Accuracy Rate Maximization in Edge Federated Learning With Delay and Energy Constraints
IEEE SYSTEMS JOURNAL(2023)
摘要
Deploying deep learning models on edge servers can effectively alleviate the pressure on cloud data centers in computing, communication, and energy, etc. In this study, we attempt to extend this deployment scheme from edge servers to mobile terminals to further save spectrum resources, utilize communication resources, and provide intelligent applications. Unfortunately, the learning model experiences a slow convergence speed, and the mobile terminal consumes much energy if the model is only trained on the local devices with weak computing power. Therefore, we deploy deep learning algorithms (both in edge servers and mobile terminals) by considering the delay and energy consumption constraints of terminals in task processing. Specifically, a lightweight federated learning model is first proposed through the cooperative training between edge servers and mobile terminals. The trained model is then migrated to mobile terminals. Finally, image identification is considered to verify the effectiveness and efficiency of our proposed model. The experimental results show that the accuracy of the model reaches 90% using two datasets and three network models. In addition, it further reduces the processing delay and saves energy.
更多查看译文
关键词
Training, Mobile handsets, Servers, Delays, Computational modeling, Energy consumption, Collaborative work, Cooperative training, edge computing, federated learning, intelligent recognition, tensor-flow lite
AI 理解论文
溯源树
样例
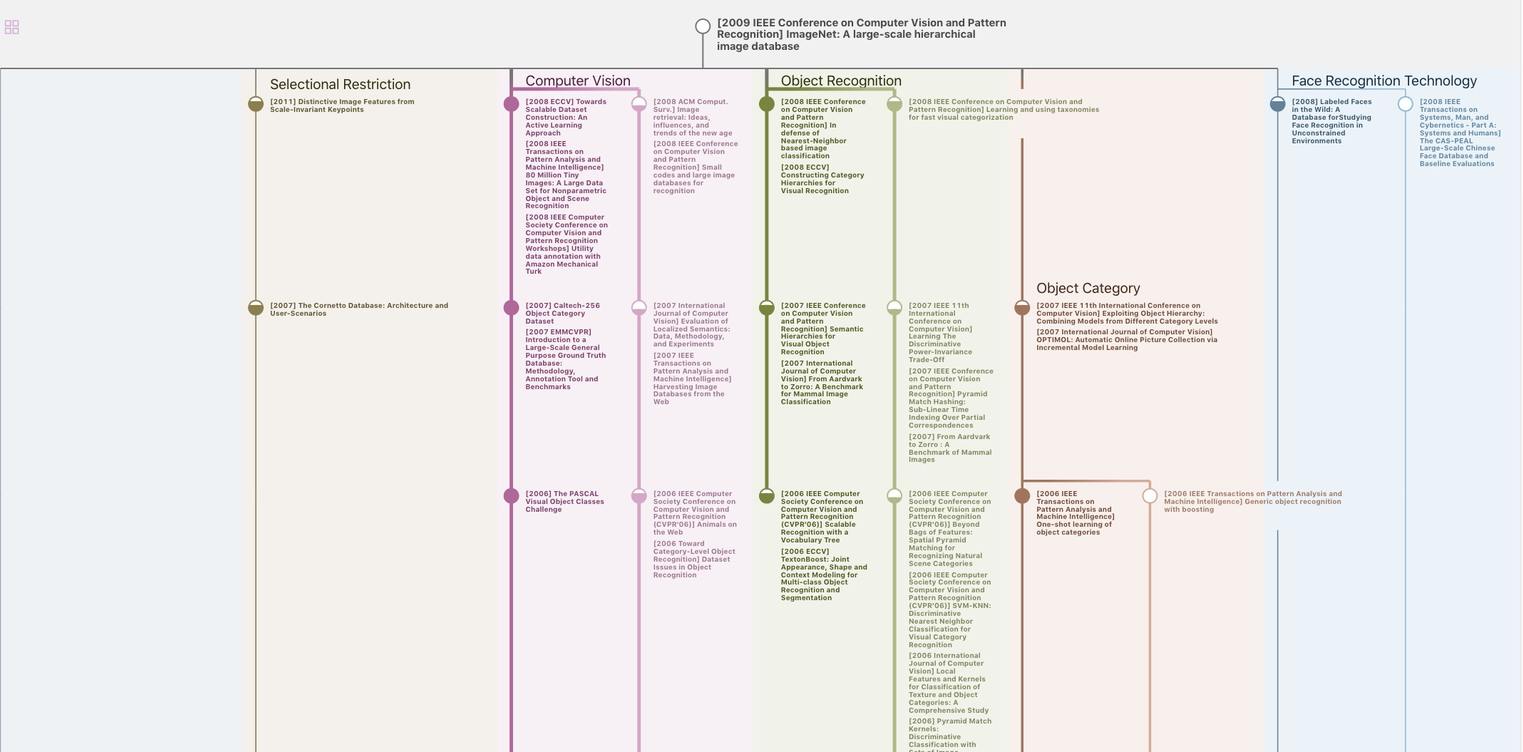
生成溯源树,研究论文发展脉络
Chat Paper
正在生成论文摘要