Short-term wind speed forecasting with regime-switching and mixture models at multiple weather stations over a large geographical area
JOURNAL OF RENEWABLE AND SUSTAINABLE ENERGY(2022)
摘要
This paper presents a methodology to incorporate large-scale atmospheric information into short-term wind speed forecast over a large geographical area of about 435 000 square kilometers in Alberta, Canada. The analysis was done using two publicly accessible datasets. The ERA5 reanalysis dataset is used for atmospheric clustering by applying the k-means algorithm and the hidden Markov model on atmospheric variables related to wind speeds. It is shown that atmospheric clustering results align with some known wind patterns in Alberta. For short-term wind forecast, we propose time series regime-switching models and mixture models that integrate the clustering results to predict 6-h ahead wind speed at 23 weather stations in Alberta, Canada. The predictive performance is compared for atmospheric clustering methods and forecasting models. The results show that models that take into account meteorological conditions perform better than those do not. Furthermore, modeling multiple locations simultaneously produces fewer forecasting errors than modeling at a single location. Published under an exclusive license by AIP Publishing.
更多查看译文
AI 理解论文
溯源树
样例
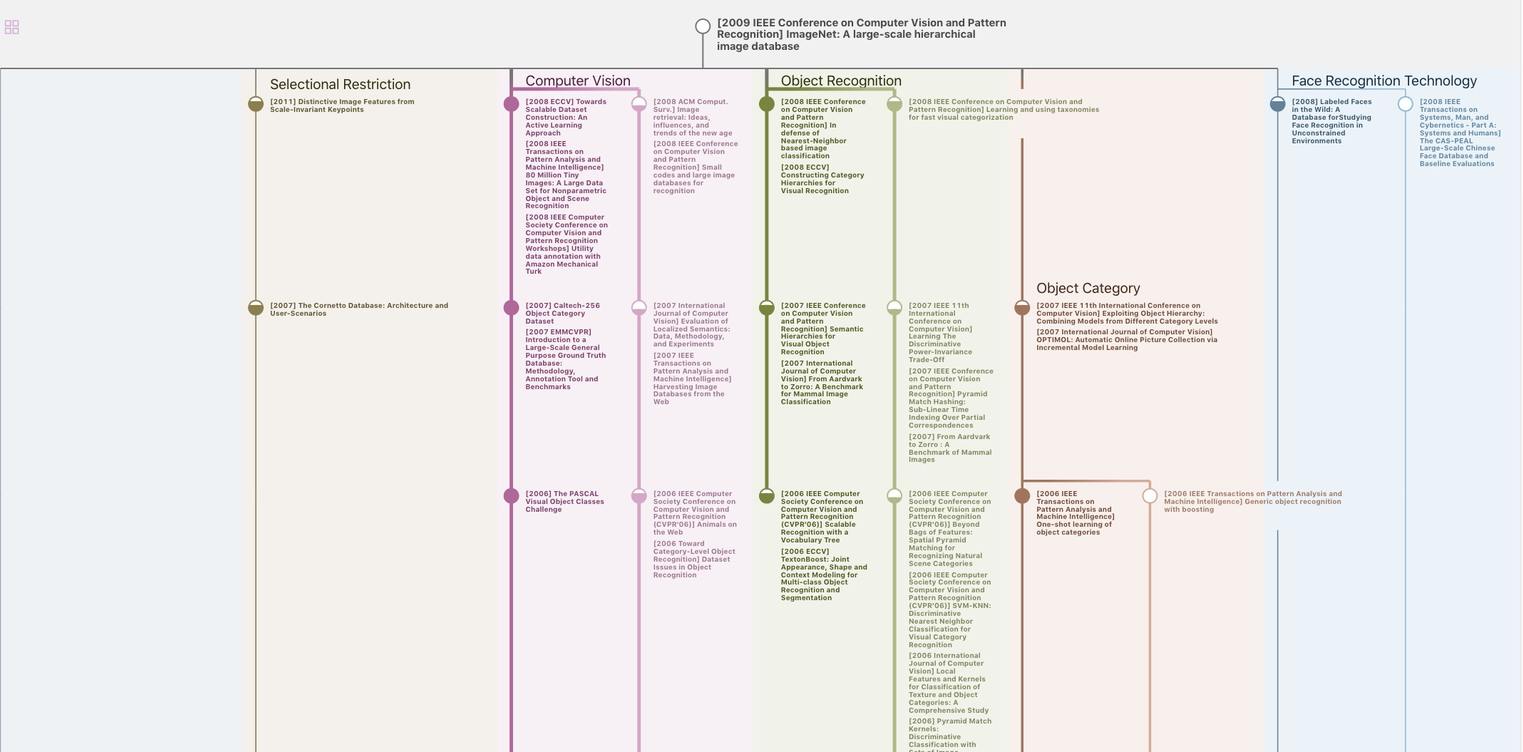
生成溯源树,研究论文发展脉络
Chat Paper
正在生成论文摘要