Pediatric CT Cranial Radiation Dose Estimation Using Deep Learning
MEDICAL IMAGING 2022: PHYSICS OF MEDICAL IMAGING(2022)
摘要
Background: Ionizing radiation is used in X-ray CT (Computed Tomography) imaging systems to produce in vivo images of the human body. Due to the inherent nature of these photons, the quantity of energy deposited in the body is measured as radiation dose. Estimating the patient's dose is especially important in pediatric patients where unnecessary radiation exposure should be limited. Generating patient specific dose estimates typically employ Monte-Carlo (MC) simulations, which are computationally intensive and time consuming, on the order of hours. Here we propose a method that utilizes machine learning with neural networks (NN) to distill MC simulations and produce a comparable dose map, on the order of seconds. Methods: Monte-Carlo simulations were performed using the MC-GPU simulation package, then the estimates were used to train the NN. 400 pediatric patients were used in training and a separate set of 256 pediatric patients were used in validation. The network is designed as a convolutional U-net with skip connections and was implemented in Python 3.7 with Tensorflow 2.1 and Keras 2.4. Results: NN dose estimates of the validation data set were compared to the ground truth MC simulations and generated regression slopes of 1.0 and y intercepts of 0.0 with an r2 larger than 0.99. The NN demonstrated that the standard deviations between the simulated and predicted total cranial doses are less than 0.5 mGy for the validation data set. Conclusions: Patient specific dose estimates generated from NNs can be produced accurately and quickly enough to be performed during CT acquisition.
更多查看译文
关键词
Pediatric, Dose, Estimation, Monte-Carlo, Deep Learning, Neural Networks, Machine Learning
AI 理解论文
溯源树
样例
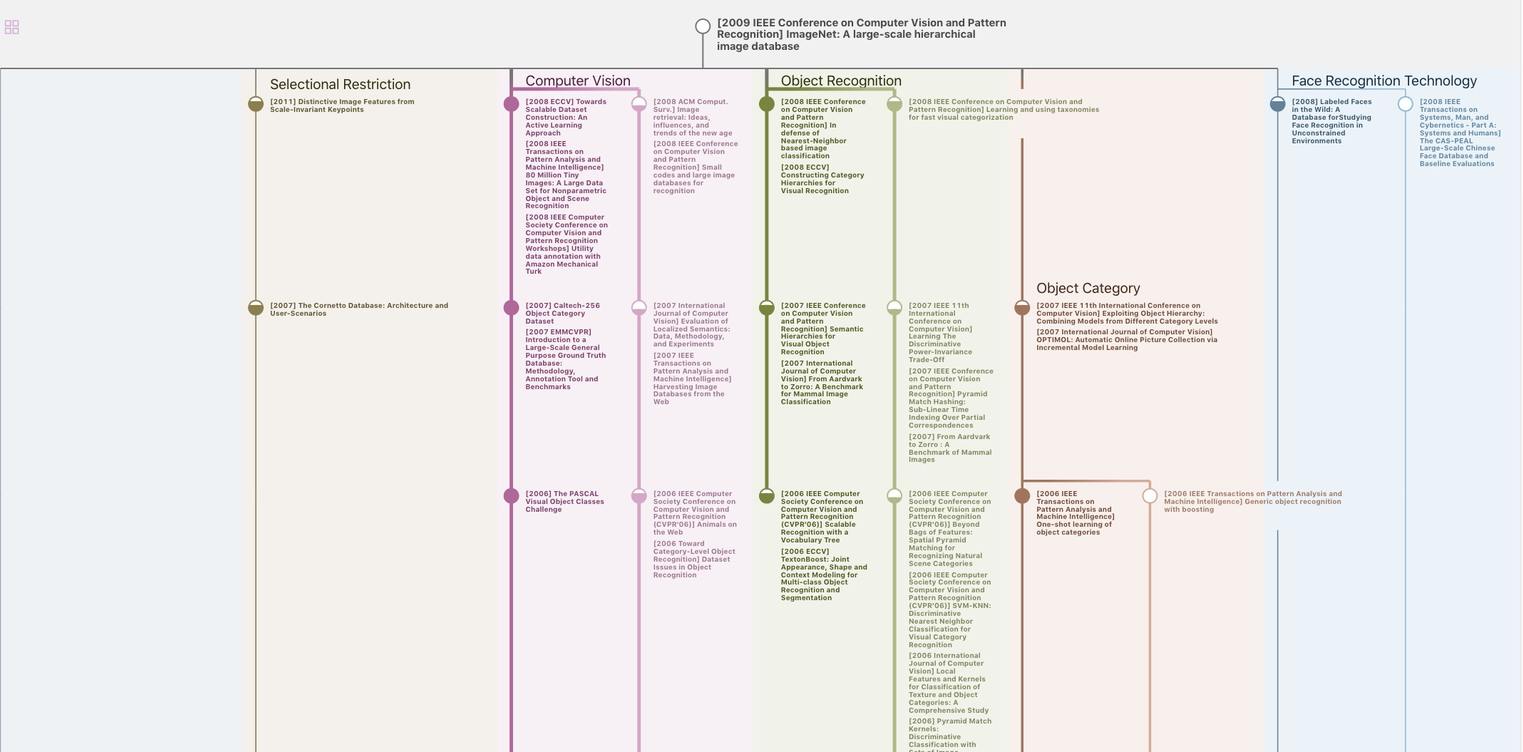
生成溯源树,研究论文发展脉络
Chat Paper
正在生成论文摘要