Network Traffic Overload Prediction with Temporal Graph Attention Convolutional Networks
2022 IEEE INTERNATIONAL CONFERENCE ON COMMUNICATIONS WORKSHOPS (ICC WORKSHOPS)(2022)
摘要
With the rapid explosion of network traffic volume, the prediction of traffic overload in the cellular network, which is defined as whether the traffic of a base station exceeds a predefined threshold, has become a crucial research problem regarding energy utilization and resource allocation. Most existing methods primarily model the dynamic patterns of traffic time series and compare the results with the predefined thresholds to predict traffic overload, taking into account a large amount of small-scale redundant data. To focus on the changes near the thresholds, i.e., the traffic burst circumstances, this paper adopts the softattention mechanism to fuse the threshold-based discrete and continuous time series characteristics to predict traffic overload. In addition, to capture the spatial correlations of the most related neighbors, we employ a Dual-KNN mechanism to select neighboring base stations and leverage the Graph Attention Network (GAT) to capture the spatial dependencies. Furthermore, we deploy a gated Temporal Convolutional Network (TCN) to model the temporal dependencies of the network traffic. Extensive experiments demonstrate that our proposed method effectively forecasts the traffic overload and outperforms the state-of-the-art algorithms by 4.07%.
更多查看译文
关键词
traffic overload prediction, graph attention networks, temporal convolutional networks
AI 理解论文
溯源树
样例
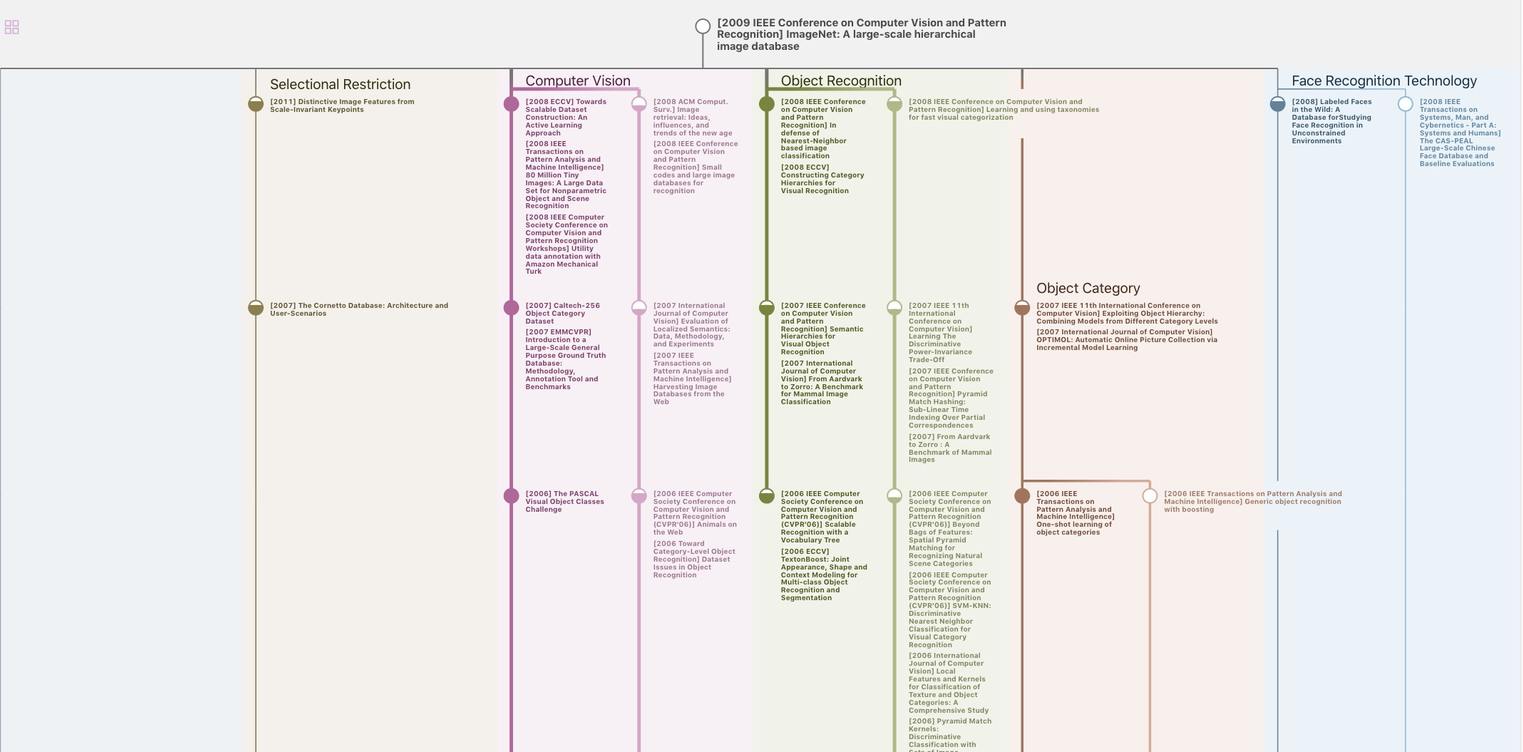
生成溯源树,研究论文发展脉络
Chat Paper
正在生成论文摘要