Clinical Applications of Machine Learning for Urolithiasis and Benign Prostatic Hyperplasia: A Systematic Review.
Journal of endourology(2023)
摘要
Previous systematic reviews related to machine learning (ML) in urology often overlooked the literature related to endourology. Therefore, we aim to conduct a more focused systematic review examining the use of ML algorithms for the management of benign prostatic hyperplasia (BPH) or urolithiasis. In addition, we are the first group to evaluate these articles using the Standardized Reporting of Machine Learning Applications in Urology (STREAM-URO) framework. Searches of MEDLINE, Embase, and the Cochrane CENTRAL databases were conducted from inception through July 12, 2021. Keywords included those related to ML, endourology, urolithiasis, and BPH. Two reviewers screened the citations that were eligible for title, abstract, and full-text screening, with conflicts resolved by a third reviewer. Two reviewers extracted information from the studies, with discrepancies resolved by a third reviewer. The data collected were then qualitatively synthesized by consensus. Two reviewers evaluated each article according to the STREAM-URO checklist with discrepancies resolved by a third reviewer. After identifying 459 unique citations, 63 articles were retained for data extraction. Most articles consisted of tabular ( = 32) and computer vision ( = 23) tasks. The two most common problem types were classification ( = 40) and regression ( = 12). In general, most studies utilized neural networks as their ML algorithm ( = 36). Among the 63 studies retrieved, 58 were related to urolithiasis and 5 focused on BPH. The urolithiasis studies were designed for outcome prediction ( = 20), stone classification ( = 18), diagnostics ( = 17), and therapeutics ( = 3). The BPH studies were designed for outcome prediction ( = 2), diagnostics ( = 2), and therapeutics ( = 1). On average, the urolithiasis and BPH articles met 13.8 (standard deviation 2.6), and 13.4 (4.1) of the 26 STREAM-URO framework criteria, respectively. The majority of the retrieved studies effectively helped with outcome prediction, diagnostics, and therapeutics for both urolithiasis and BPH. While ML shows great promise in improving patient care, it is important to adhere to the recently developed STREAM-URO framework to ensure the development of high-quality ML studies.
更多查看译文
关键词
benign prostatic hyperplasia,endourology,machine learning,urolithiasis
AI 理解论文
溯源树
样例
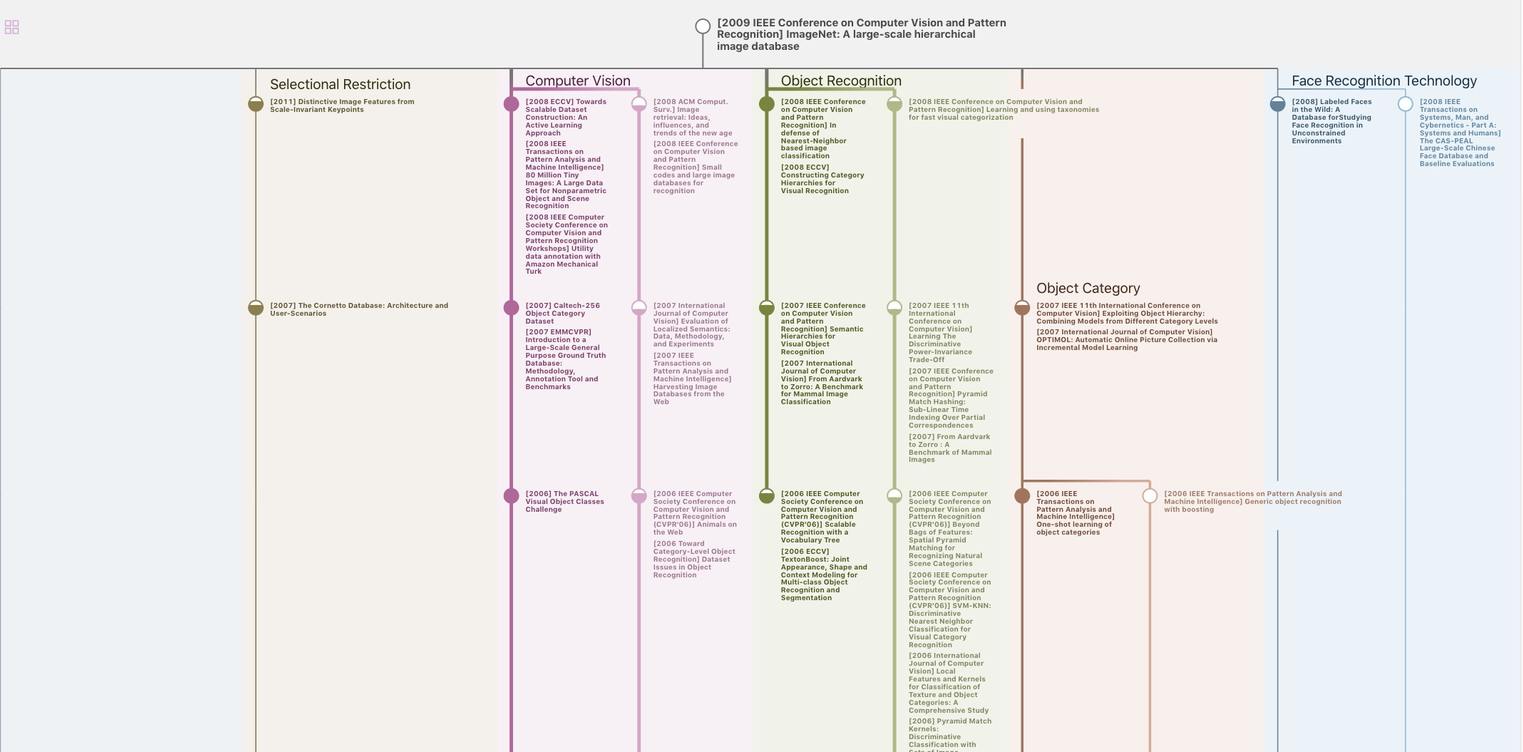
生成溯源树,研究论文发展脉络
Chat Paper
正在生成论文摘要