A Multi-Layer Auto-Segmentation Quality Assurance and Correction Pipeline for MR-Guided Adaptive Radiotherapy
International journal of radiation oncology, biology, physics(2022)
摘要
Purpose/Objective(s) Fast and accurate auto-segmentation on daily image is essential for MR-guided adaptive radiotherapy (MRgART). However, the state-of-the-art deep learning auto-segmentation (DLAS) still has limited success particularly for complex structures such as those in the abdomen. Inaccurate contours from DLAS need to be inspected and edited and this manual process can be labor intensive, time consuming and subject to human errors. This work aims to develop a multi-layer pipeline that includes an automatic contour quality assurance (ACQA) test, an automatic contour refinement (ACR) process, and an interactive semi-automatic segmentation (ISS) tool to quickly identify and correct for inaccurate auto-segmented contours, thus replacing the manual process in MRgART. Materials/Methods The proposed pipeline includes the ACQA, ACR and ISS modules that are developed to detect and correct for inaccurate auto-segmented contours in the following steps: (1) identifying contours/image slices of acceptable or with minor or major errors from the input auto-segmented contours using ACQA, (2) correcting the identified inaccurate contours/slices using ACR, (3) repeating steps (1) and (2) on the contours obtained from the previous ACR until the contours/slices become acceptable or the performance of ACR becomes saturated, and (4) correcting the un-correctable contours from ACR using ISS tool. The steps 1-3 are designed to be fully automated. Details on ACQA, ACR and ISS are being investigated in separate studies. Briefly, the ACQA module uses either machine learning or DL classification algorithms based on organ and image properties to validate contour quality. The ACR module utilizes multiple correction methods, including an active contour model (ACM), U-Net and Dense UNet DL models to sequentially refine the inaccurate contours with minor and/or major errors. The ISS tool uses the accurate contours on an adjacent slice as prior knowledge informant to guide a 2D UNet DL model to predict contours on a subsequent slice. We have demonstrated the performance of the multi-layer pipeline on complex abdominal organs on various MRIs. Results The ACQA models achieved AUC/accuracy of 0.95/0.89 for pancreas and 0.92/0.88 for stomach contours. The ACM model improved 54%/49% of bowels/pancreas contours from major into minor errors and the UNet/Dense UNet ACR model improved 43%/33% of the minor bowel/stomach contours to be acceptable. The ISS tool achieved DSC of 0.92, 0.91, 0.91, 0.87, and 0.87 for colon, small bowel, stomach, pancreas, and duodenum. The expected execution time of the pipeline on an auto-segmented MRI was within 5 minutes. Conclusion This proposed multi-layer pipeline can complement deep learning auto-segmentation and can improve the segmentation efficiency and accuracy. As part of our on-going efforts, the pipeline is being implemented as a post DLAS process, substantially speeding up daily recontouring in MRgART.
更多查看译文
AI 理解论文
溯源树
样例
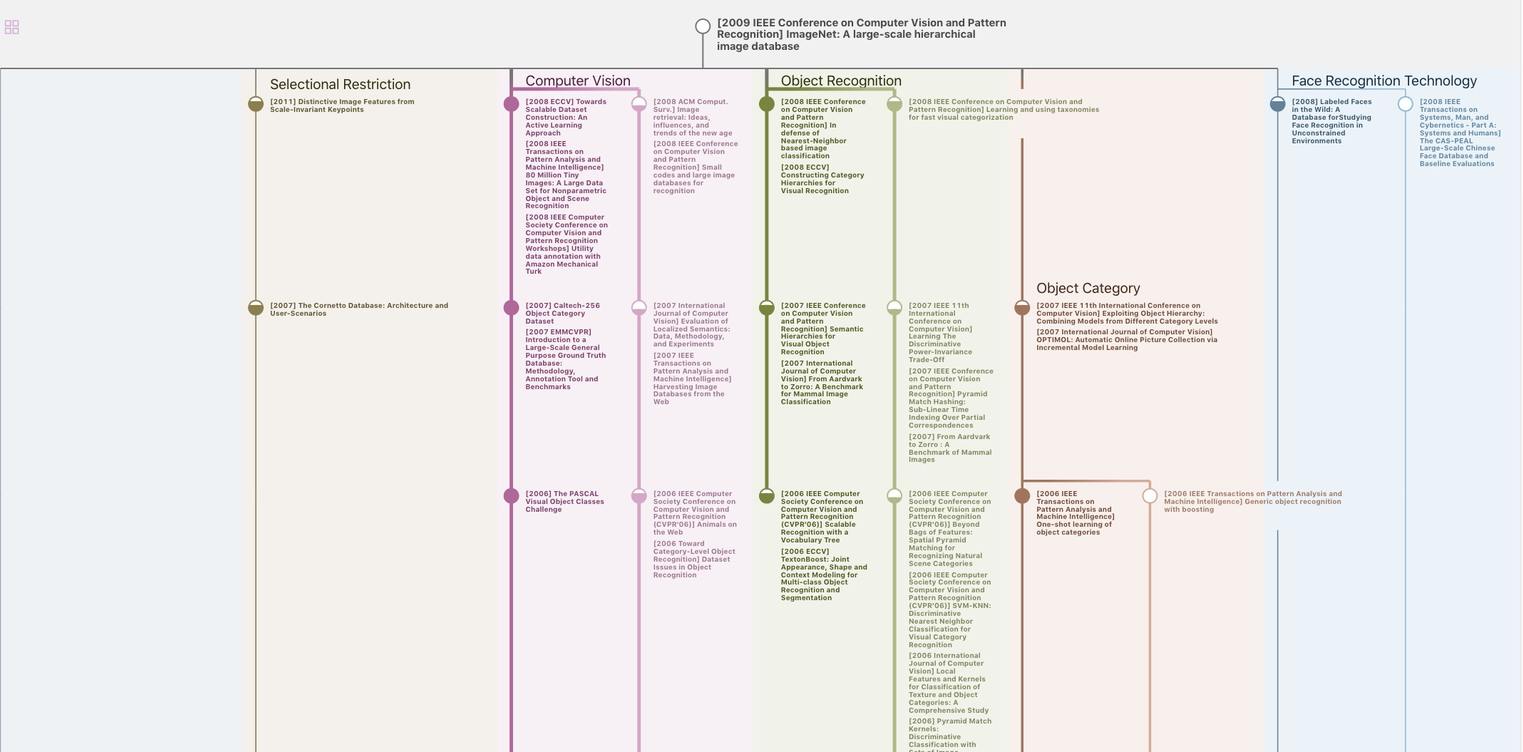
生成溯源树,研究论文发展脉络
Chat Paper
正在生成论文摘要