End-to-end forecasting of needle trajectory in percutaneous ablation
MEDICAL IMAGING 2021: IMAGE-GUIDED PROCEDURES, ROBOTIC INTERVENTIONS, AND MODELING(2021)
摘要
Percutaneous techniques are becoming more widely adopted for treating solid organ tumours. With many of these techniques using image guidance, accuracy is essential to reduce the risk of complications and tumour recurrence. We propose a novel approach to needle trajectory forecasting using a 3D U-Net trained on interventional computed tomography (iCT) images from renal cryoablation procedures. The U-Net is trained to predict future needle locations from present iCT images, supervised by ground truth labels at future time points. Furthermore, we demonstrate that forecasting needle trajectory may be substantially improved by Monte Carlo dropout (MCDO), in addition to generating uncertainty maps of the predictions. With 122 training iCT volumes, a Dice coefficient of 0.48 on highly elongated needle morphology was achieved based on 41 unseen test cases, significantly outperforming two models not using MCDO at inference with Dice scores of 0.14 and 0.32. MCDO also greatly improved the predicted needle morphology and was able to incorporate directional information into the predictions. Our approach shows promise for improving accuracy and workflow in image-guided procedures, with an interesting research direction to predict uncertain future interventional events by ensemble in supervised approaches.
更多查看译文
关键词
Forecasting,needle,convolutional neural network,uncertainty,interventional radiology,computed tomography
AI 理解论文
溯源树
样例
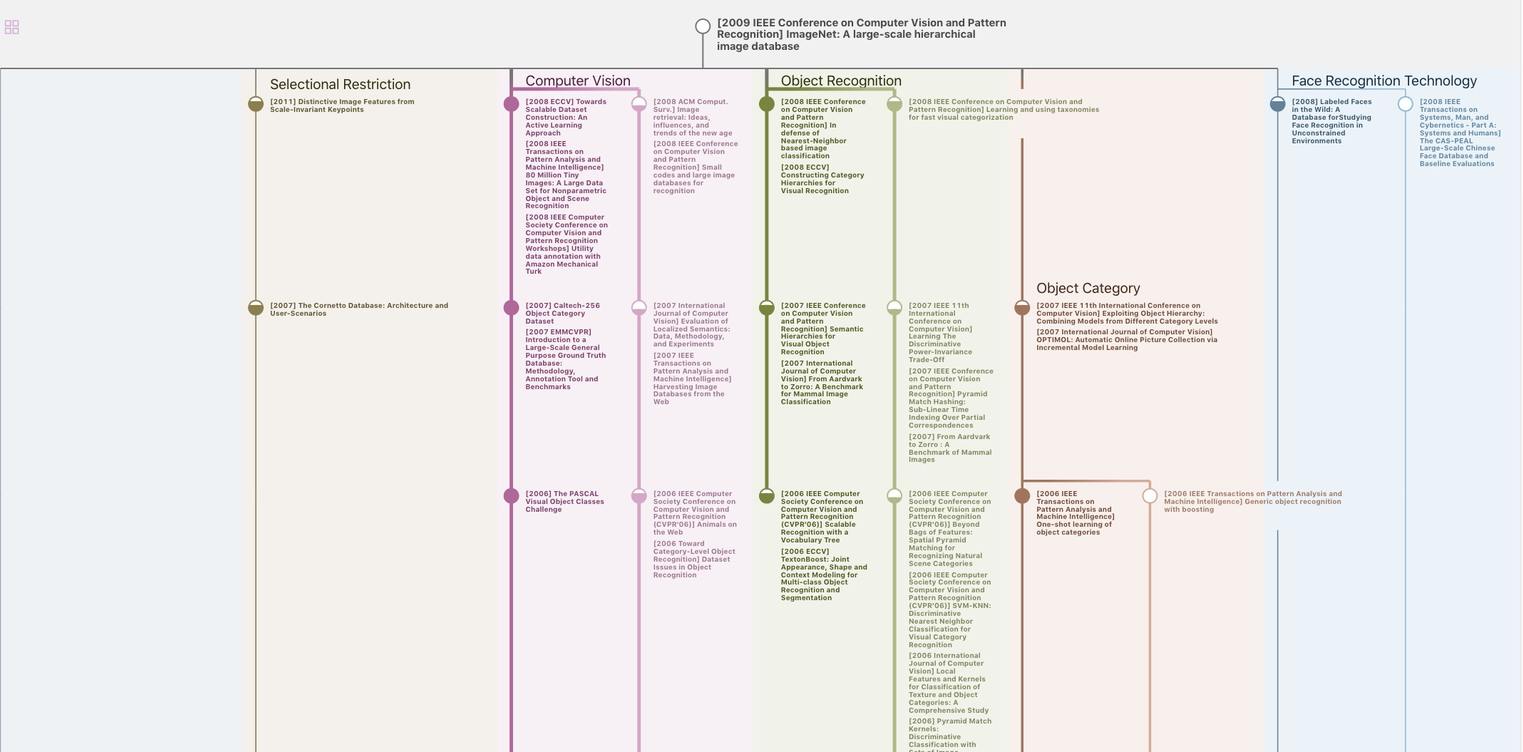
生成溯源树,研究论文发展脉络
Chat Paper
正在生成论文摘要