Inference and Prediction Using Functional Principal Components Analysis: Application to Diabetic Kidney Disease Progression in the Chronic Renal Insufficiency Cohort (CRIC) Study
arxiv(2022)
摘要
Repeated longitudinal measurements are commonly used to model long-term disease progression, and timing and number of assessments per patient may vary, leading to irregularly spaced and sparse data. Longitudinal trajectories may exhibit curvilinear patterns, in which mixed linear regression methods may fail to capture true trends in the data. We applied functional principal components analysis to model kidney disease progression via estimated glomerular filtration rate (eGFR) trajectories. In a cohort of 2641 participants with diabetes and up to 15 years of annual follow-up from the Chronic Renal Insufficiency Cohort (CRIC) study, we detected novel dominant modes of variation and patterns of diabetic kidney disease (DKD) progression among subgroups defined by the presence of albuminuria. We conducted inferential permutation tests to assess differences in longitudinal eGFR patterns between groups. To determine whether fitting a full cohort model or separate group-specific models is more optimal for modeling long-term trajectories, we evaluated model fit, using our goodness-of-fit procedure, and future prediction accuracy. Our findings indicated advantages for both modeling approaches in accomplishing different objectives. Beyond DKD, the methods described are applicable to other settings with longitudinally assessed biomarkers as indicators of disease progression. Supplementary materials for this article are available online.
更多查看译文
关键词
functional principal components analysis
AI 理解论文
溯源树
样例
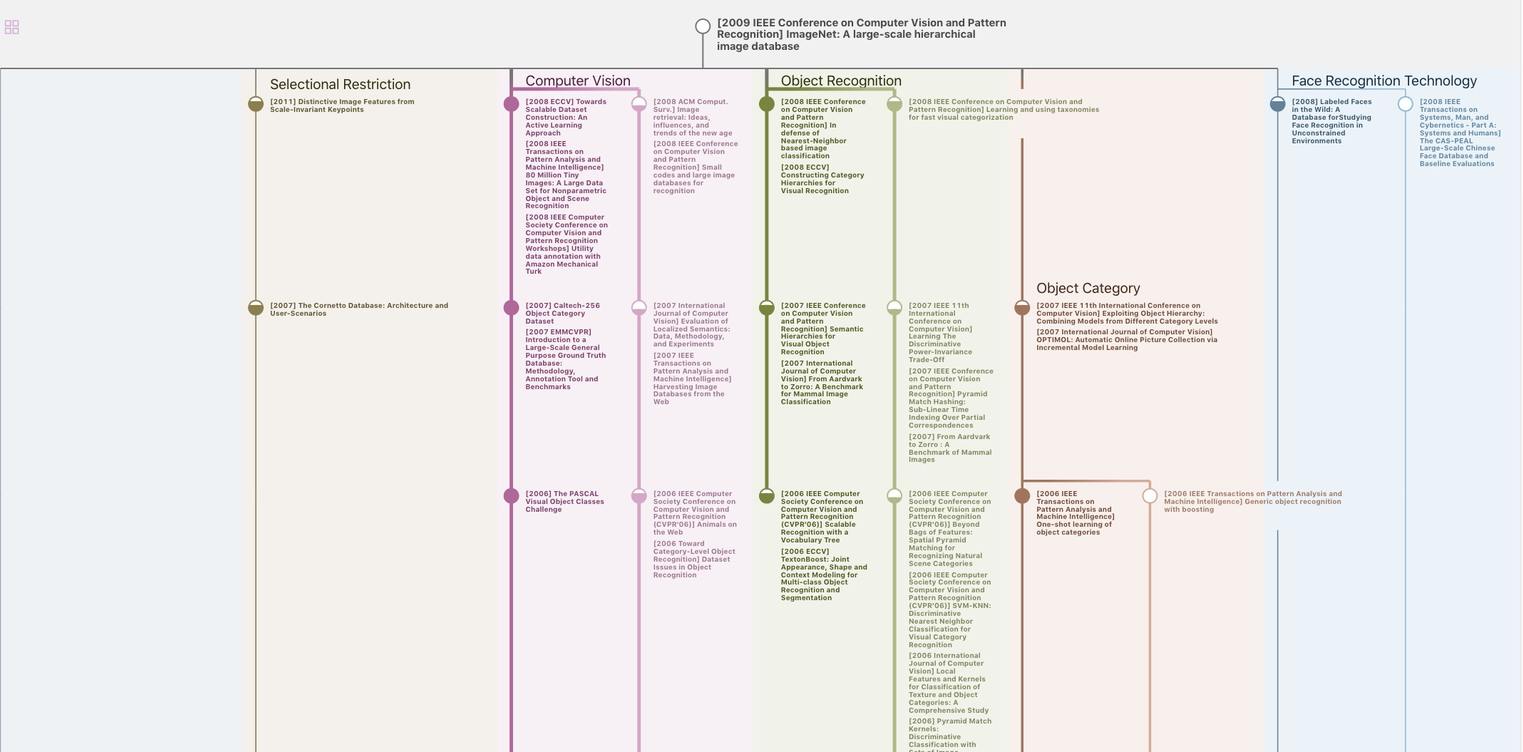
生成溯源树,研究论文发展脉络
Chat Paper
正在生成论文摘要