Multivariate Time Series Imputation: an Approach Based on Dictionary Learning.
Entropy(2022)
摘要
The problem addressed by dictionary learning (DL) is the representation of data as a sparse linear combination of columns of a matrix called dictionary. Both the dictionary and the sparse representations are learned from the data. We show how DL can be employed in the imputation of multivariate time series. We use a structured dictionary, which is comprised of one block for each time series and a common block for all the time series. The size of each block and the sparsity level of the representation are selected by using information theoretic criteria. The objective function used in learning is designed to minimize either the sum of the squared errors or the sum of the magnitudes of the errors. We propose dimensionality reduction techniques for the case of high-dimensional time series. For demonstrating how the new algorithms can be used in practical applications, we conduct a large set of experiments on five real-life data sets. The missing data (MD) are simulated according to various scenarios where both the percentage of MD and the length of the sequences of MD are considered. This allows us to identify the situations in which the novel DL-based methods are superior to the existing methods.
更多查看译文
关键词
multivariate time series,missing data,imputation,dictionary learning,information theoretic criteria
AI 理解论文
溯源树
样例
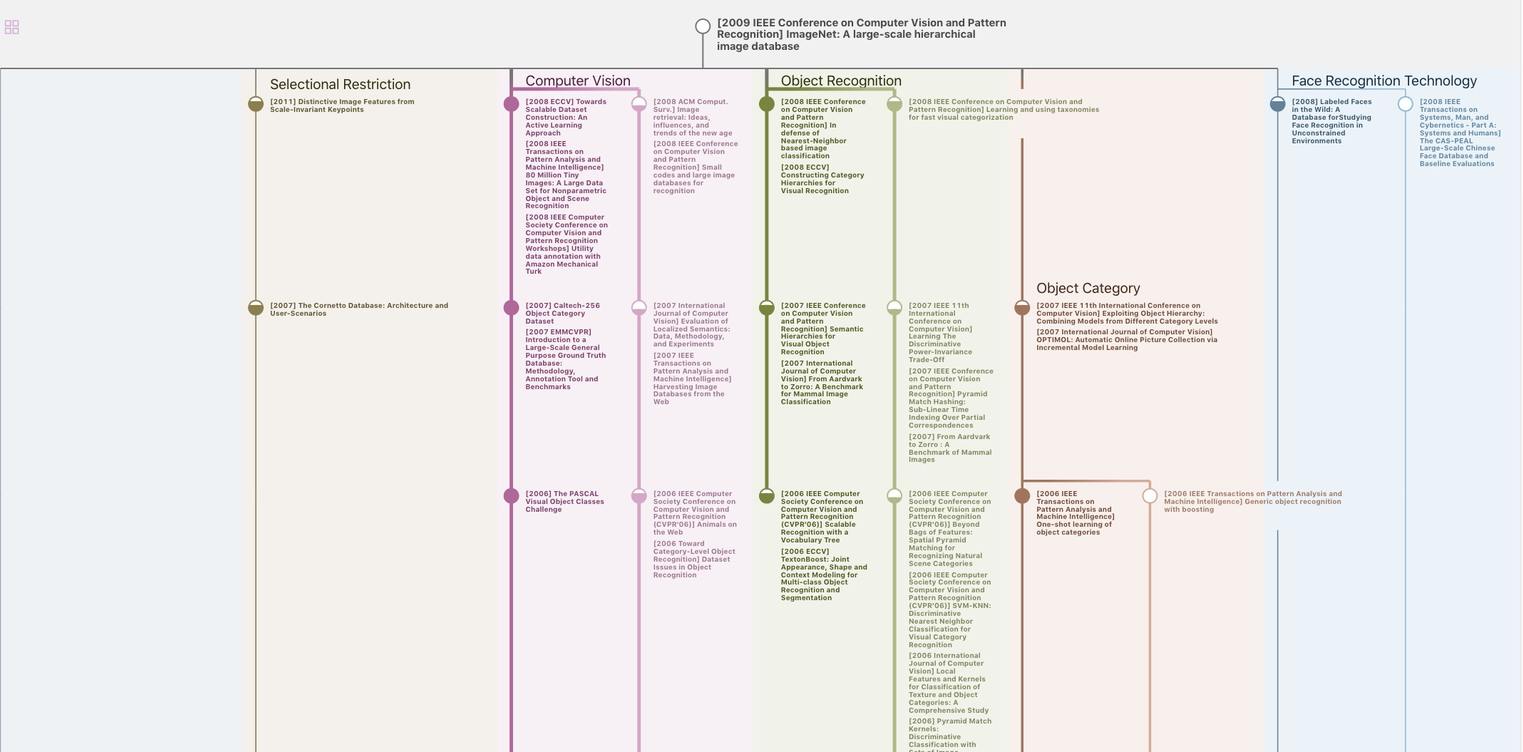
生成溯源树,研究论文发展脉络
Chat Paper
正在生成论文摘要