Coil optimization of ultra-deep azimuthal electromagnetic resistivity logging while drilling tool based on numerical simulation
Journal of Petroleum Exploration and Production Technology(2022)
摘要
This paper presents a coil optimization method of the ultra-deep azimuthal electromagnetic (EM) resistivity Logging While Drilling (LWD) to obtain the best parameters of the tool for formation boundary detection by theory. First, a rapid numerical simulation algorithm, i.e. the Fast Hankel Transform (FHT) method, is developed to simulate the logging responses of the layered formation. A system of double inclined coils is selected based on the response characteristics and the depth of detection (DOD) from four common coils. Simulation results demonstrated that the optimal coils are perfectly suitable for anisotropic formation. Then, the crucial parameters of the frequency and spacing of the selected coils with a DOD of 30 m are determined using the Picasso diagram. In addition, the influences of the electrical parameter of the drilling collar and resistivity anisotropy of the formation on the response are investigated. The results show that the conductivity of the drilling collar, the thickness and the resistivity anisotropy of the formation all affect the DOD. Finally, formation models with different layers are created to verify the performance and signal intensity. The results demonstrate that the optimized coils not only have a large DOD but also possess a high signal intensity, further confirming the advantages of the preferred coils. Compared with the conventional physical experiment tests, the method of coil optimization proposed in this paper is more economical and efficient. The findings of this study can help better understand the detection characteristics of the different coils, which can provide theoretical support for designing and developing a tool with the best boundary capability.
更多查看译文
关键词
Ultra-deep azimuthal EM resistivity, LWD, Coils optimization, DOD, Numerical simulation, Fast Hankel Transform, FEM
AI 理解论文
溯源树
样例
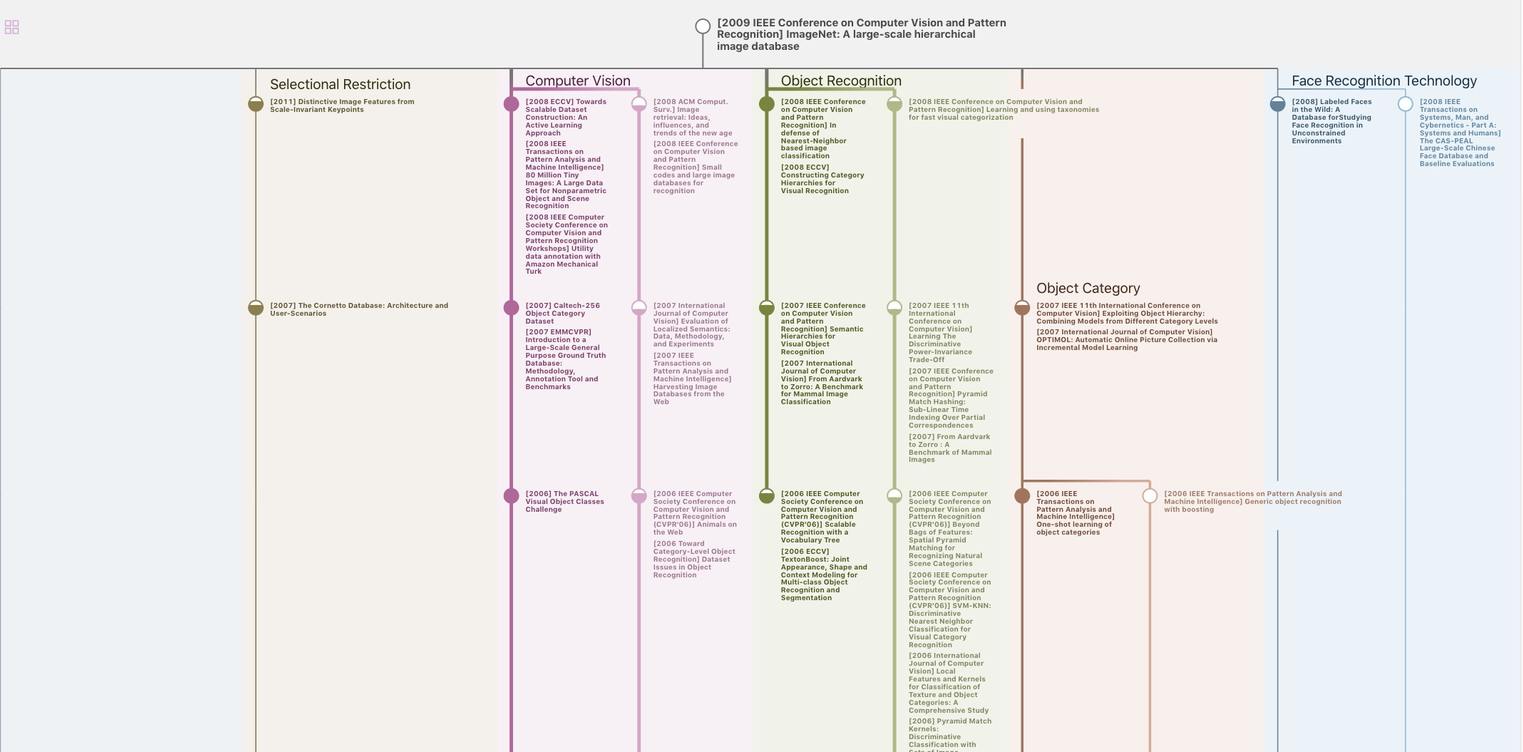
生成溯源树,研究论文发展脉络
Chat Paper
正在生成论文摘要