Pre-Event Two-Stage Proactive Control for Enhanced Distribution System Resiliency
IEEE ACCESS(2022)
摘要
Recent advancements in prediction technologies have motivated the power distribution utilities to actively utilize forecasts to minimize the impact of high-impact low frequency (HILF) events. Accurate forecasting coupled with past operational experiences potentially enables pre-event control, aiming to reduce the impact on the system in a network with finitely large numbers of automatic and manually operated control devices. Impacts to the critical infrastructure facilities, also known as critical loads (CLs), are minimized when a section of the network is appropriately deenergized before the event strikes. However, predictions are not perfect, and given limited accuracy with ever-changing weather forecasts, control actions are required to be constantly updated until the event has passed. Once the events are predicted with high enough confidence and the necessary reconfiguration strategy has been identified, the operator must configure the manually operable switches significantly ahead of event landfall to ensure the operational crews' safe return from potentially hazardous zones. Given limited resource availability and requisite manual switching operations, some of the loads would also have to be deenergized. Overall resiliency of the system will be improved if the loads remain connected through remotely operable switches until minutes before the event makes landfall. The proposed two-stage control framework with a necessary mathematical formulation facilitates the same. Furthermore, the proposed framework enables continuous corrective action based on available lead time. The developed proactive control framework is demonstrated using a modified IEEE 123-Node system model and a real-world isolated mu-grid based on the Cordova Electric Cooperative system with superior performance results.
更多查看译文
关键词
Critical loads, distribution system resiliency, extreme events, optimization, proactive restoration
AI 理解论文
溯源树
样例
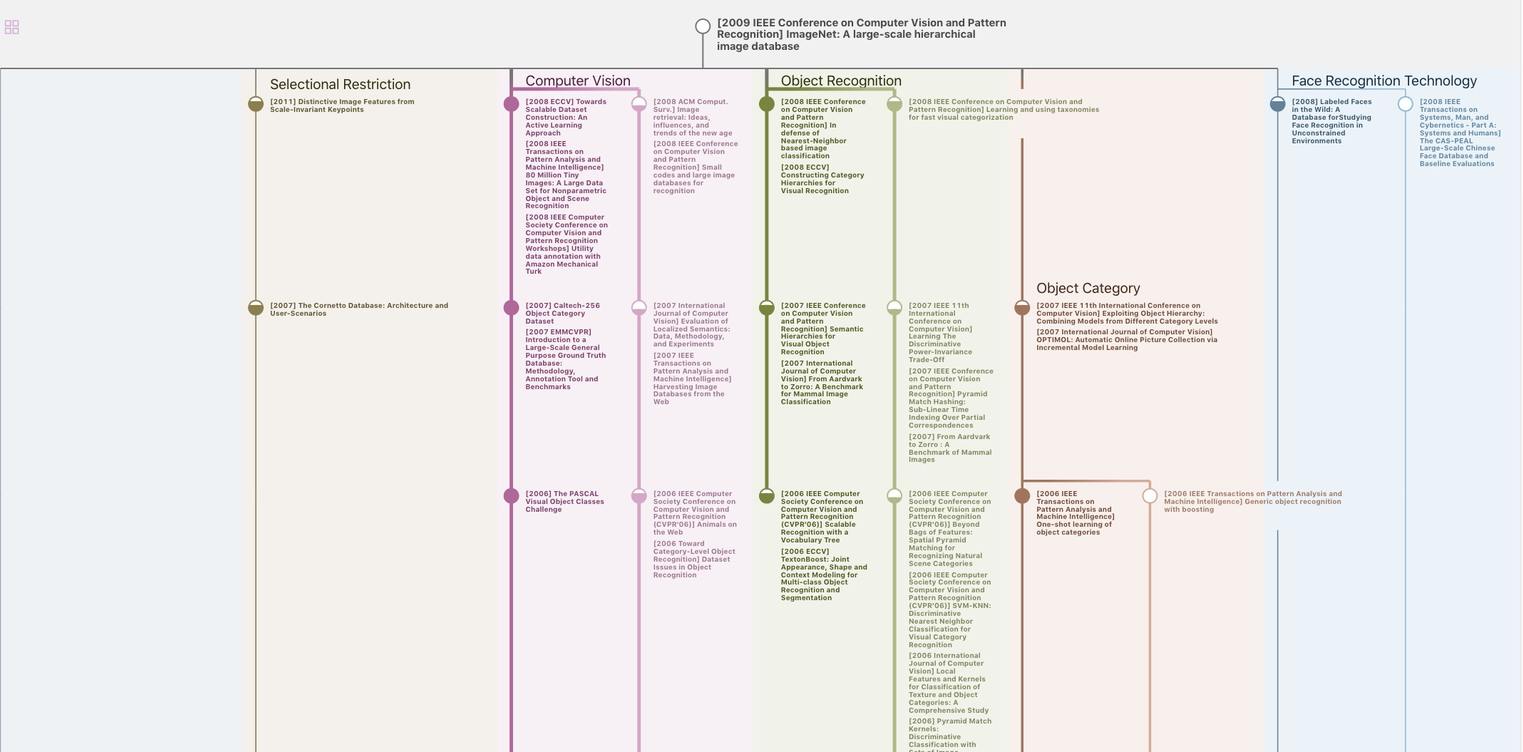
生成溯源树,研究论文发展脉络
Chat Paper
正在生成论文摘要