Using Machine Learning to Identify Novel Hydroclimate States
PHILOSOPHICAL TRANSACTIONS OF THE ROYAL SOCIETY A-MATHEMATICAL PHYSICAL AND ENGINEERING SCIENCES(2022)
关键词
machine learning,climate change,drought
AI 理解论文
溯源树
样例
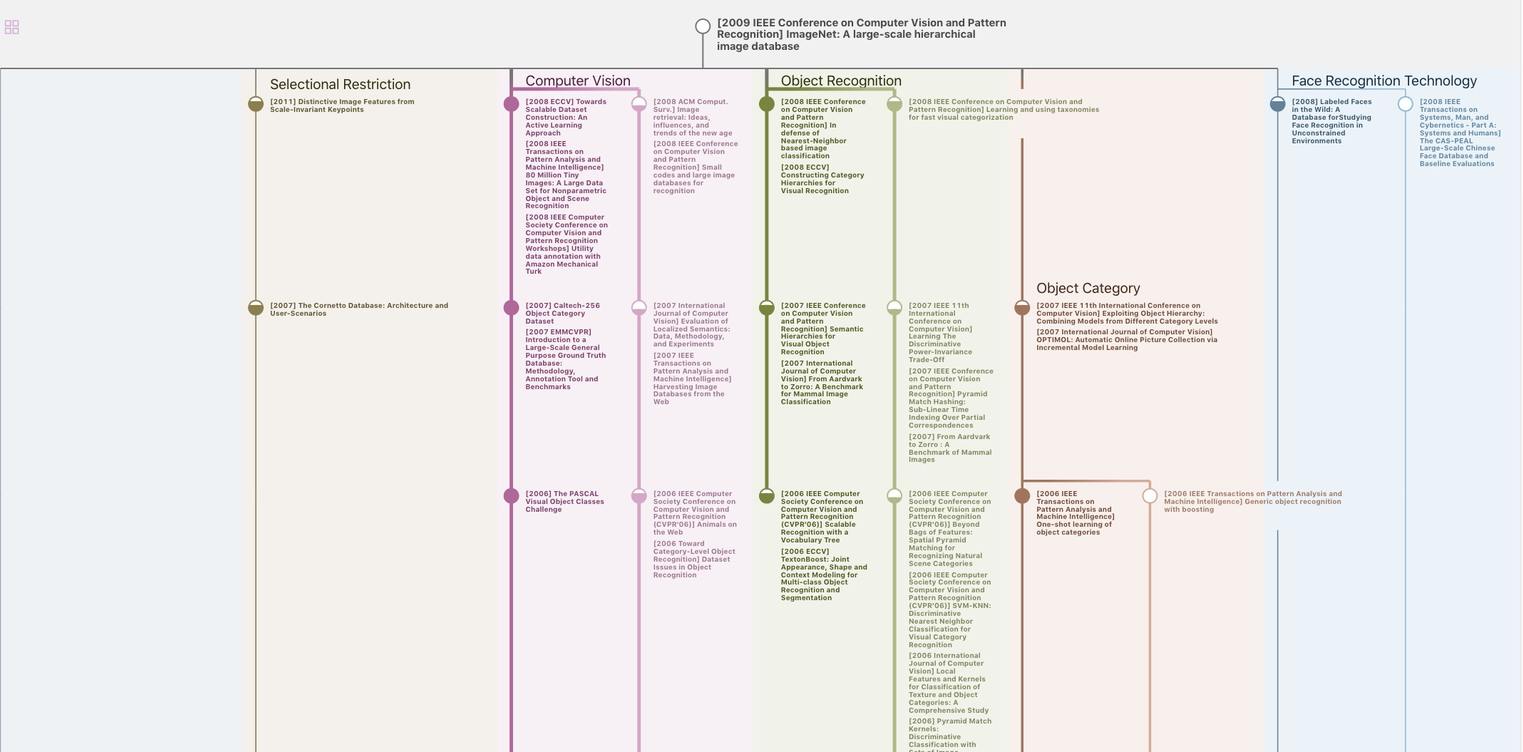
生成溯源树,研究论文发展脉络
Chat Paper
正在生成论文摘要