Soft Actor-Critic with Inhibitory Networks for Retraining UAV Controllers Faster
2022 INTERNATIONAL CONFERENCE ON UNMANNED AIRCRAFT SYSTEMS (ICUAS)(2022)
摘要
Active research in autonomous unmanned aerial vehicles (UAVs) seeks to combine the agility of Proportional-Integral-Derivative (PID) systems for low-level control with the adaptability of Deep Reinforcement Learning (DRL) to navigate through challenging, non-stationary environments. In the real world, there is often a need to quickly adapt trained DRL agents to more difficult tasks with conflicting rewards. For efficient retraining, the ability to leverage previously learned skills becomes critical. Unfortunately, using traditional DRL algorithms like soft actor critic (SAC) for retraining a policy can lead to catastrophic forgetting of the policy's known skills. In this work, inspired by neuroscience research, we propose a novel approach using SAC with inhibitory networks to allow separate and adaptive state value evaluations, as well as distinct automatic entropy tuning. We validate our method through experiments using a quadcopter in a realistic simulation environment and demonstrate the advantage of retraining. Moreover, we present the superiority of our approach compared to baseline methods with respect to both sample efficiency and cumulative success.
更多查看译文
关键词
deep reinforcement learning, soft actor-critic, transfer learning, UAV navigation, quadcopter simulation
AI 理解论文
溯源树
样例
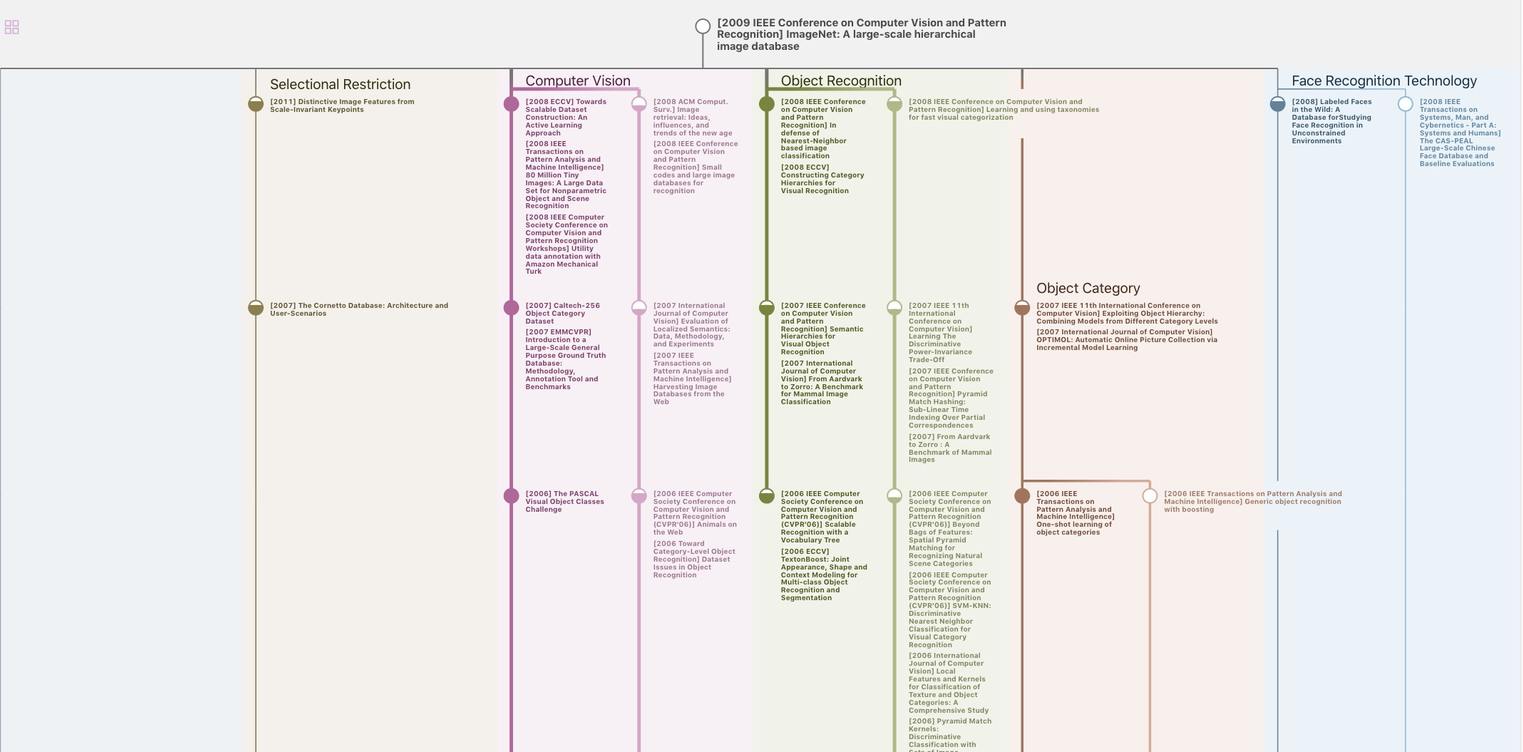
生成溯源树,研究论文发展脉络
Chat Paper
正在生成论文摘要