Comparative Study for Patch-Level and Pixel-Level Segmentation of Deep Learning Methods on Transparent Images of Environmental Microorganisms: From Convolutional Neural Networks to Visual Transformers
APPLIED SCIENCES-BASEL(2022)
摘要
Currently, the field of transparent image analysis has gradually become a hot topic. However, traditional analysis methods are accompanied by large amounts of carbon emissions, and consumes significant manpower and material resources. The continuous development of computer vision enables the use of computers to analyze images. However, the low contrast between the foreground and background of transparent images makes their segmentation difficult for computers. To address this problem, we first analyzed them with pixel patches, and then classified the patches as foreground and background. Finally, the segmentation of the transparent images was completed through the reconstruction of pixel patches. To understand the performance of different deep learning networks in transparent image segmentation, we conducted a series of comparative experiments using patch-level and pixel-level methods. In two sets of experiments, we compared the segmentation performance of four convolutional neural network (CNN) models and a visual transformer (ViT) model on the transparent environmental microorganism dataset fifth version. The results demonstrated that U-Net++ had the highest accuracy rate of 95.32% in the pixel-level segmentation experiment followed by ViT with an accuracy rate of 95.31%. However, ResNet50 had the highest accuracy rate of 90.00% and ViT had the lowest accuracy of 89.25% in the patch-level segmentation experiments. Hence, we concluded that ViT performed the lowest in patch-level segmentation experiments, but outperformed most CNNs in pixel-level segmentation. Further, we combined patch-level and pixel-level segmentation results to reduce the loss of segmentation details in the EM images. This conclusion was also verified by the environmental microorganism dataset sixth version dataset (EMDS-6).
更多查看译文
关键词
patch-level, pixel-level, image classification, image segmentation, transparent images, deep learning, convolutional neural network, visual transformer, environmental microorganism
AI 理解论文
溯源树
样例
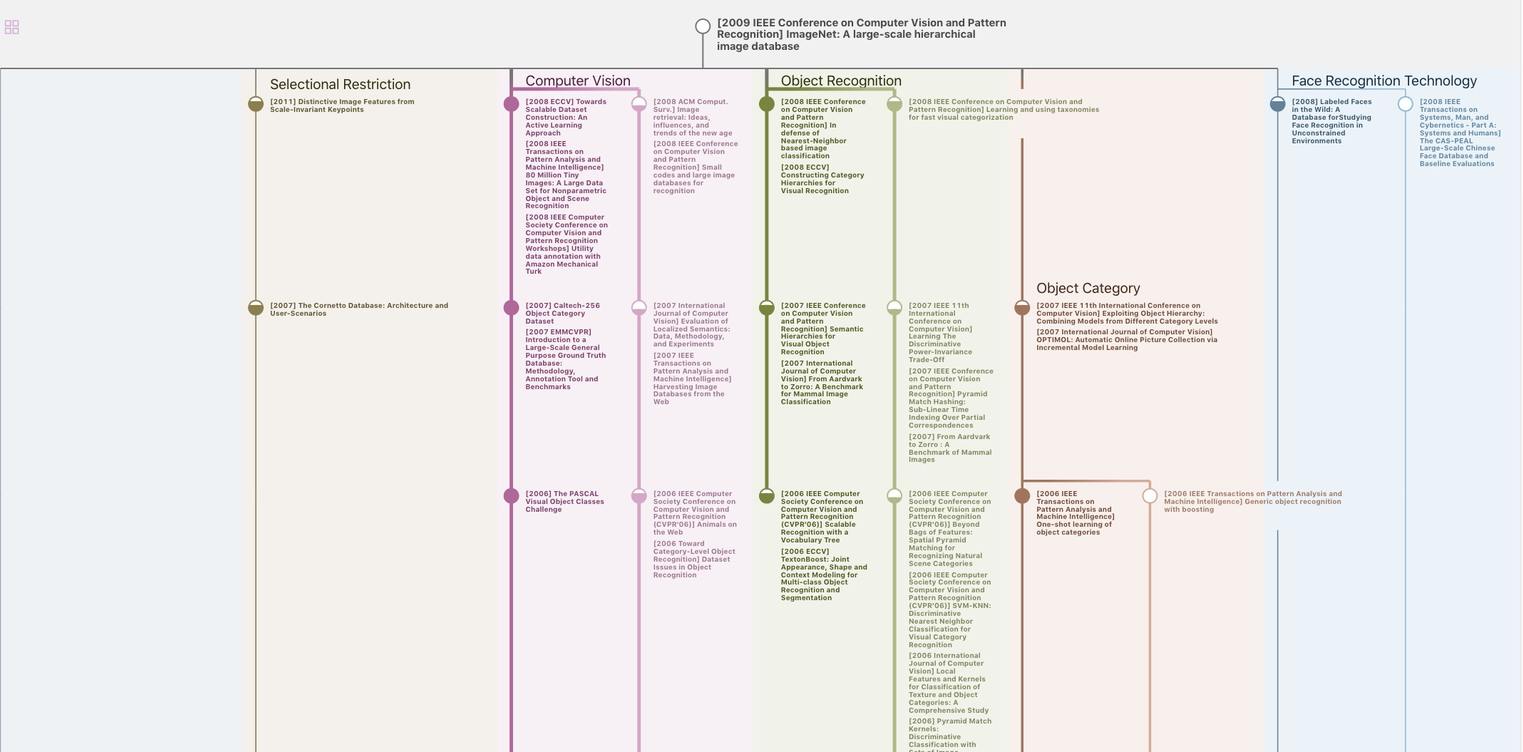
生成溯源树,研究论文发展脉络
Chat Paper
正在生成论文摘要