nantes.fr
PATTERN RECOGNITION(2022)
摘要
The prevalence of employing attention mechanisms has brought along concerns about the interpretability of attention distributions. Although it provides insights into how a model is operating, utilizing atten-tion as the explanation of model predictions is still highly dubious. The community is still seeking more interpretable strategies for better identifying local active regions that contribute the most to the final decision. To improve the interpretability of existing attention models, we propose a novel Bilinear Repre-sentative Non-Parametric Attention (BR-NPA) strategy that captures the task-relevant human-interpretable information. The target model is first distilled to have higher-resolution intermediate feature maps. From which, representative features are then grouped based on local pairwise feature similarity, to produce finer-grained, more precise attention maps highlighting task-relevant parts of the input. The obtained attention maps are ranked according to the activity level of the compound feature, which provides in-formation regarding the important level of the highlighted regions. The proposed model can be easily adapted in a wide variety of modern deep models, where classification is involved. Extensive quantitative and qualitative experiments showcase more comprehensive and accurate visual explanations compared to state-of-the-art attention models and visualization methods across multiple tasks including fine-grained image classification, few-shot classification, and person re-identification, without compromising the clas-sification accuracy. The proposed visualization model sheds imperative light on how neural networks 'pay their attention' differently in different tasks. (c) 2022 Elsevier Ltd. All rights reserved.
更多查看译文
关键词
Deep learning, Interpretability, Spatial attention, Resolution, Non-parametric
AI 理解论文
溯源树
样例
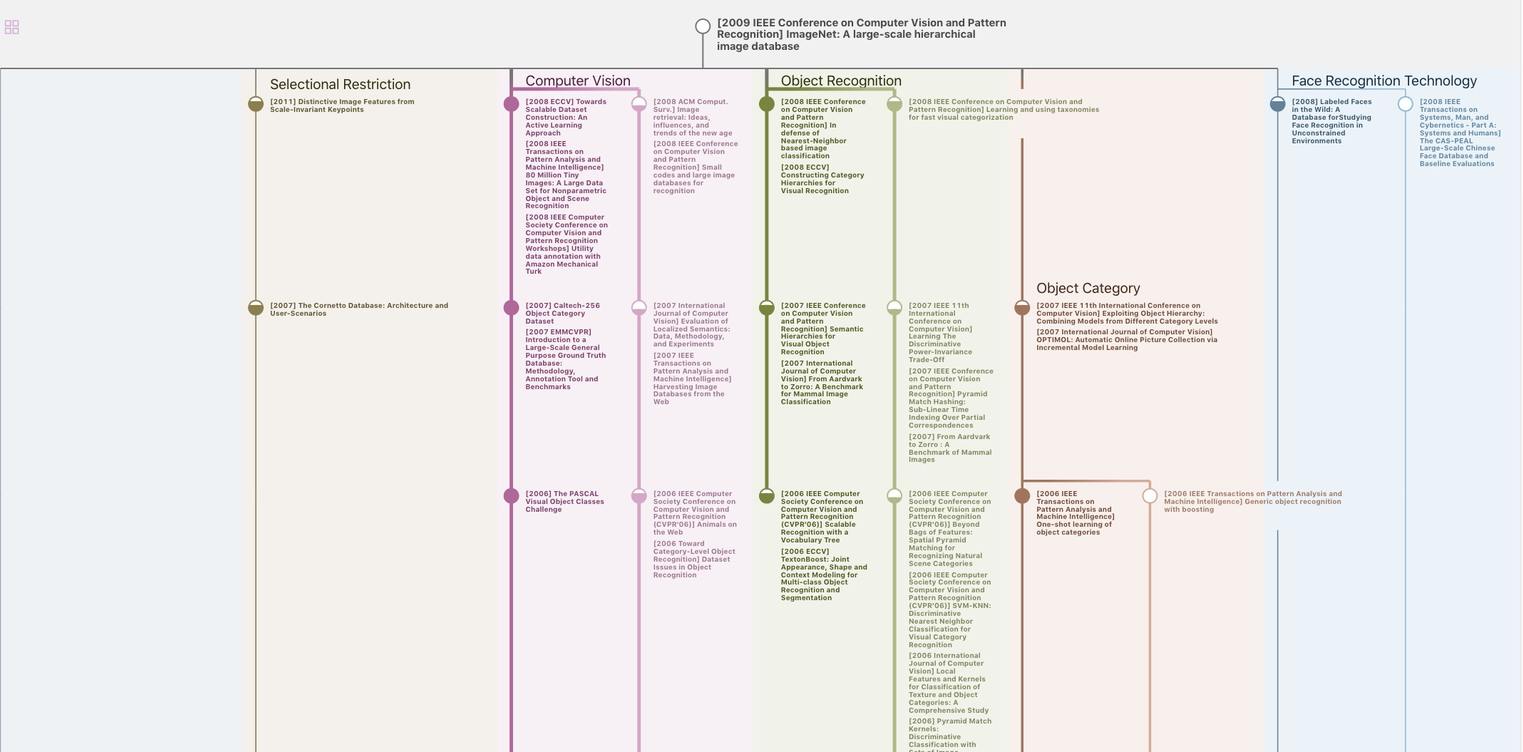
生成溯源树,研究论文发展脉络
Chat Paper
正在生成论文摘要