Impact Evaluation of Deep learning on Image Segmentation for Automatic Bluefin Tuna Sizing
Aquacultural Engineering(2022)
摘要
This paper evaluates the impact of using deep learning techniques in an automatic fish sizing process. Automatic fish sizing with a non-invasive approach involves working with different views of the fish's body and changing environments, being the stage of extraction of individuals in the image and the quality of the segmentation essential to obtain good sizing measurements. The goal of this work is to improve the results and functionality achieved in our previous studies with conventional segmentation methods based on local thresholding, where different limitations were observed, mainly the necessity of parameters tuning and a high computational cost. The number of detections must also increase significantly to increase the reliability of the statistical results. An approach using convolutional neural networks is proposed for fish detection and segmentation in videos acquired under real conditions, which eliminates the engineering procedure of parameter adjustment and generalises the solution for fish segmentation to deal with different environmental conditions (illumination and water turbidity) and background variability. The results show that the fish sizing procedure is enhanced thanks to the improvement in fish image instance segmentation. In particular, the number of fish measurements increases by up to 2.45 times when using Mask R-CNN and the PointRend module, thus increasing the accuracy of the fish length estimation, and the number of measurements per minute of computing time increases by up to 3.5 times. Our proposal obtains highly accurate fish length estimations in juvenile bluefin tuna based on a stereoscopic vision system and a deformable model of the fish's silhouette, both from the ventral and dorsal perspectives. An important improvement is achieved by applying CNN, as demonstrated by the number of segmented instances, the time required to segment an instance, and the accuracy of the fish sizing achieved.
更多查看译文
关键词
Underwater stereo vision,Computer vision,Fishery management,Automatic fish sizing,Biomass estimation,Convolutional neural networks
AI 理解论文
溯源树
样例
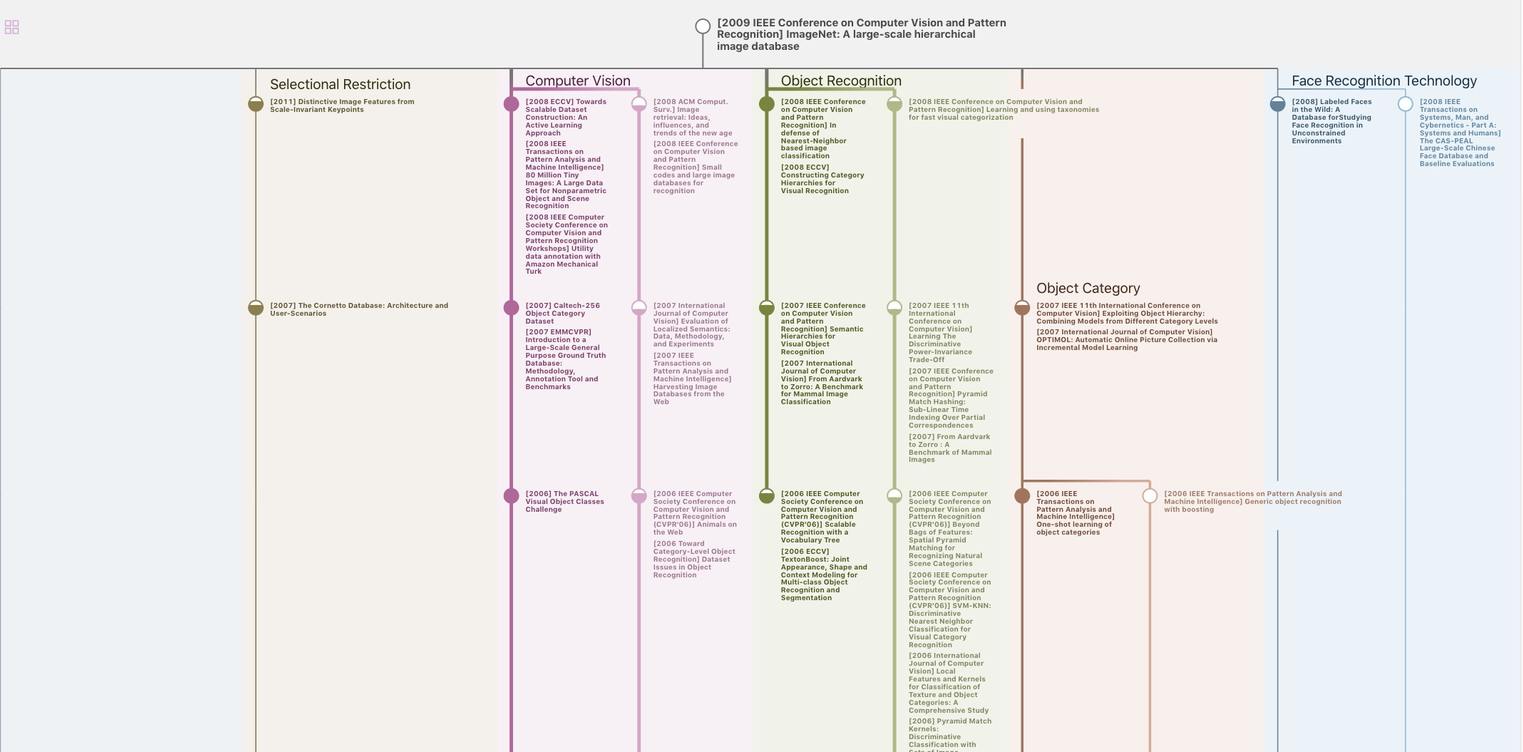
生成溯源树,研究论文发展脉络
Chat Paper
正在生成论文摘要