An Exploration of Data Efficiency in Intra-Dataset Task Transfer for Dialog Understanding
CoRR(2022)
摘要
Transfer learning is an exciting area of Natural Language Processing that has the potential to both improve model performance and increase data efficiency. This study explores the effects of varying quantities of target task training data on sequential transfer learning in the dialog domain. We hypothesize that a model can utilize the information learned from a source task to better learn a target task, thereby reducing the number of target task training samples required. Unintuitively, our data shows that often target task training data size has minimal effect on how sequential transfer learning performs compared to the same model without transfer learning. Our results lead us to believe that this unexpected result could be due to the effects of catastrophic forgetting, motivating further work into methods that prevent such forgetting.
更多查看译文
关键词
dialog understanding,data efficiency,task,intra-dataset
AI 理解论文
溯源树
样例
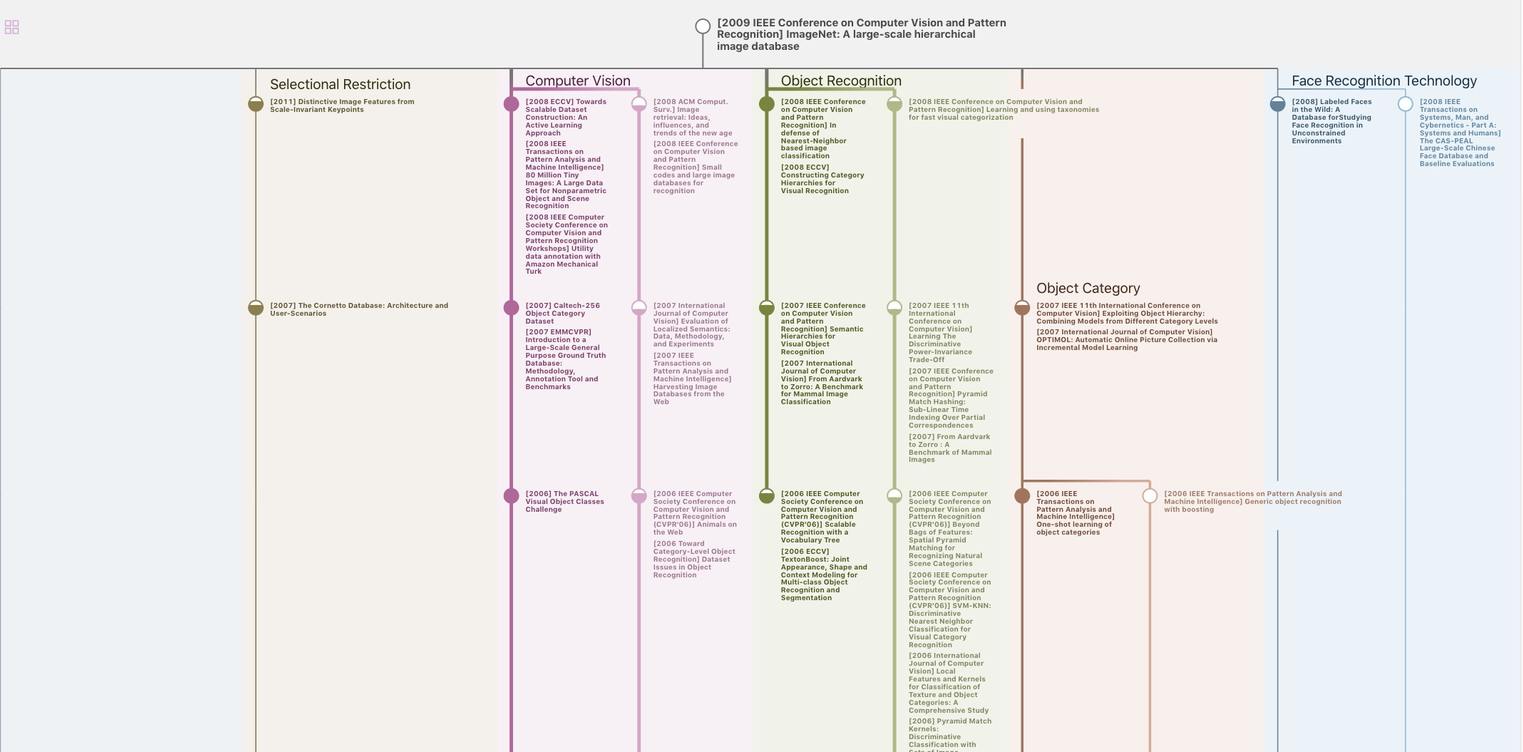
生成溯源树,研究论文发展脉络
Chat Paper
正在生成论文摘要