PRISMS-Plasticity TM: An Open-Source Rapid Texture Evolution Analysis Pipeline
INTEGRATING MATERIALS AND MANUFACTURING INNOVATION(2022)
摘要
The evolution of texture during different mechanical processes is an integral part of Integrated Computational Materials Engineering (ICME) of metals and alloys. In the present work, PRISMS-Plasticity TM, an open-source rapid texture evolution analysis pipeline, based on the Taylor model is integrated into the open-source crystal plasticity software, PRISMS-Plasticity. However, unlike PRISMS-Plasticity, the pipeline does not involve any finite element solver. The Taylor-type crystal plasticity model is used to capture the evolution of texture. The pipeline aims to significantly minimize the cost of texture evolution simulations, so one can use the framework along with data-driven ICME design methods and generate high-throughput data with low costs for general analysis or machine learning applications. All the rate-independent and rate-dependent crystal plasticity models available in the Materials library of PRISMS-Plasticity can be used in the pipeline. The models can capture extension twinning as an important deformation mechanism in alloys with hexagonal close-packed crystal structures. The PRISMS-Plasticity TM pipeline is efficiently integrated with experimental characterization techniques such as electron backscatter diffraction (EBSD), and these pipelines use other open-source software packages such as DREAM.3D where appropriate. In addition, the PRISMS-Plasticity TM results are integrated with the PRISMS Center Materials Commons information repository. In this investigation, three different examples of texture evolution in a polycrystalline OFHC copper, extruded Mg alloy ZK60A, and an aluminum alloy 7075-T6 are presented to show the capability of PRISMS-Plasticity TM. The pipeline can take advantage of the parallel performance of PRISMS-Plasticity and scales exceptionally well for large problems running on multiple processors. The computational efficiency of this tool can be used to build large databases for use in machine learning applications. Such a large dataset is established containing process–microstructure relationships of 7075-T6 Al. The extracted data is visualized in 2-D latent space using an unsupervised machine learning model called the variational autoencoder (VAE). The trained VAE model is used to identify economical process sequences which lead to desired properties.
更多查看译文
关键词
Texture,Crystal plasticity,PRISMS-Plasticity,ICME,Twinning,Machine learning
AI 理解论文
溯源树
样例
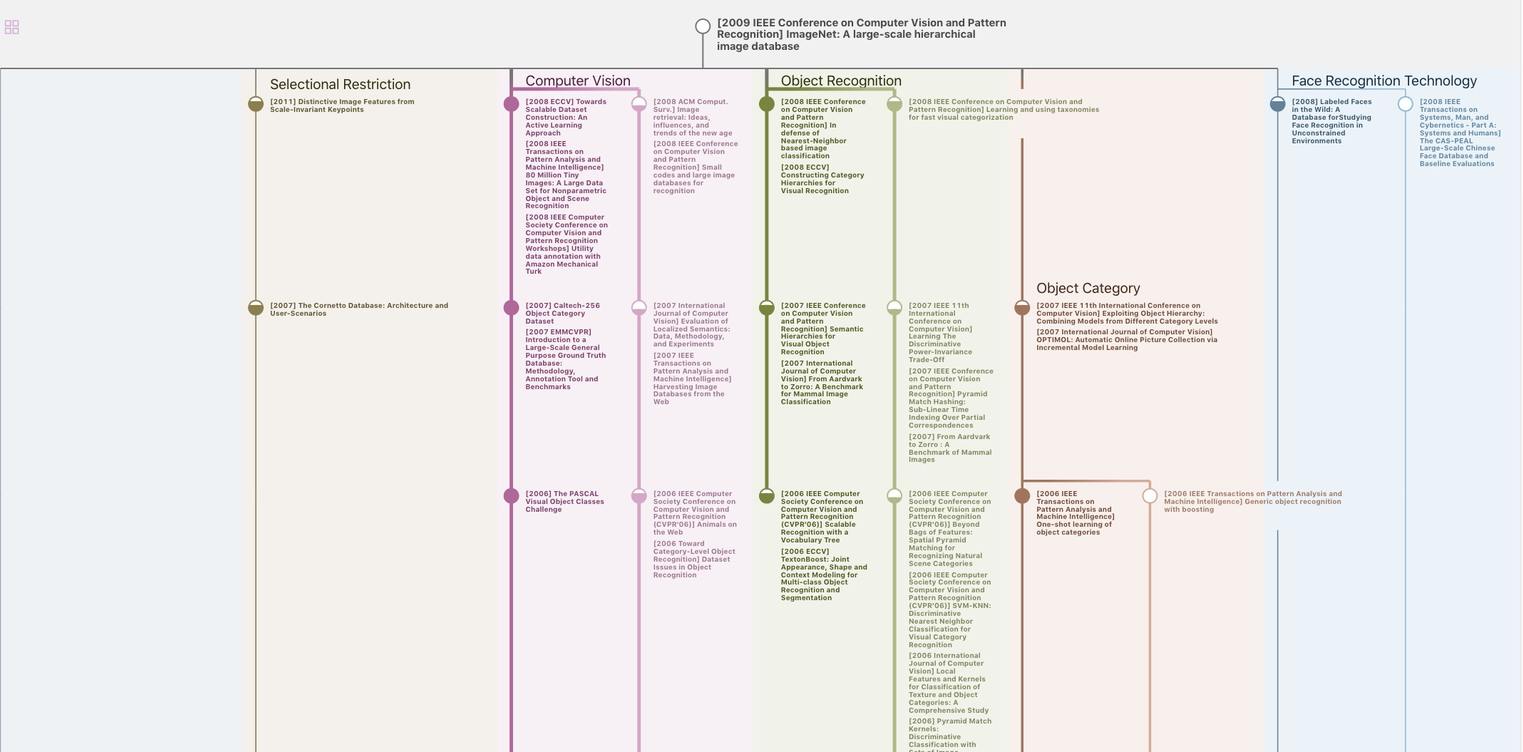
生成溯源树,研究论文发展脉络
Chat Paper
正在生成论文摘要