A quantitative study of the structure-activity relationship and molecular docking of 5.6.7-trimethoxy-N-aryl-2-styrylquinolin-4-amines as potential anticancer agents using quantum chemical descriptors and statistical methods
Journal of Molecular Structure(2022)
摘要
To find a safe and effective anticancer drug, a huge number of inhibitors are being designed. In this work, we opt to explore the relationship between the molecular structures and the anticancer activity to support the design of novel and potent anticancer agents. A set of 48 of 5.6.7-trimethoxy-N-aryl-2- styrylquinolin-4-amines compounds as a potential anticancer agent is applied for this goal. The density functional theory (DFT) with the B3LYP functional and the extended base 6–31 G is used to calculate the suitable molecular descriptorsto link them to the studied activity. 20 linear models were established by changing training set compounds and further applied in the test set to calculate predicted IC50 values of compounds. Each molecular structure was encoded by 14 descriptors. The prediction performance of each constructed model was subjected to various validation tests: internal validation, external validation, and Y-randomization. Model 16 is chosen with higher values of R2, R2test, and Q2.The strong correlation between the experimental and predicted activity values proves the validity and reliability of the obtained QSAR model. The best proposed QSAR model shows that there are three descriptors: ELUMO, MW, POLZ govern the anticancer activity. According to the picked QSAR model, some design of new molecules with desired activity has been proposed. This study could aid scientists in forecasting the inhibitory activity of novel or untested compounds before their synthesis. Molecular docking simulations of compounds 42 and 43 with Chk1 protein were also performed, and the two compounds exhibited reasonable molecular interactions with favorable docking scores.
更多查看译文
关键词
QSAR studies,Molecular docking,Chk1 protein,Quinoline derivatives
AI 理解论文
溯源树
样例
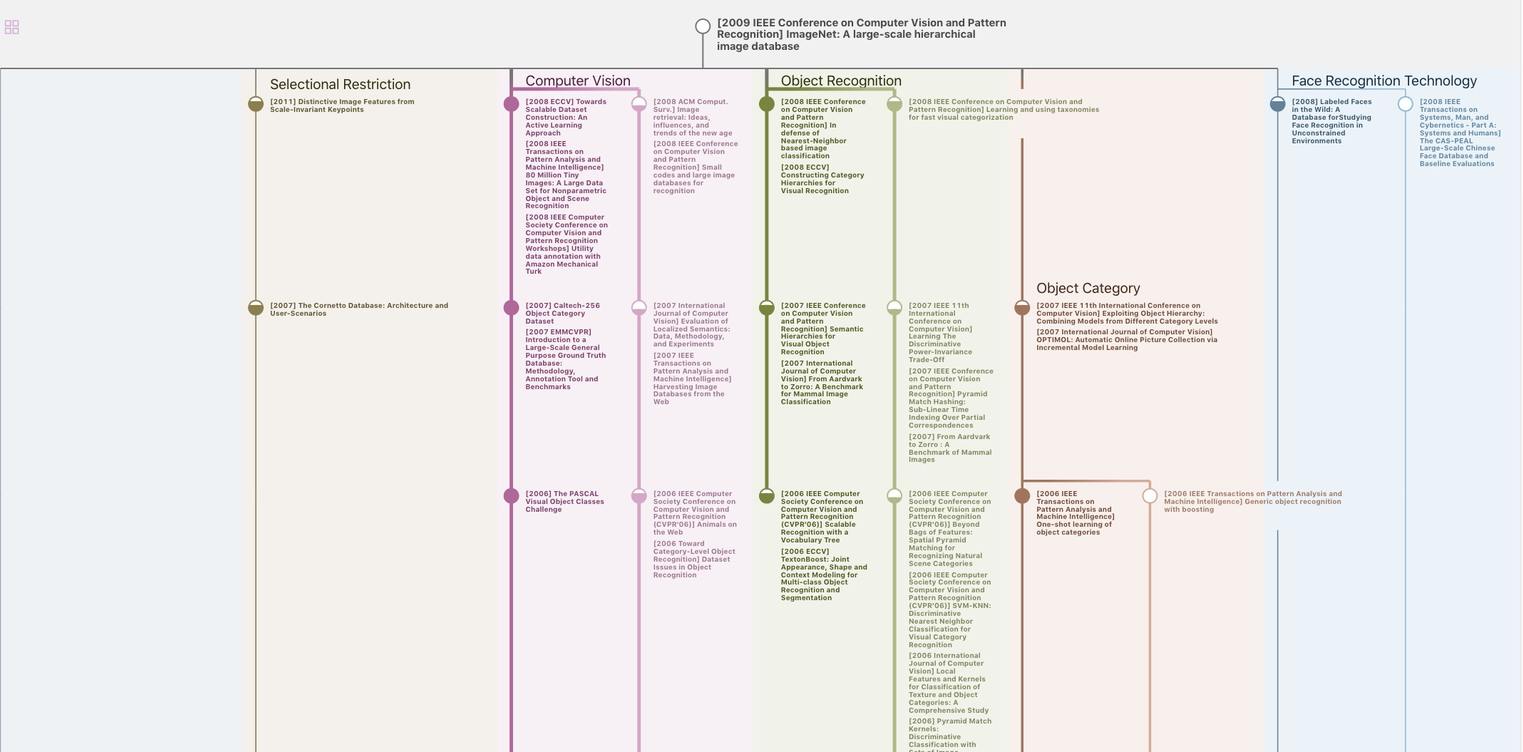
生成溯源树,研究论文发展脉络
Chat Paper
正在生成论文摘要