An Analysis of the Optimal Features for Sentinel-1 Oil Spill Datasets Based on an Improved J-M/K-Means Algorithm
REMOTE SENSING(2022)
摘要
With the rapid development of world shipping, oil spill accidents such as tanker collisions, illegal sewage discharges, and oil pipeline ruptures occur frequently. As the SAR system expands from single polarization to multipolarization, the Polarmetric Synthetic Aperture Radar (Pol-SAR) system has been widely used in marine oil spill detection. However, in the studies of the oil spill extraction in SAR images, there are some problems that limit large-scale oil spill detection work. As a transition from single-polarized to full-polarized, the dual-polarized system carries some polarization information and can be obtained in large quantities for free, which has become a major breakthrough in solving the problem of large-scale oil spill detection. In order to optimize the multisource features that can be extracted from dual-polarized SAR images, greatly improve the utilization rate of dual-polarized SAR oil spill images under the premise of reducing workload, and ensure the accuracy of marine oil spill extraction, this paper adopts the metric of inter-class separability, the Jeffries-Matusita distance, which improves on the traditional K-means algorithm by focusing on the noise sensitivity defect of the K-means algorithm; the artificial influence of J-M distance in measuring the separability between classes improves the algorithm in three aspects: sample selection, distance calculation, and data evaluation. Finally, using the inter-sample J-M distance of multisource features, the overall accuracy of image segmentation, the F1-score, and the results of correlation analysis between features, three advantageous features and three subdominant features are selected that can be used for marine oil spill detection.
更多查看译文
关键词
oil spills, feature selection, characteristic parameters, J-M distance, advantageous features
AI 理解论文
溯源树
样例
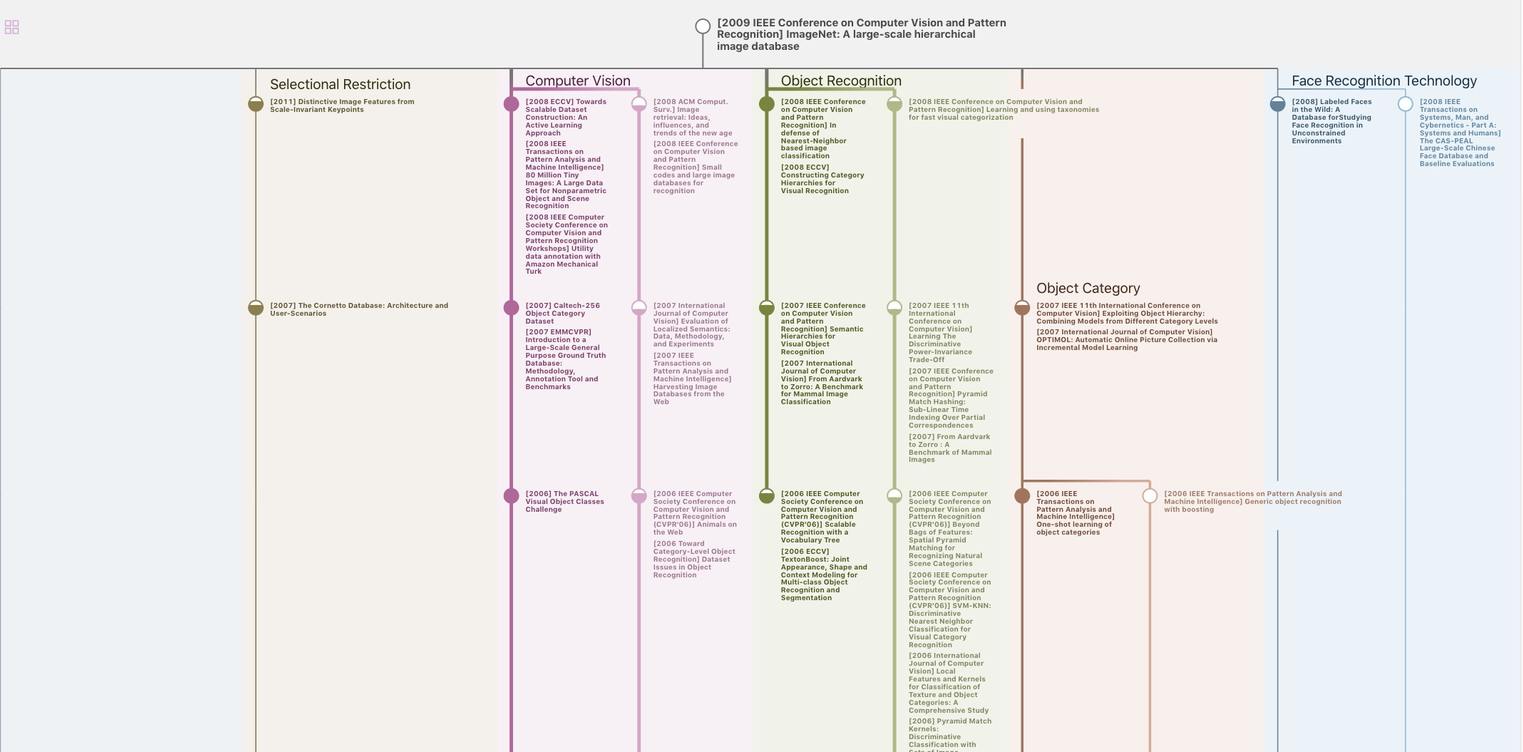
生成溯源树,研究论文发展脉络
Chat Paper
正在生成论文摘要