Wind turbine quantification and reduction of uncertainties based on a data-driven data assimilation approach
JOURNAL OF RENEWABLE AND SUSTAINABLE ENERGY(2022)
摘要
In this paper, we propose a procedure for quantifying and reducing uncertainties that impact numerical simulations involved in the estimation of the fatigue of a wind turbine structure. The present study generalizes a previous work carried out by the authors proposing to quantify and to reduce uncertainties that affect the properties of a wind turbine model by combining a global sensitivity analysis and a recursive Bayesian filtering approach. We extend the procedure to include the uncertainties involved in the modeling of a synthetic wind field. Unlike the model properties having a static or slow time-variant behavior, the parameters related to the external solicitation have a non-explicit dynamic behavior, which must be taken into account during the recursive inference. A non-parametric data-driven approach to approximate the non-explicit dynamic of the inflow related parameters is used. More precisely, we focus on data assimilation methods combining a nearest neighbor or an analog sampler with a stochastic filtering method such as the ensemble Kalman filter. The so-called data-driven data assimilation approach is used to recursively reduce the uncertainties that affect the parameters related to both model properties and wind field. For the approximation of the non-explicit dynamic of the wind inflow related parameters, in situ observations obtained from a light detection and ranging system and a cup-anemometer device are used. For the data-assimilation procedure, synthetic data simulated from the aero-servo-elastic numerical model are considered. The next investigations will be to verify the procedure with real in situ data.
更多查看译文
关键词
data-driven assimilation approach,turbine,uncertainties,wind
AI 理解论文
溯源树
样例
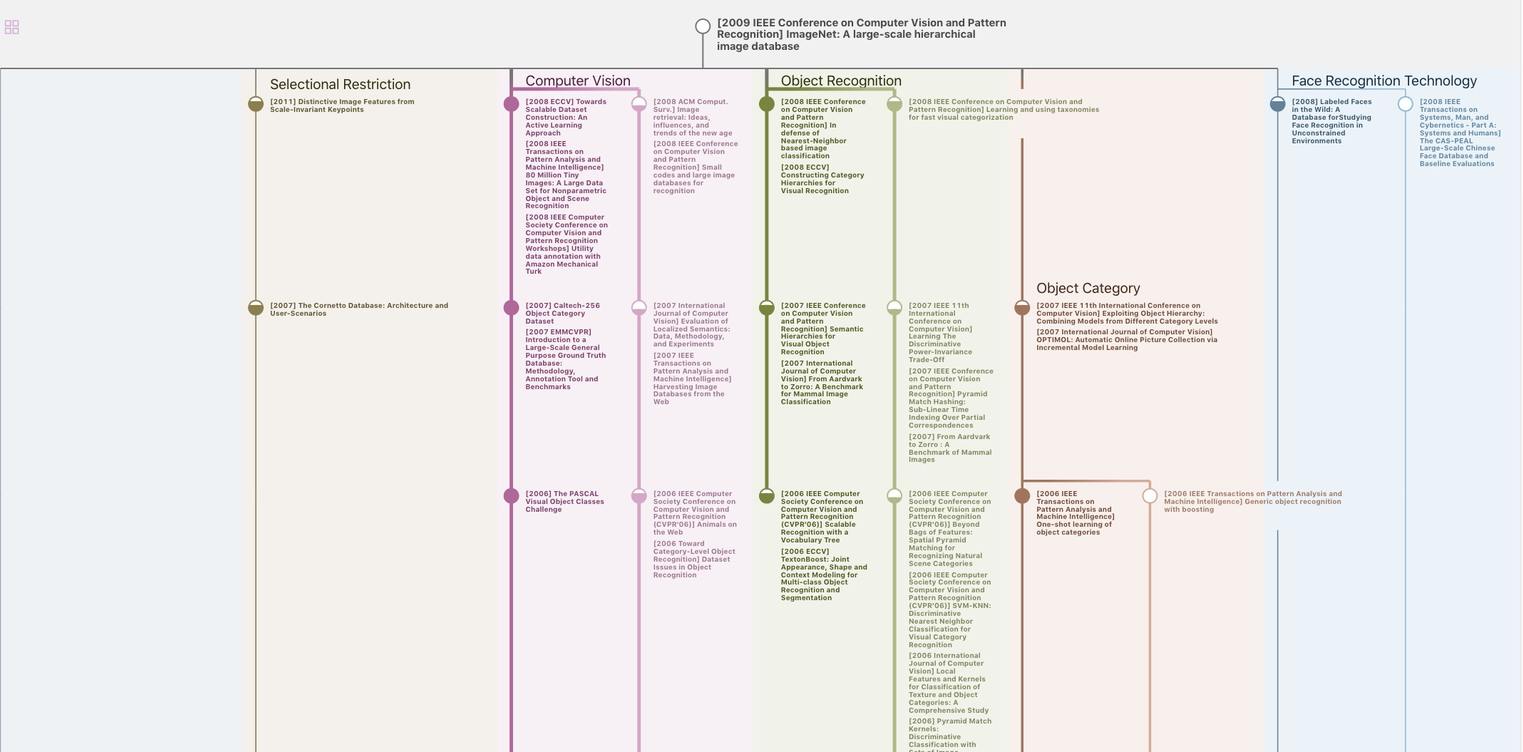
生成溯源树,研究论文发展脉络
Chat Paper
正在生成论文摘要