Meta-FSDet: a meta-learning based detector for few-shot defects of photovoltaic modules
JOURNAL OF INTELLIGENT MANUFACTURING(2022)
摘要
In the initial stage of the establishment of photovoltaic (PV) module production lines or the upgrading of production processes, the available data for some defects are limited. The detection for these data-scarce defects of photovoltaic (PV) modules is a challenging task, because most detectors hardly extract meaningful high-level features from limited information of several samples. To address this challenge, this paper proposes a novel end-to-end meta-learning based few-shot detector (Meta-FSDet). Firstly, a prototype vector extractor (PVE) is proposed, which utilizes locational prior knowledge of data-scarce defects to extract corresponding prototype vectors. Then, a novel saliency highlighting network (SHN), which measures the similarity between extracted prototype vectors from PVE and each spatial-position vector of the query feature map, is proposed to infer a class-agnostic saliency map, in which the defective areas are well highlighted. Next, a remodel region proposal network (RRPN), which further leverages the saliency map from SHN to pixel-wisely reweight the inputted feature map of region proposal network (RPN), is presented to better infer meaningful high-level region-of-interest (RoI) features of data-scarce defects. Finally, these RoI features from RRPN are further processed by downstream classification and regression module to generate final prediction results. Extensive experiments on PV module dataset demonstrate our Meta-FSDet owns superior performance compared to other existing methods.
更多查看译文
关键词
Photovoltaic module,Data-scarce defects,Few-shot detection,Deep learning,Defect inspection
AI 理解论文
溯源树
样例
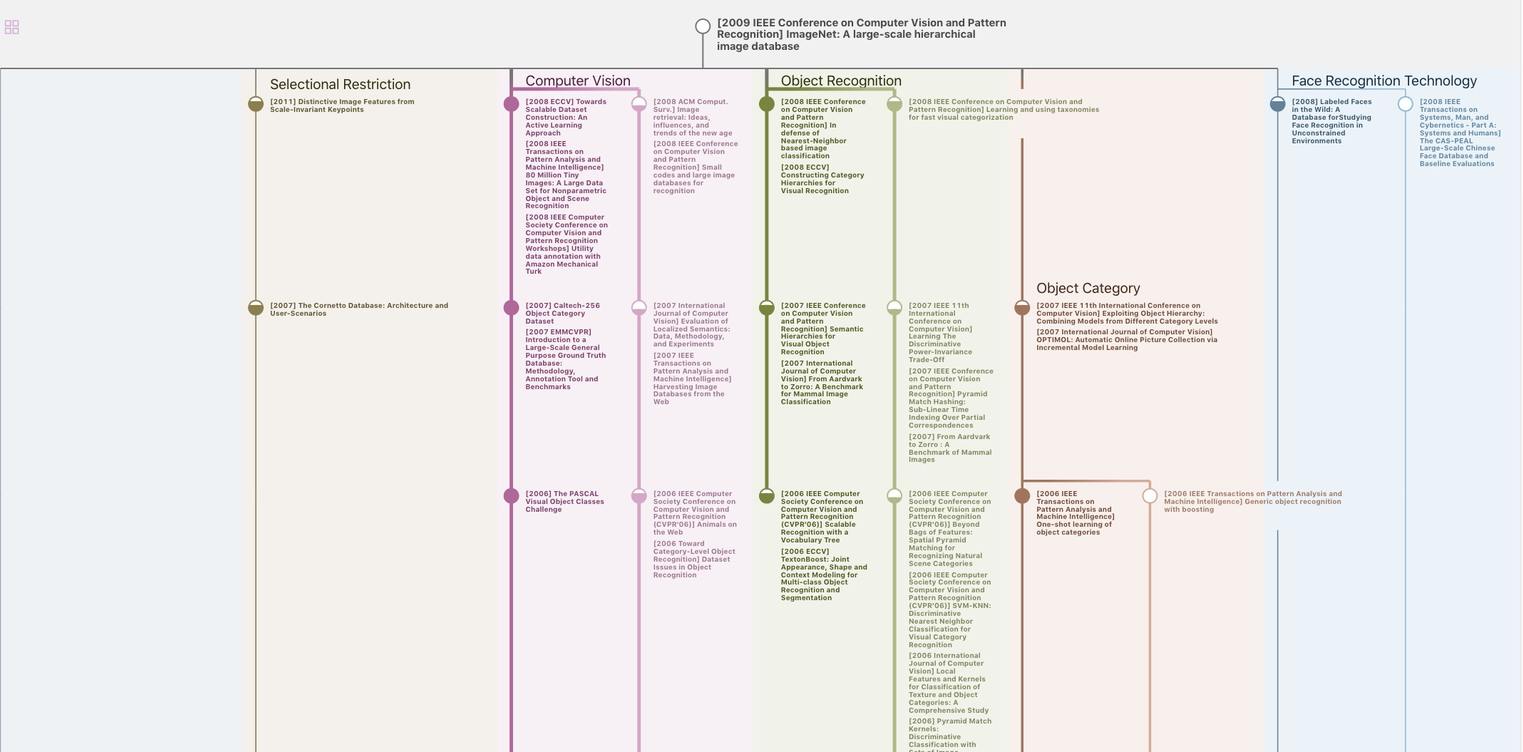
生成溯源树,研究论文发展脉络
Chat Paper
正在生成论文摘要