Multi-Agent Low-Bias Reinforcement Learning for Resource Allocation in UAV-Assisted Networks
2022 IEEE International Conference on Communications Workshops (ICC Workshops)(2022)
摘要
This paper considers an unmanned aerial vehicle (UAV)-assisted downlink transmission. To maximize the aver-age achievable channel capacity among the ground users, a multi-agent low-bias deep reinforcement learning (MA-LB-DRL) scheme is proposed to solve the joint optimization problem of trajectory design, channel selection and power control. Firstly, considering the NP-hard, non-convex, and mixed-integer features, the joint optimization problem is decoupled into two sub-problems, which deal with the integer-action and continuous-action problem respectively. Secondly, an MA-LB-DRL scheme, which consists of a multi-agent double deep Q-network (MAD-DQN) for channel selection and a multi-agent twin delayed deep deterministic policy gradient (MATD3PG) for trajectory design and power control, is proposed to overcome the overestimation bias via reducing value function approximation error at the expense of tiny complexity. Finally, the simulation results demon-strate that the proposed scheme achieves high channel capacity than the benchmark schemes.
更多查看译文
关键词
unmanned aerial vehicle (UAV),deep reinforcement learning,channel selection,power control,trajectory design
AI 理解论文
溯源树
样例
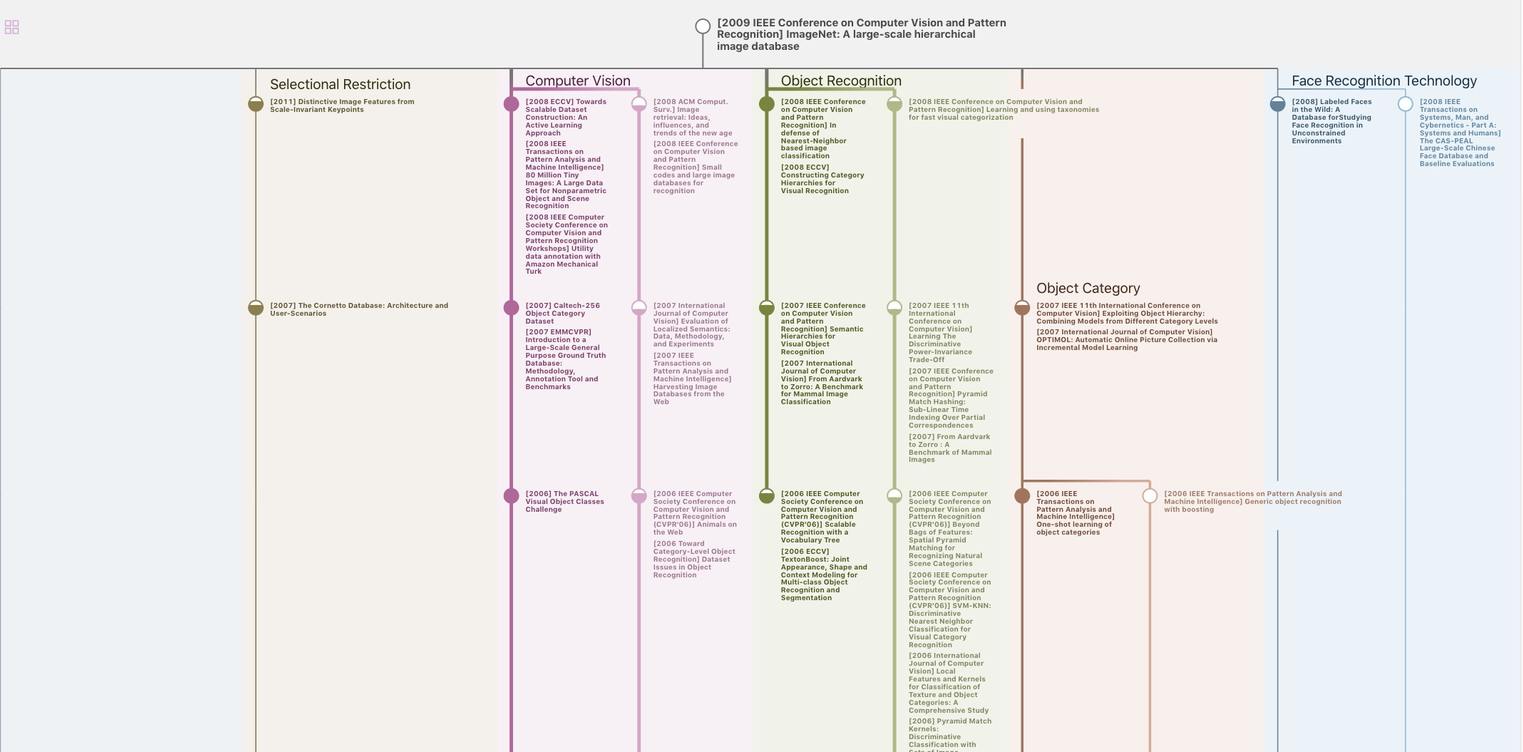
生成溯源树,研究论文发展脉络
Chat Paper
正在生成论文摘要