How Cerebellar Architecture and Dense Activation Patterns Facilitate Online Learning in Dynamic Tasks
biorxiv(2023)
摘要
The cerebellum has a distinctive architecture in which inputs undergo a massive size expansion in dimensionality in early layers. Marr and Albus’s classic codon theory and more recent extensions[1][1]–[4][2] argue that this architecture facilitates learning via pattern separation. The essence of this idea is that sparsely active clusters —‘codons’— of inputs are more easily separable in a higher dimensional representation. However, recent physiological data indicate that cerebellar activity is not sparse in the way anticipated by codon theory. Moreover, there is a conceptual gap between static pattern separation and the critical role of the cerebellum in dynamic tasks such as motor learning. We use mathematical analysis and simulations of cerebellar learning to identify specific difficulties inherent to online learning of dynamic tasks. We find that size expansions directly mitigate these difficulties, and that this benefit is maximised when granule cell activity is dense.
### Competing Interest Statement
The authors have declared no competing interest.
[1]: #ref-1
[2]: #ref-4
更多查看译文
AI 理解论文
溯源树
样例
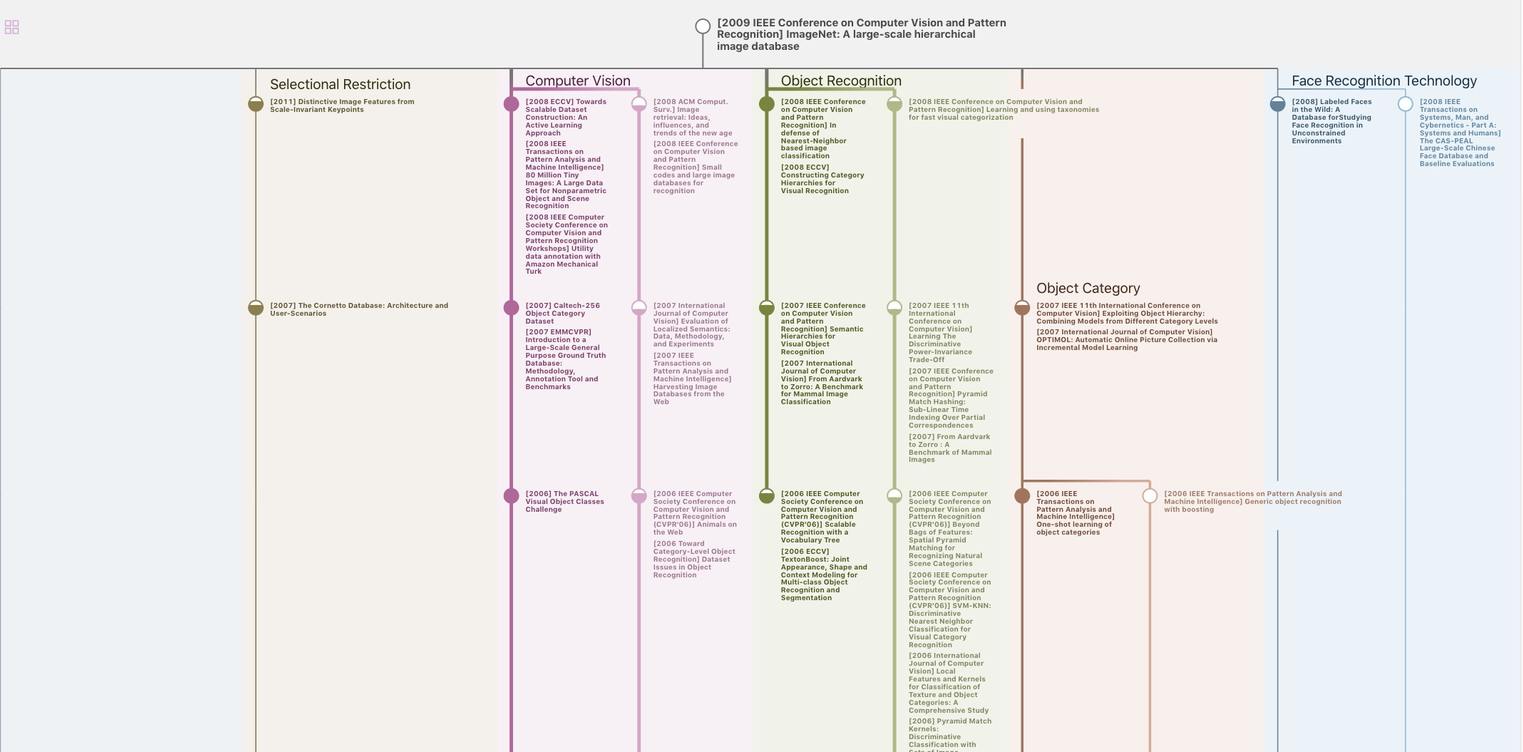
生成溯源树,研究论文发展脉络
Chat Paper
正在生成论文摘要