Hyper clustering model for dynamic network intrusion detection
IET Communications(2022)
摘要
Generally, the existing Intrusion Detection Systems (IDS) solutions suffer from low detection accuracy for some attack types compared with the overall detection accuracy of attacks. The data imbalance technically affects the ratio of detection accuracy of low frequent attacks class (e.g. zero-day attack) compared to attacks with more instances. Therefore, IDS-based machine learning algorithms potentially suffer from high false-positive rates. To overcome the limitation of existing solutions, a hyper-clustering model is proposed for dynamic intrusion detection based on the Density-Based Spatial Clustering of Applications with Noise (DBSCAN) and cosine similarity. The proposed solution develops the standard DBSCAN by adding a new evolving process based on distance measures between the clusters to overcome the imbalance dataset. Moreover, a new classifier is proposed based on cosine similarity to predict the labelling of abnormal behaviour. The experimental results show that the proposed model outperformed the original DBCAN and the related works. The mean silhouette of the proposed DBSCAN achieves a high score of 0.87 compared to other solutions. Furthermore, the proposed DBSCAN reduces the mean square error from 0.66 to 0.13 and achieves 86.82%, 79.10% and 90.03% in general accuracy on KDDTest+, KDDTest-21 NSL-KDD and UNSW-NB15 benchmark datasets, respectively.
更多查看译文
关键词
intrusion detection system,machine learning,clustering,DBSCAN
AI 理解论文
溯源树
样例
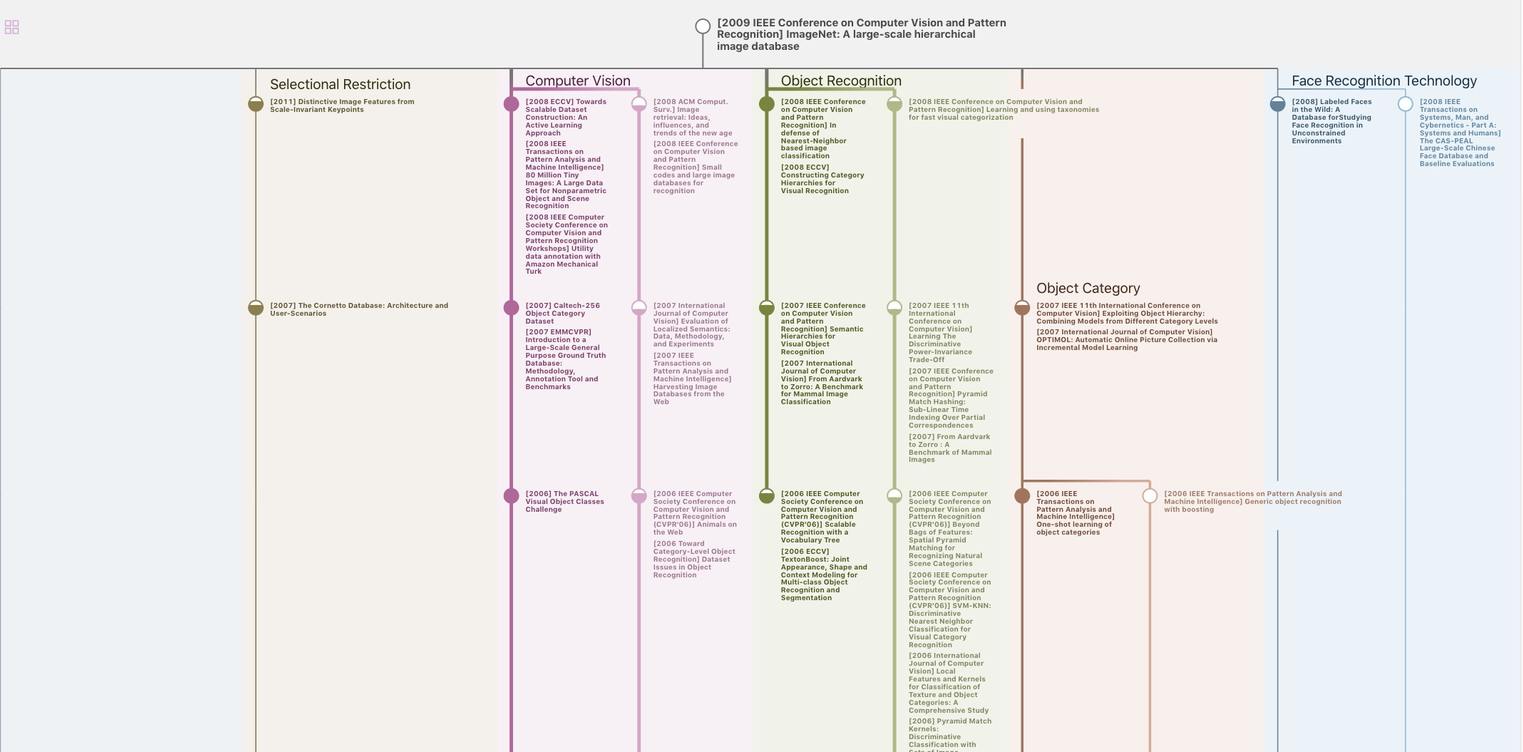
生成溯源树,研究论文发展脉络
Chat Paper
正在生成论文摘要