Insitutype: likelihood-based cell typing for single cell spatial transcriptomics
biorxiv(2022)
摘要
Accurate cell typing is fundamental to analysis of spatial single-cell transcriptomics, but legacy scRNA-seq algorithms can underperform in this new type of data. We have developed a cell typing algorithm, Insitutype, designed for statistical and computational efficiency in spatial transcriptomics data. Insitutype is based on a likelihood model that weighs the evidence from every expression value, extracting all the information available in each cell's expression profile. This likelihood model underlies a Bayes classifier for supervised cell typing, and an Expectation-Maximization algorithm for unsupervised and semi-supervised clustering. Insitutype also leverages alternative data types collected in spatial studies, such as cell images and spatial context, by using them to inform prior probabilities of cell type calls. We demonstrate rapid clustering of millions of cells and accurate fine-grained cell typing of kidney and non-small cell lung cancer samples.
### Competing Interest Statement
PD, EZ, ZY, DR, MG, ZR, TKK, SH, DH and JMB are current/former employees and shareholders of NanoString.
更多查看译文
关键词
spatial transcriptomics,single cell,cell typing,likelihood-based
AI 理解论文
溯源树
样例
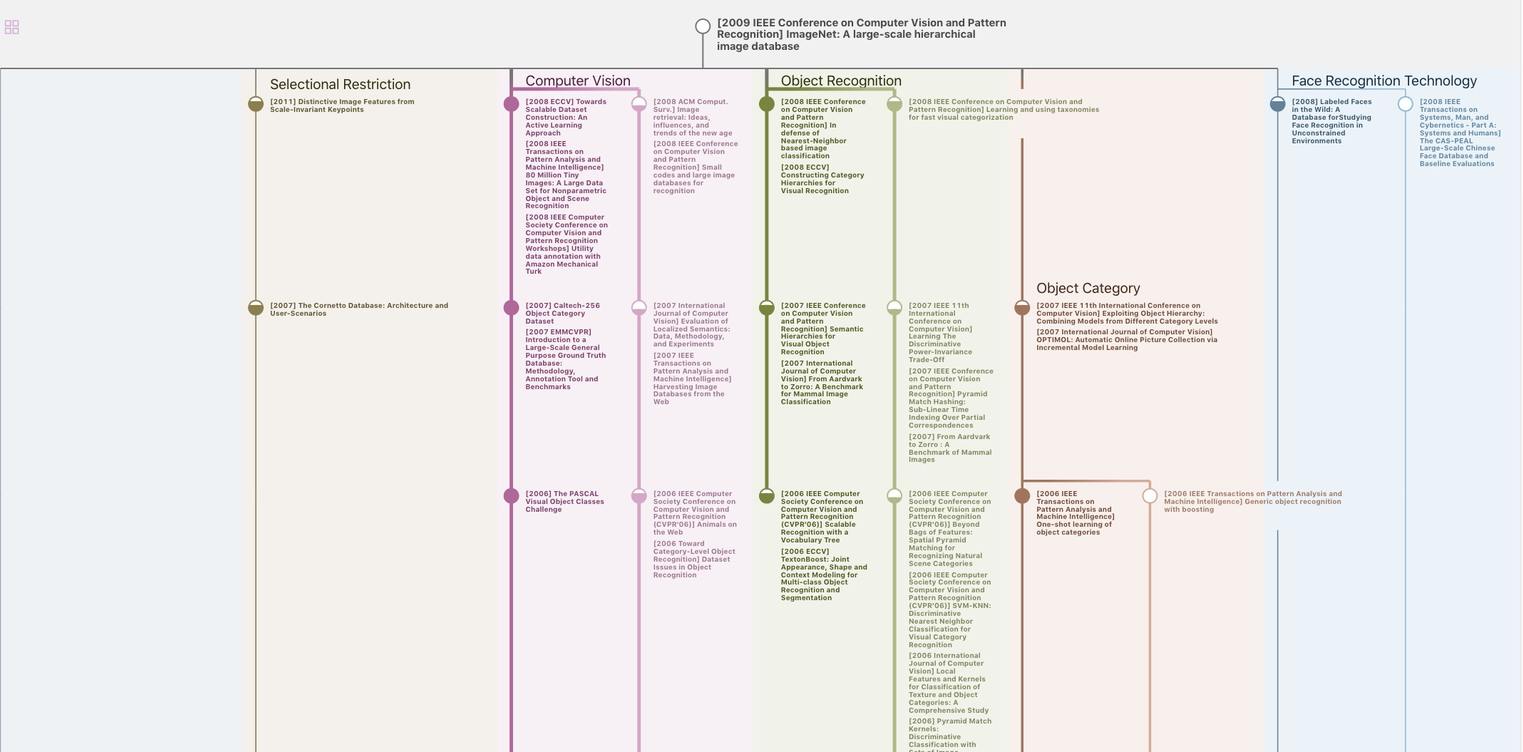
生成溯源树,研究论文发展脉络
Chat Paper
正在生成论文摘要