Model-based Lifelong Reinforcement Learning with Bayesian Exploration
NeurIPS 2022(2022)
摘要
We propose a model-based lifelong reinforcement-learning approach that estimates a hierarchical Bayesian posterior distilling the common structure shared across different tasks. The learned posterior combined with a sample-based Bayesian exploration procedure increases the sample efficiency of learning across a family of related tasks. We first derive an analysis of the relationship between the sample complexity and the initialization quality of the posterior in the finite MDP setting. We next scale the approach to continuous-state domains by introducing a Variational Bayesian Lifelong Reinforcement Learning algorithm that can be combined with recent model-based deep RL methods, and that exhibits backward transfer. Experimental results on several challenging domains show that our algorithms achieve both better forward and backward transfer performance than state-of-the-art lifelong RL methods.
更多查看译文
关键词
Deep Reinforcement Learning,Lifelong Reinforcement Learning
AI 理解论文
溯源树
样例
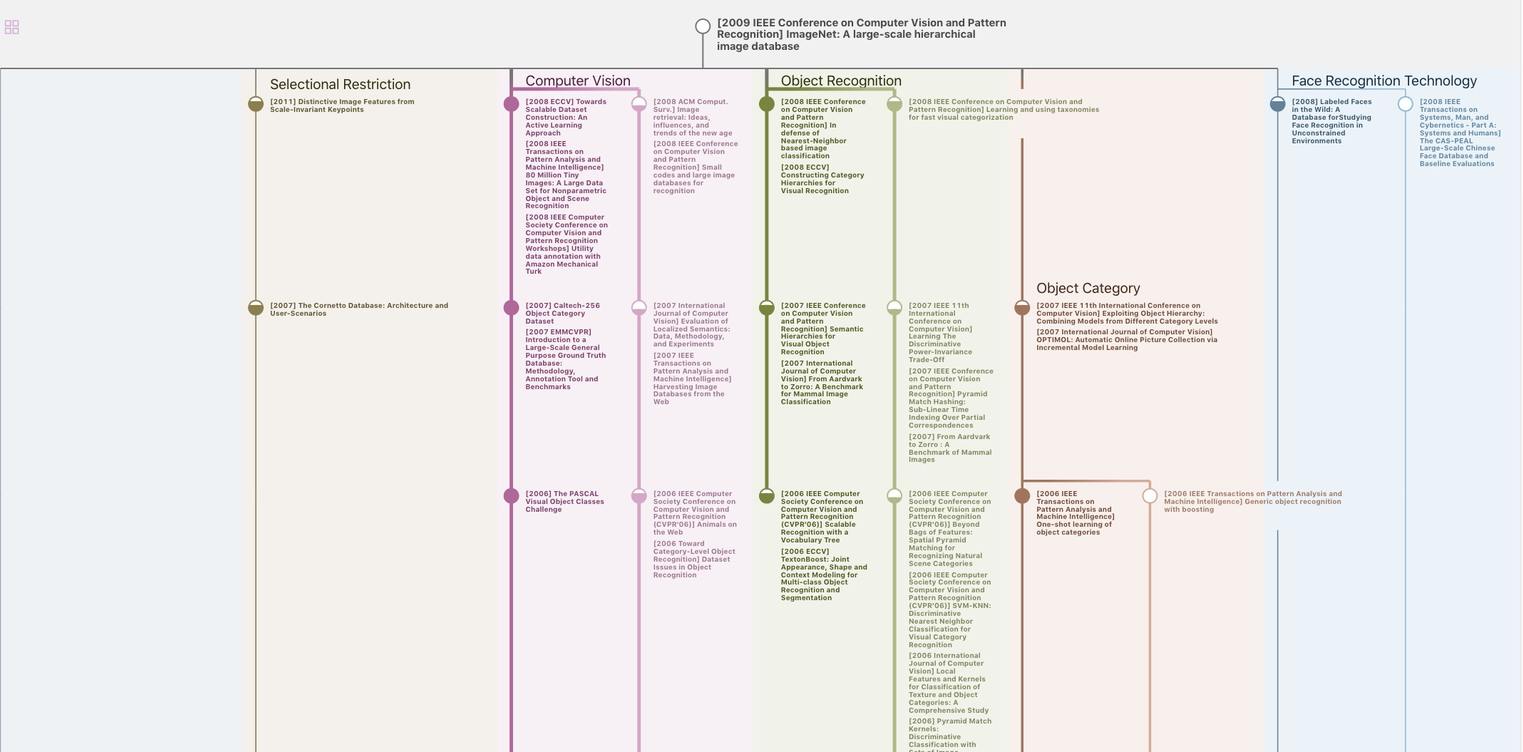
生成溯源树,研究论文发展脉络
Chat Paper
正在生成论文摘要