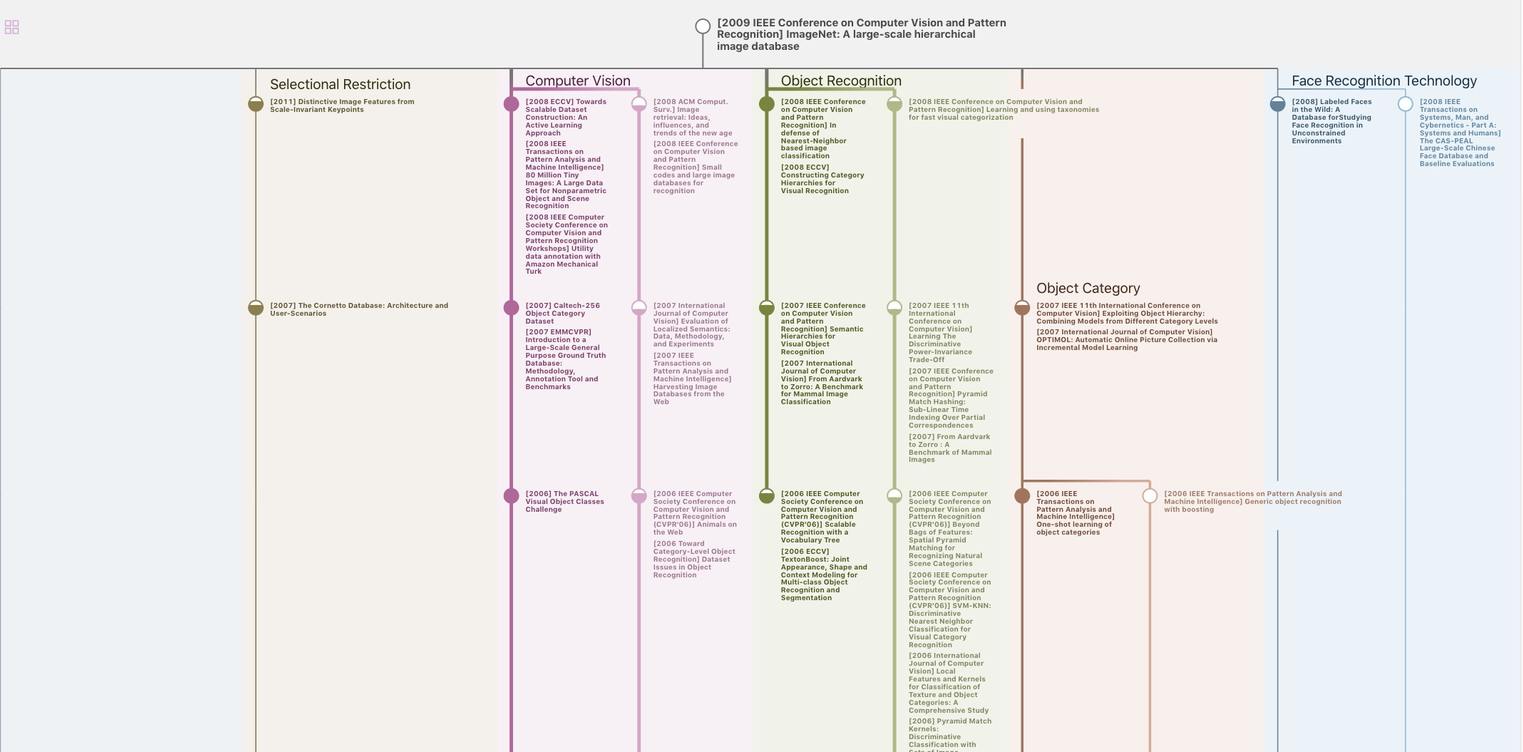
生成溯源树,研究论文发展脉络
Chat Paper
正在生成论文摘要