Low-Power Energy-Based Spike Detector ASIC for Implantable Multichannel BMIs
ELECTRONICS(2022)
摘要
Advances in microtechnology have enabled an exponential increase in the number of neurons that can be simultaneously recorded. To meet high-channel count and implantability demands, emerging applications require new methods for local real-time processing to reduce the data to transmit. Nonlinear energy operators are widely used to distinguish neural spikes from background noise featuring a good tradeoff between hardware resources and accuracy. However, they require an additional smoothing filter, which affects both area occupation and power dissipation. In this paper, we investigate a spike detector, based on a series of two nonlinear energy operators, and a simple and adaptive threshold, based on a three-point median operator. We show that our proposal provides good accuracy compared to other energy-based detectors on a synthetic dataset at different noise levels. Based on the proposed technique, a 1024-channel neural signal processor was designed in a 28 nm TSMC CMOS process by using latch-based static random-access memory (SRAM), demonstrating a total power consumption of 1.4 mu W/ch and a silicon area occupation of 230 mu m(2)/ch. These features, together with a comparison with the state of the art, demonstrate that our proposal constitutes an alternative for the development of next-generation multichannel neural interfaces.
更多查看译文
关键词
BMI, action potentials, noise estimate, memory, VLSI, low power
AI 理解论文
溯源树
样例
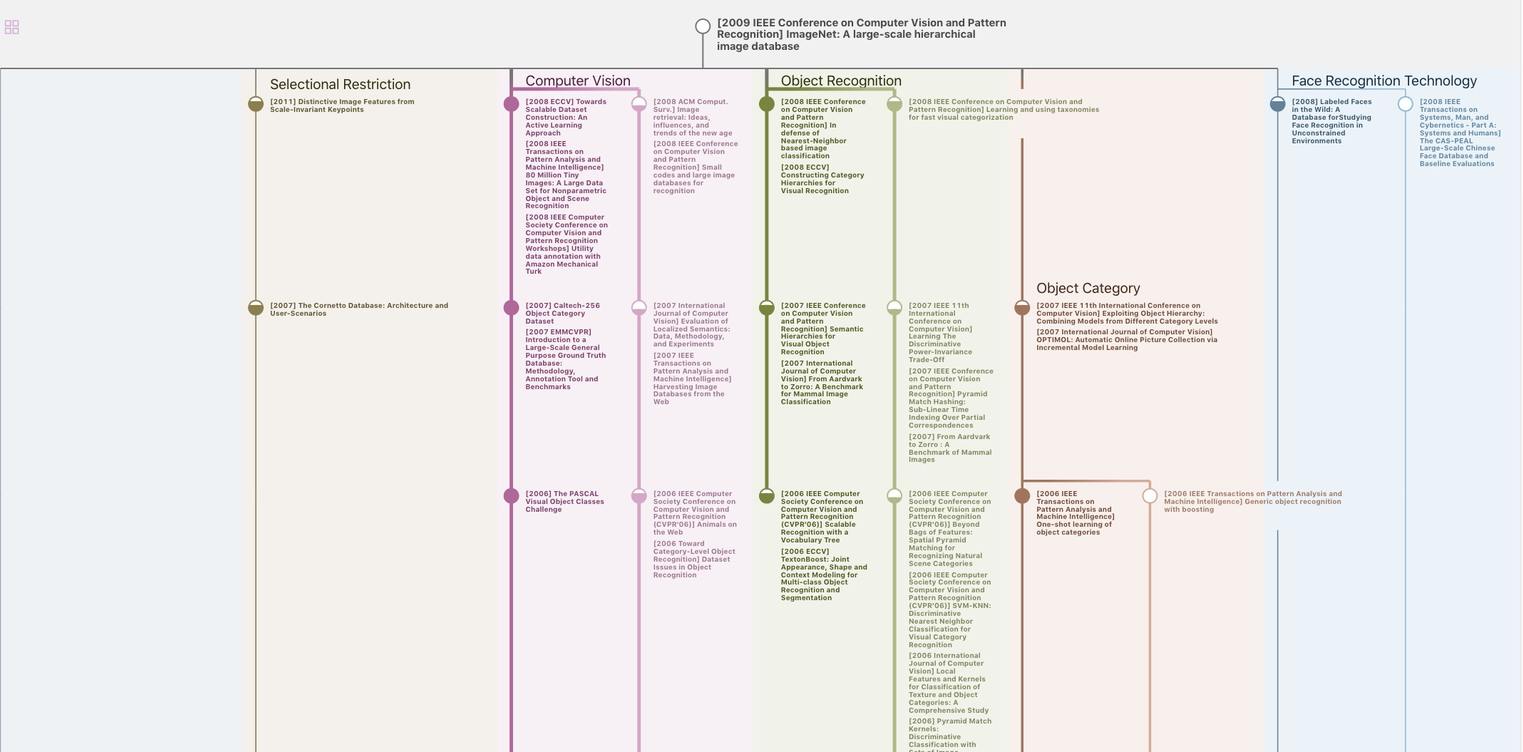
生成溯源树,研究论文发展脉络
Chat Paper
正在生成论文摘要