Estimation of Potential Evapotranspiration in the Yellow River Basin Using Machine Learning Models
ATMOSPHERE(2022)
摘要
Potential evapotranspiration (PET) is an important input variable of many ecohydrological models, but commonly used empirical models usually input numerous meteorological factors. In consideration of machine learning for complex nonlinear learning, we evaluated the applicability of three machine learning algorithms in PET estimation in the Yellow River basin (YRB), in addition to determining significant factors affecting the accuracy of machine learning. Furthermore, the importance of meteorological factors at varying altitudes and drought index grades for PET simulation were evaluated. The results show that the accuracy of PET simulation in the YRB depends on the input of various meteorological factors; however, machine learning models including average temperature (T-mean) and sunshine hours (n) as input achieved satisfactory accuracy in the absence of complete meteorological data. Random forest generally performed best among all investigated models, followed by extreme learning machine, whereas empirical models overestimated or underestimated PET. The importance index shows that T-mean is the most influential factor with respect to PET, followed by n, and the influence of T-mean on PET gradually decreased with increased altitude and drier climate, whereas the influence of n shows the opposite trend.
更多查看译文
关键词
potential evapotranspiration, machine learning, empirical model, importance index, Yellow River basin
AI 理解论文
溯源树
样例
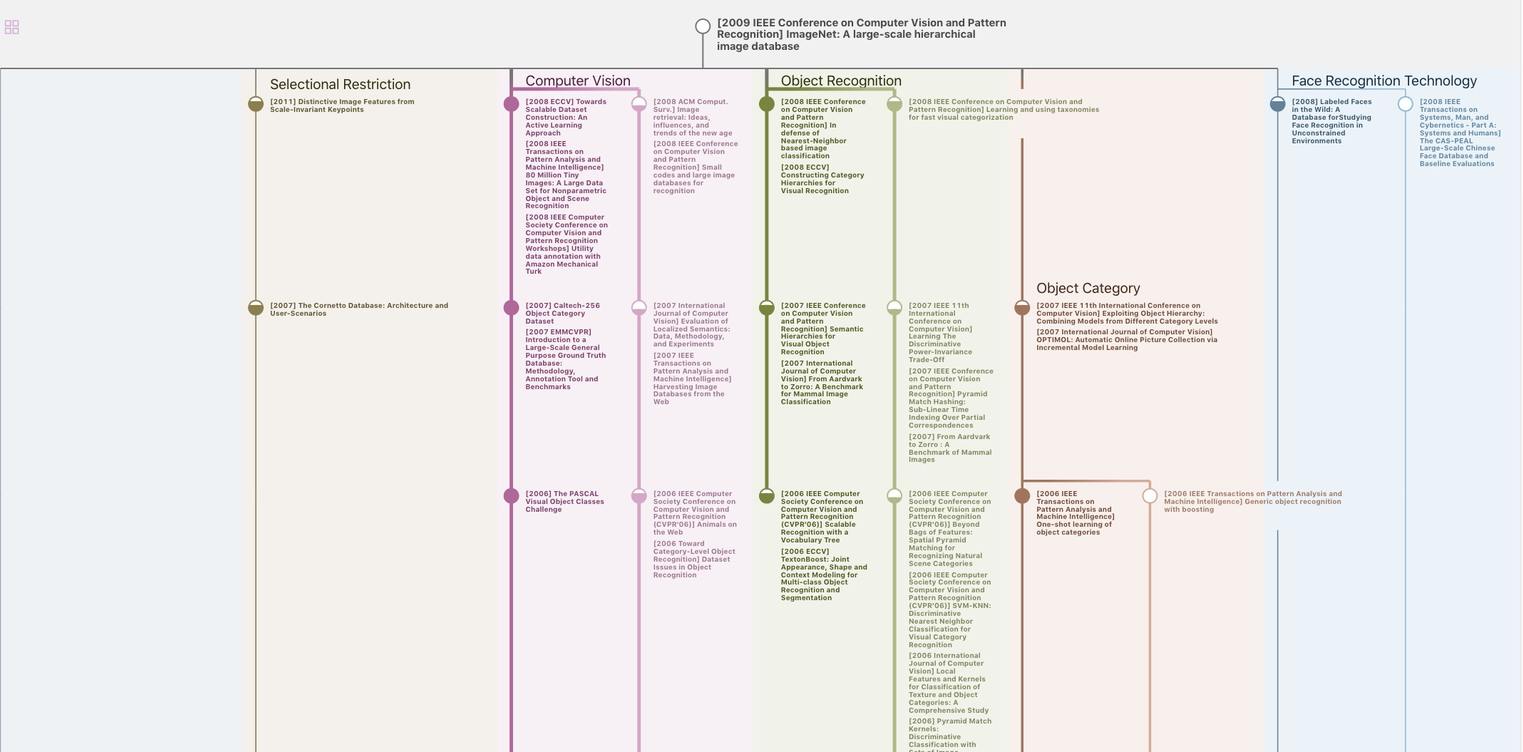
生成溯源树,研究论文发展脉络
Chat Paper
正在生成论文摘要