Live Fish Species Classification in Underwater Images by Using Convolutional Neural Networks Based on Incremental Learning with Knowledge Distillation Loss
MACHINE LEARNING AND KNOWLEDGE EXTRACTION(2022)
摘要
Nowadays, underwater video systems are largely used by marine ecologists to study the biodiversity in underwater environments. These systems are non-destructive, do not perturb the environment and generate a large amount of visual data usable at any time. However, automatic video analysis requires efficient techniques of image processing due to the poor quality of underwater images and the challenging underwater environment. In this paper, we address live reef fish species classification in an unconstrained underwater environment. We propose using a deep Convolutional Neural Network (CNN) and training this network by using a new strategy based on incremental learning. This training strategy consists of training the CNN progressively by focusing at first on learning the difficult species well and then gradually learning the new species incrementally using knowledge distillation loss while keeping the high performances of the old species already learned. The proposed approach yields an accuracy of 81.83% on the LifeClef 2015 Fish benchmark dataset.
更多查看译文
关键词
underwater image, fish recognition, deep learning, convolutional neural network, incremental learning, knowledge distillation loss
AI 理解论文
溯源树
样例
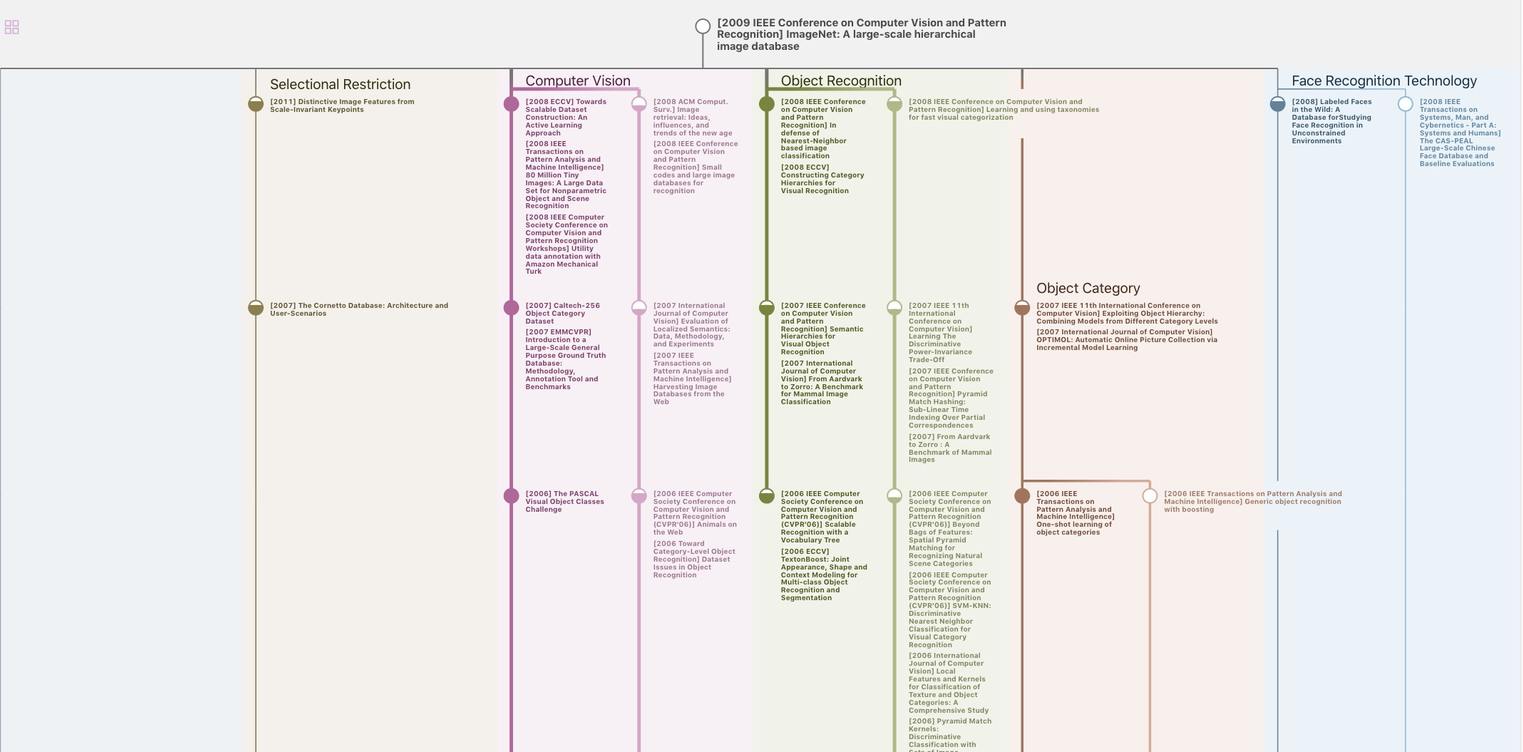
生成溯源树,研究论文发展脉络
Chat Paper
正在生成论文摘要