Neural Networks-Based Detection of Line Overflow Cyberattacks on AC State-Estimation of Smart Grids
IEEE SYSTEMS JOURNAL(2023)
摘要
A new category of false data injection (FDI) cyberattacks was recently proposed that can result in line overflow and cascading failures in electric grids by bypassing ac state-estimation. However, these attacks are bounded by multiple nonlinear constraints that provide different temporal features in the bad data that existing FDI detectors might fail to identify. In this article, a cyberattack model using stealthy FDIs on load or generation units is formulated that may result in multiple transmission line overflows without being detected by bad data detection (BDD) algorithms embedded in ac state-estimation. A detection framework based on neural networks is developed that uses bad data generated from the attack model for training purposes and detects the stealthy FDIs. Results confirm the detection of stealthy FDIs with accuracy and precision higher than 99% with 0-15% measurement noise levels. The developed detection framework outperforms existing BDD algorithms of ac state-estimators.
更多查看译文
关键词
Computer crime, Transmission line measurements, Power systems, Deep learning, Power measurement, Load modeling, Detectors, Deep neural networks, learning-based detection algorithm, stealthy false data injection (FDI) cyberattacks
AI 理解论文
溯源树
样例
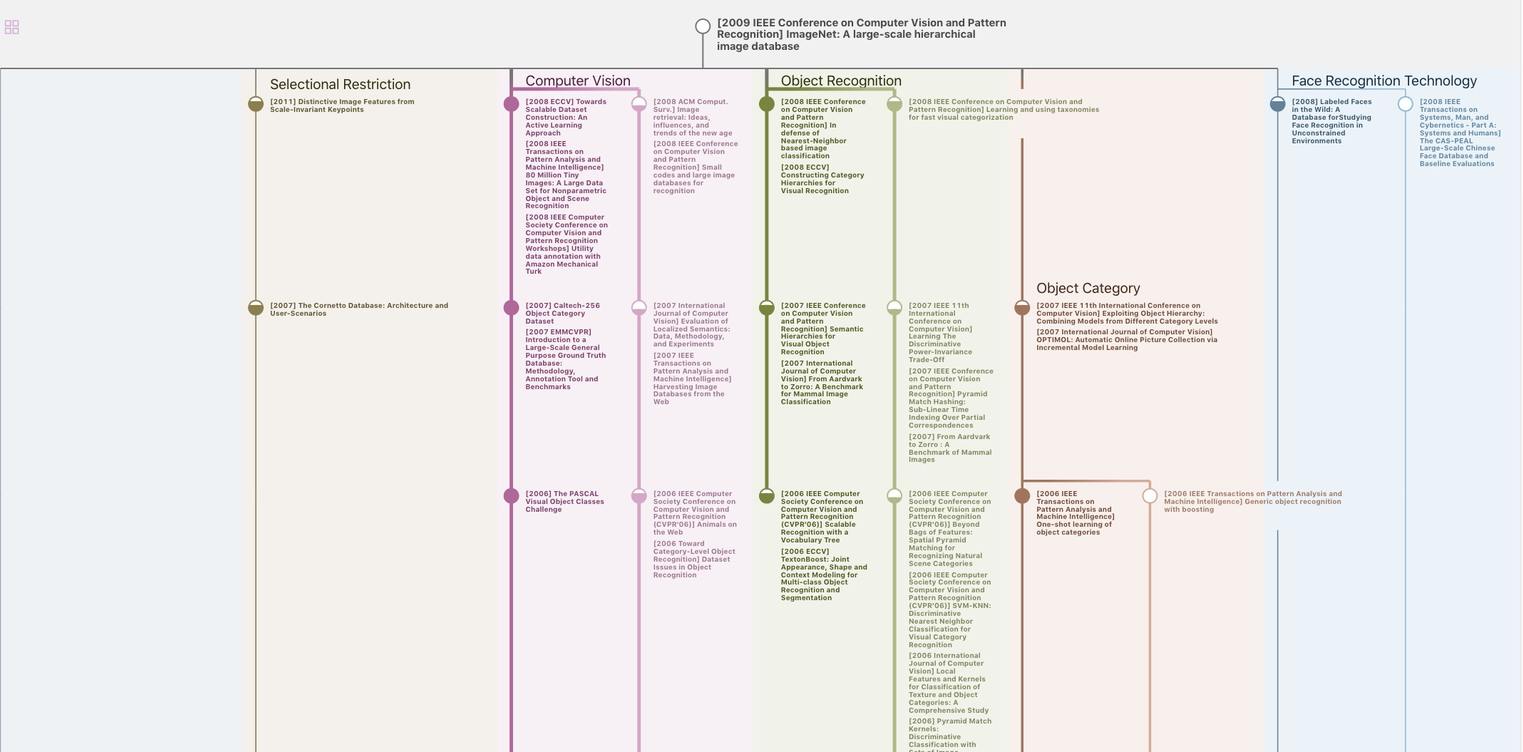
生成溯源树,研究论文发展脉络
Chat Paper
正在生成论文摘要