Data-Driven Quickest Change Detection in Markov Models
arxiv(2022)
摘要
The problem of quickest change detection in Markov models is studied. A sequence of samples are generated from a Markov model, and at some unknown time, the transition kernel of the Markov model changes. The goal is to detect the change as soon as possible subject to false alarm constraints. The data-driven setting is investigated, where neither the pre- nor the post-change Markov transition kernel is known. A kernel based data-driven algorithm is developed, which applies to general state space and is recursive and computationally efficient. Performance bounds on the average running length and worst-case average detection delay are derived. Numerical results are provided to validate the performance of the proposed algorithm.
更多查看译文
关键词
Sequential Change Detection,Maximum Mean Discrepancy,Computationally Efficient,CuSum-Type Test,Second-Order Markov Chain
AI 理解论文
溯源树
样例
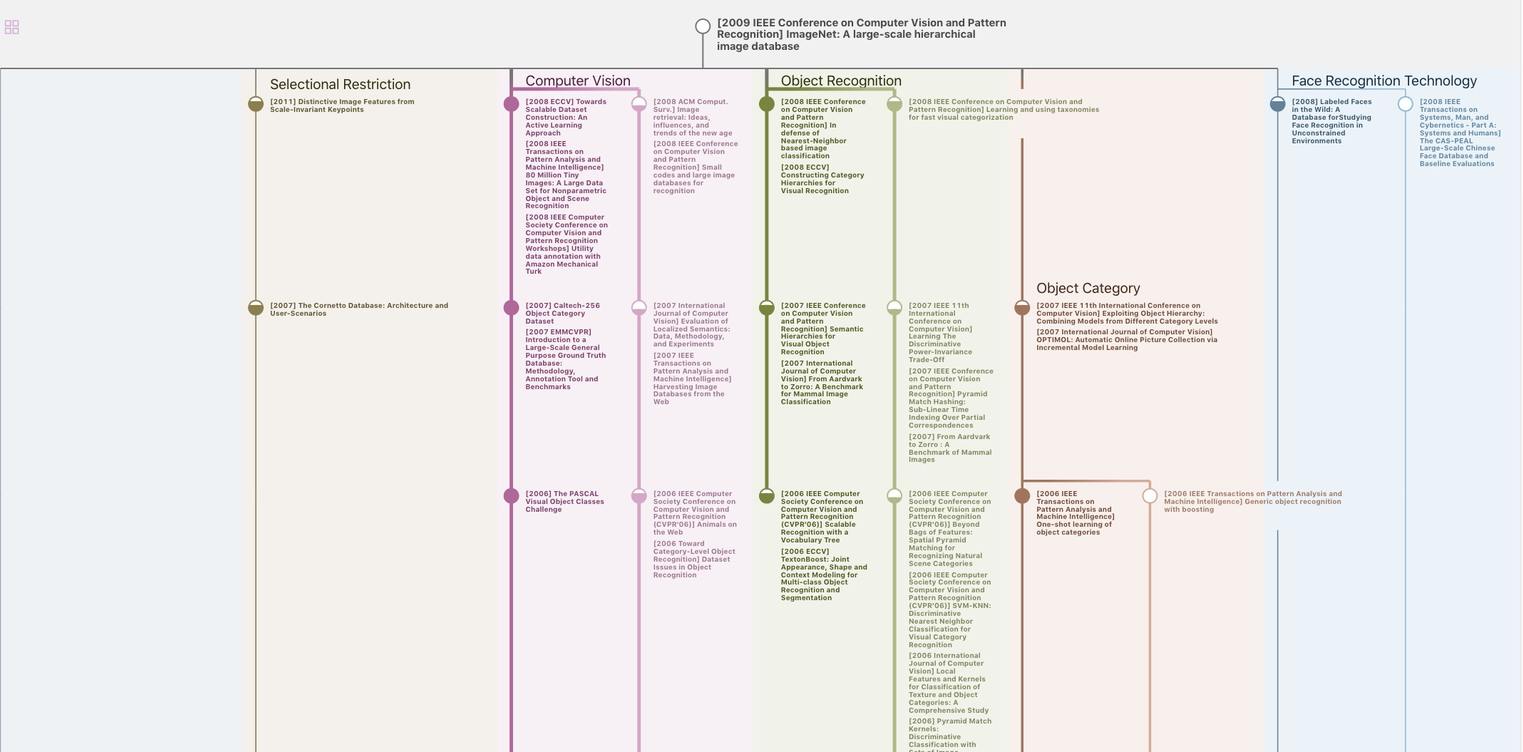
生成溯源树,研究论文发展脉络
Chat Paper
正在生成论文摘要