VIDEO-BASED TABLE TENNIS TRACKING AND TRAJECTORY PREDICTION USING CONVOLUTIONAL NEURAL NETWORKS
FRACTALS-COMPLEX GEOMETRY PATTERNS AND SCALING IN NATURE AND SOCIETY(2022)
摘要
One of the fascinating aspects of sports rivalry is that anything can happen. The significant difficulty is that computer-aided systems must address how to record and analyze many game events, and fractal AI plays an essential role in dealing with complex structures, allowing effective solutions. In table tennis, we primarily concentrate on two issues: ball tracking and trajectory prediction. Based on these two components, we can get ball parameters such as velocity and spin, perform data analysis, and even create a ping-pong robot application based on fractals. However, most existing systems rely on a traditional method based on physical analysis and a non-machine learning tracking algorithm, which can be complex and inflexible. As mentioned earlier, to overcome the problem, we proposed an automatic table tennis-aided system based on fractal AI that allows solving complex issues and high structural complexity of object tracking and trajectory prediction. For object tracking, our proposed algorithm is based on structured output Convolutional Neural Network (CNN) based on deep learning approaches and a trajectory prediction model based on Long Short-Term Memory (LSTM) and Mixture Density Networks (MDN). These models are intuitive and straightforward and can be optimized by training iteratively on a large amount of data. Moreover, we construct a table tennis auxiliary system based on these models currently in practice.
更多查看译文
关键词
Table Tennis, Deep Learning, Object Tracking, Trajectory, Fractal AI Prediction
AI 理解论文
溯源树
样例
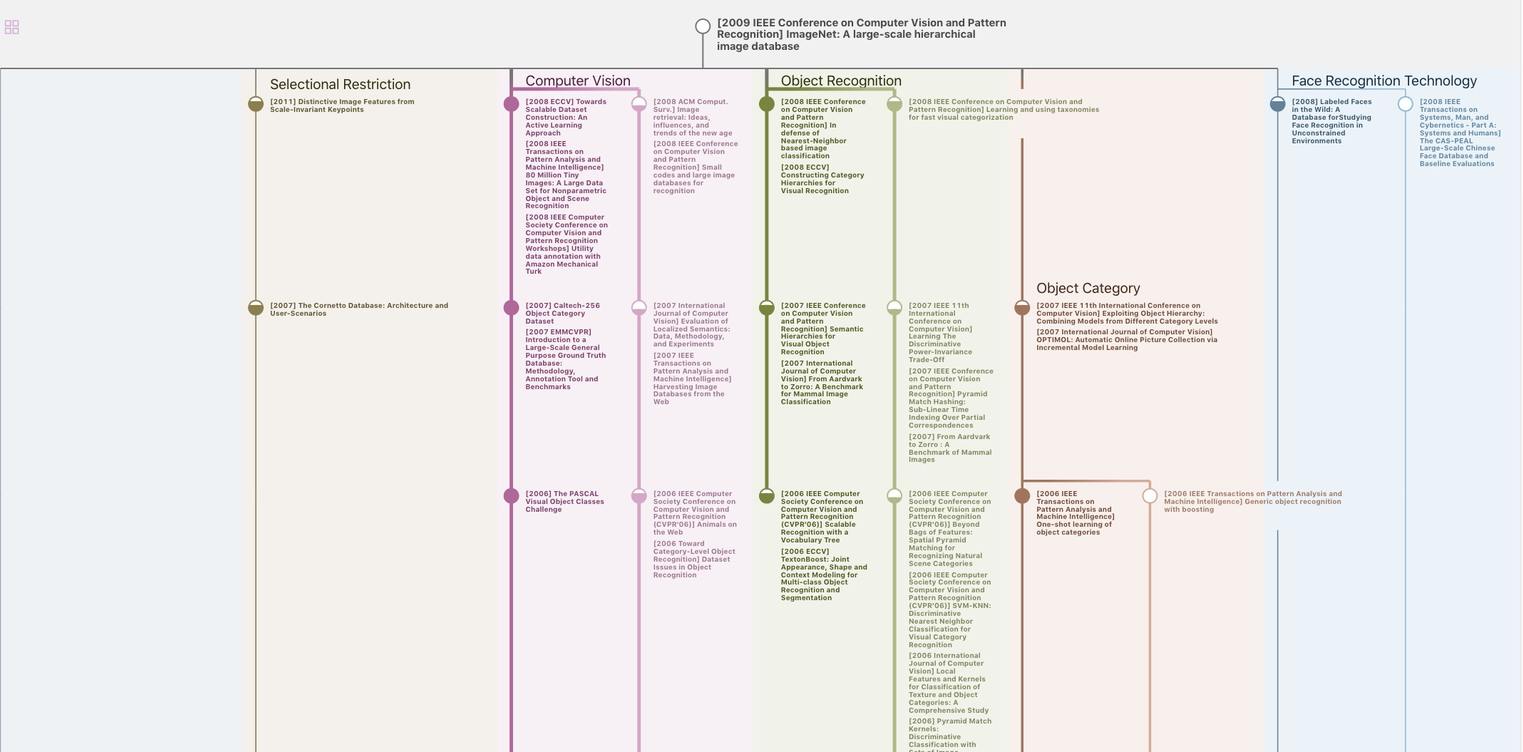
生成溯源树,研究论文发展脉络
Chat Paper
正在生成论文摘要