Refined probability distribution module for fine-grained visual categorization.
Neurocomputing(2023)
摘要
Fine-grained visual categorization is an important task in computer vision. Prior works on fine-grained visual categorization have paid much attention to addressing intra-class variation and inter-class similar-ity. However, they rarely study that task from the perspective of probability distribution. In this paper, we propose a novel refined probability distribution module based on deep convolutional neural network. Our module computes the probability of an image by fully utilizing the similarity information between images. Firstly, we use deep neural networks to obtain the initial probability distribution and extract fea-tures. Then, we build a network whose inputs are features for calculating image-to-image similarity scores. Finally, our module refines the initial probability distribution based on an effective batch random walk operation with similarity scores. Our module can be plugged into many deep convolutional neural networks. Experimental results show that our approach outperforms state-of-the-art methods on the CUB-200-2011, FGVC-Aircraft and Stanford Cars datasets respectively.CO 2022 Published by Elsevier B.V.
更多查看译文
关键词
Image -to -image similarity scores,Batch random walk,Deep learning,Fine-grained visual categorization
AI 理解论文
溯源树
样例
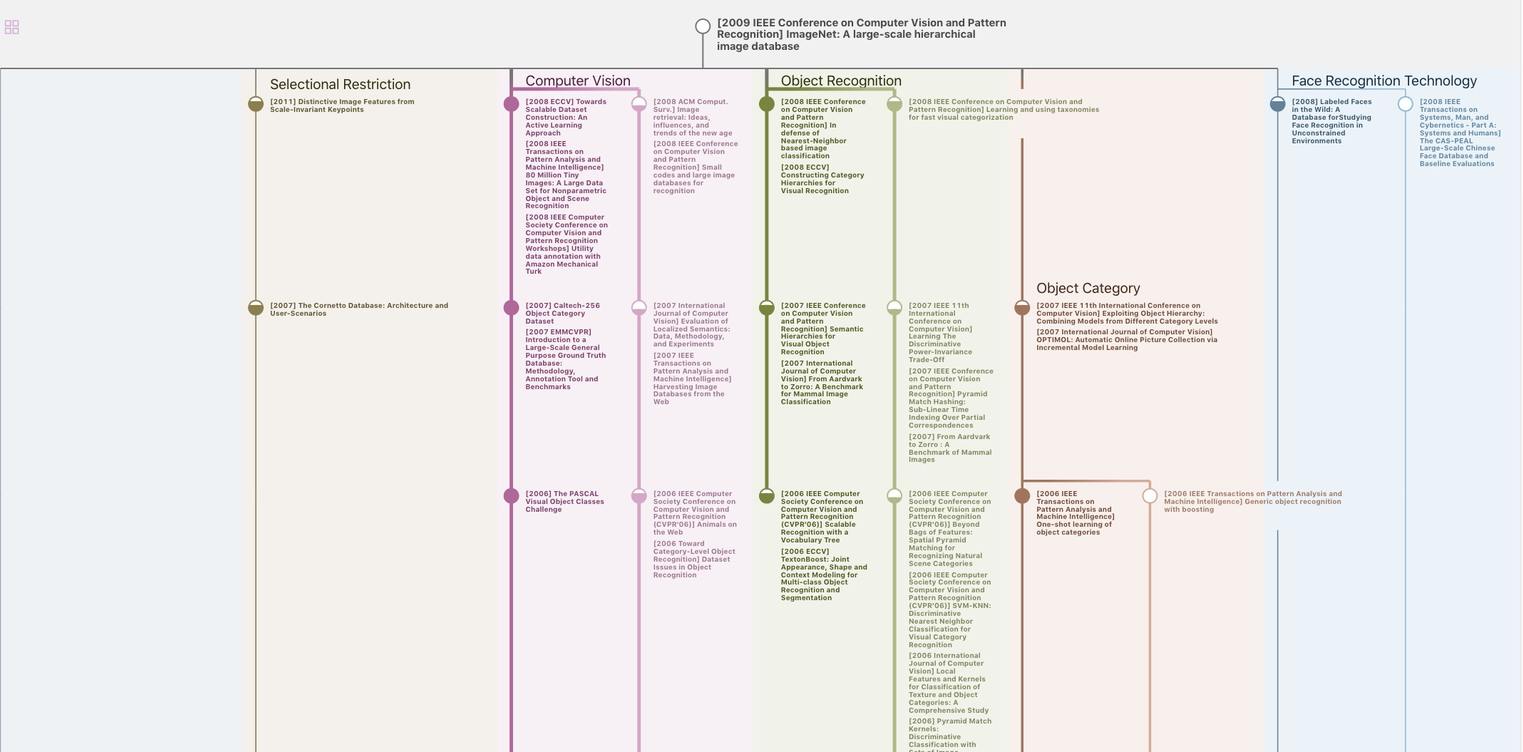
生成溯源树,研究论文发展脉络
Chat Paper
正在生成论文摘要