Synthetic FDG-Positron Emission Tomography Images for Patients with Non-Small Cell Lung Cancer: A Deep Learning-Based Approach Using Computed Tomography Images
International Journal of Radiation Oncology*Biology*Physics(2022)
摘要
Purpose/Objective(s)
Deep learning-based, medical image-to-image transformation has been limited so far to computed tomography (CT) / magnetic resonance (MR) translation, and few studies have been reported on Positron Emission Tomography (PET) synthesis. Synthetic 18F-FDG-PET (sFDG-PET) image from CT provides the advantage of lowering exposure, cost, and labor, and facilitating longitudinal follow-up, particularly for patients with limited access to a PET scanner. Furthermore, CT can be performed on demand, whereas FDG-PET entails advanced preparation. Nevertheless, sFDG-PET generation faces the impediment of determining a valid anatomy-to-function transformation. In this work, we present a novel, deep learning-based sFDG-PET generation method from lung CT images.Materials/Methods
An IRB-approved retrospective study was conducted at our institution for patients undergoing stereotactic body radiotherapy for early-stage non-small cell lung cancer. For each patient, CT, measured PET images, and planning target (PTV) volumes were extracted using COMKAT Image Tool. A workflow to generate sFDG-PET images as outputs from CT inputs, referred to as 2C-UcGAN, was developed based on conditional generative adversarial network design, and consisted of a U-Net generator accepting a 2channel-input: axial CT slices as input in channel 1, and mediastinal window-adjusted CT slices in channel 2. Training patches (size = 128 × 128 × 128) were centered around the PTV, and training was performed on an Intel workstation, 128G of RAM, NVIDIA TITAN XP GPU, Epoch = 50, using a programming environment. Standardized uptake values (SUVs) were calculated. A classic pix2pix network was used as a benchmark for comparison. Mean absolute error (MAE), root mean square error (RMSE), and correlation coefficient (R) of SUVs normalized to body weight were compared, in addition to sensitivity (Sen), specificity (Spe), positive and negative predictive values (PPV and NPV) for PTV uptake.Results
A total of 165 patients were included and randomly divided into training (n=100), validation (n=19), and testing (n=46). Among the testing patients, 15 nodules were not 18F-FDG-PET avid. Results are listed in Table 1. Prediction time was in the range of a second. 2C-UcGAN outperformed pix2pix with a MAE of 1.21 g/mL and yielded higher PTV Sen and PPV than pix2pix, with acceptable Spe and NPV.Conclusion
Using an intelligent combination of windowed CT radiological features, promising results are attained for metabolic characteristic prediction. The achieved MAE presents a considerable improvement in accuracy compared to the previously reported algorithm. Given its potential for implementing CT-only follow-up, further studies are warranted across different patient populations.查看译文
关键词
lung cancer,cell lung cancer,fdg-positron,non-small,learning-based
AI 理解论文
溯源树
样例
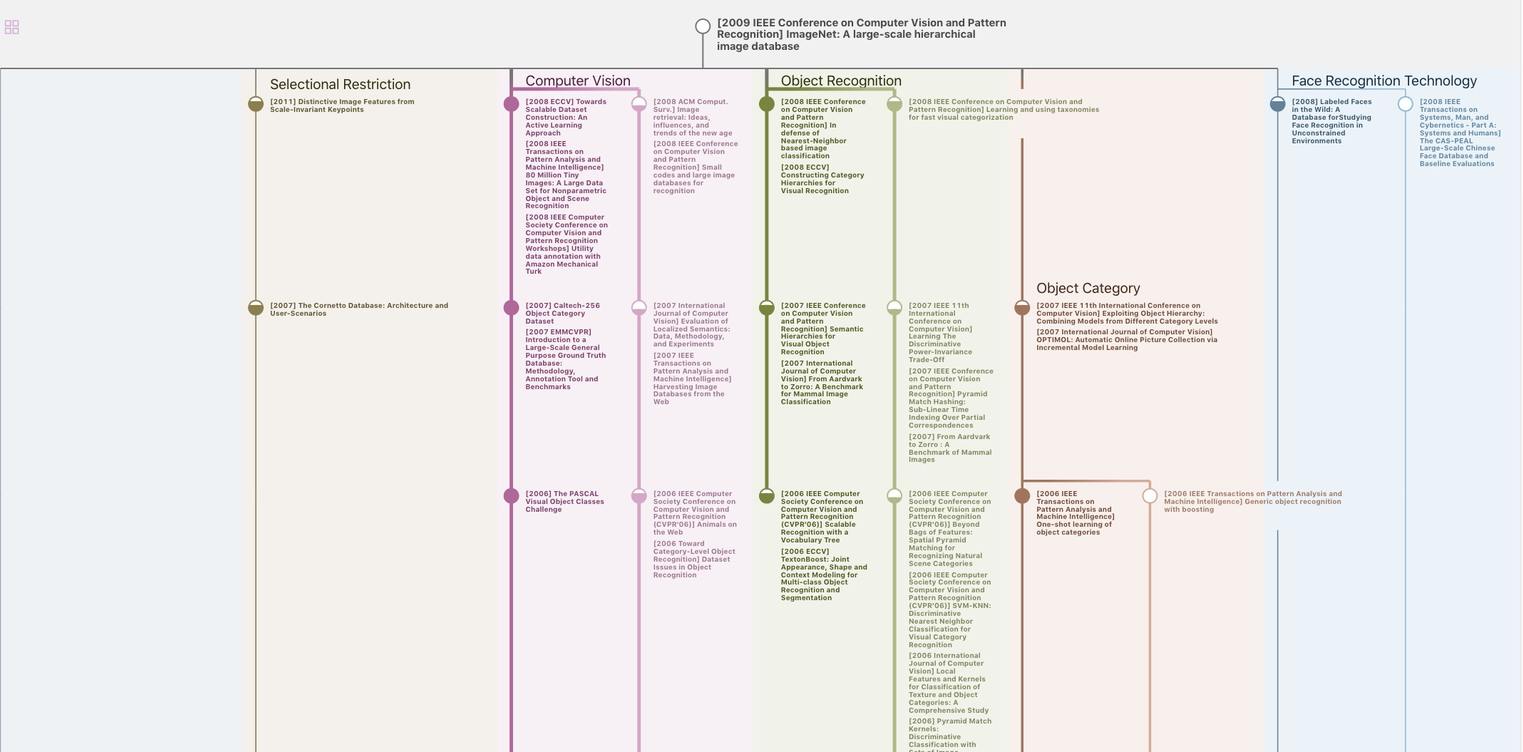
生成溯源树,研究论文发展脉络
Chat Paper
正在生成论文摘要