Overall Assessment for Selected Markers from High-Throughput Data.
Statistics in medicine(2022)
摘要
Reproducibility, a hallmark of science, is typically assessed in validation studies. We focus on high-throughput studies where a large number of biomarkers is measured in a training study, but only a subset of the most significant findings is selected and re-tested in a validation study. Our aim is to get the statistical measures of overall assessment for the selected markers, by integrating the information in both the training and validation studies. Naive statistical measures, such as the combined P $$ P $$ -value by conventional meta-analysis, that ignore the non-random selection are clearly biased, producing over-optimistic significance. We use the false-discovery rate (FDR) concept to develop a selection-adjusted FDR (sFDR) as an overall assessment measure. We describe the link between the overall assessment and other concepts such as replicability and meta-analysis. Some simulation studies and two real metabolomic datasets are considered to illustrate the application of sFDR in high-throughput data analyses.
更多查看译文
关键词
false discovery rate,reproducibility,selection adjustment,validation study
AI 理解论文
溯源树
样例
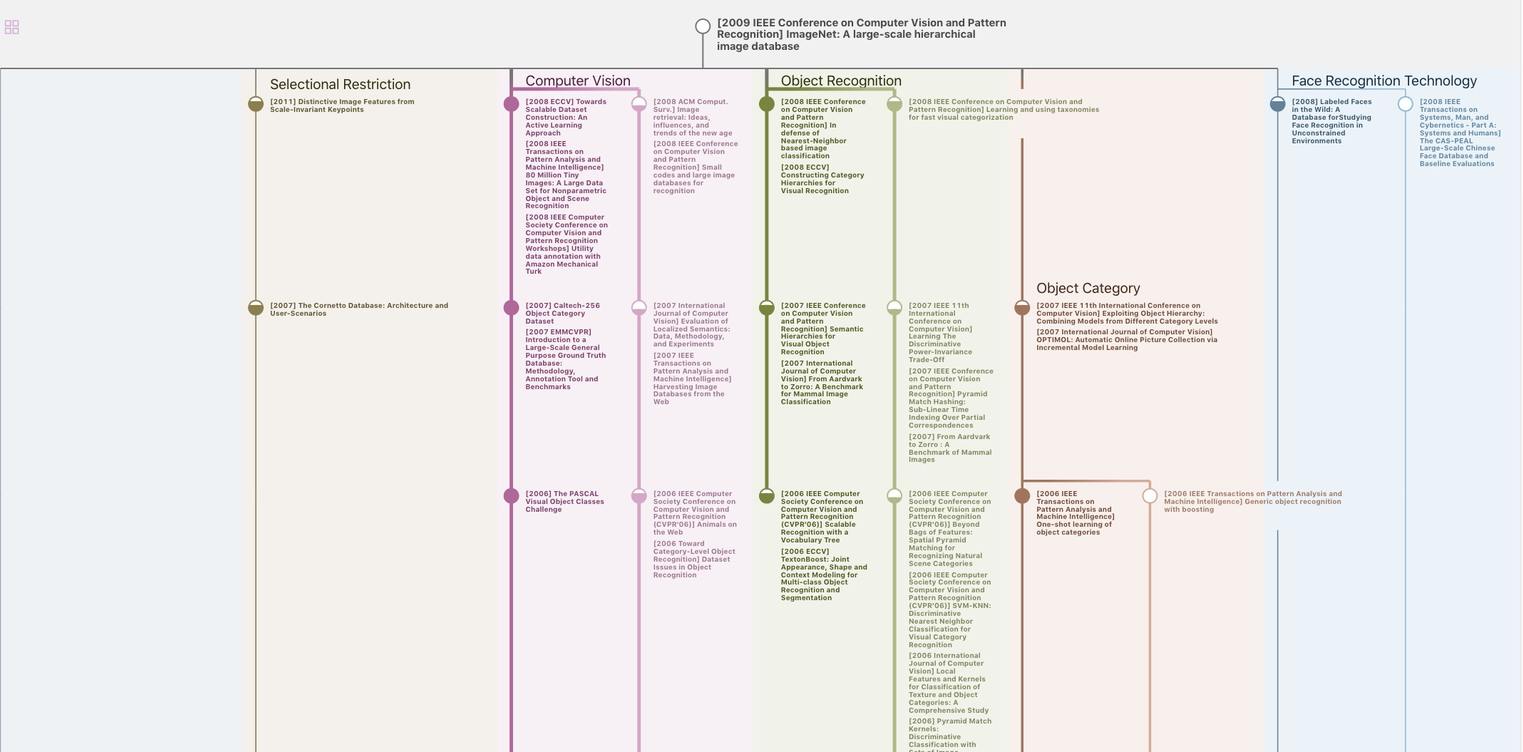
生成溯源树,研究论文发展脉络
Chat Paper
正在生成论文摘要