Fixed-time neural network control of a robotic manipulator with input deadzone
ISA transactions(2023)
摘要
In this paper, a fixed-time control method is proposed for an uncertain robotic system with actuator saturation and constraints that occur a period of time after the system operation. A model-based control and a neural network-based learning approach are proposed under the framework of fixed-time convergence, respectively. We use neural networks to handle the uncertainty, and design an adaptive law driven by approximation errors to compensate the input deadzone. In addition, a new structure of stabilizing function combining with an error shifting function is introduced to demonstrate the robotic system stability and the boundedness of all error signals. It is proved that all the tracking errors converge into the compact sets near zero in fixed-time according to the Lyapunov stability theory. Simulations on a two-joint robot manipulator and experiments on a six-joint robot manipulator verified the effectiveness of the proposed fixed-time control algorithm.(c) 2022 ISA. Published by Elsevier Ltd. All rights reserved.
更多查看译文
关键词
Fixed -time control,Adaptive neural networks,Input deadzone,Uncertainty,Robotic system
AI 理解论文
溯源树
样例
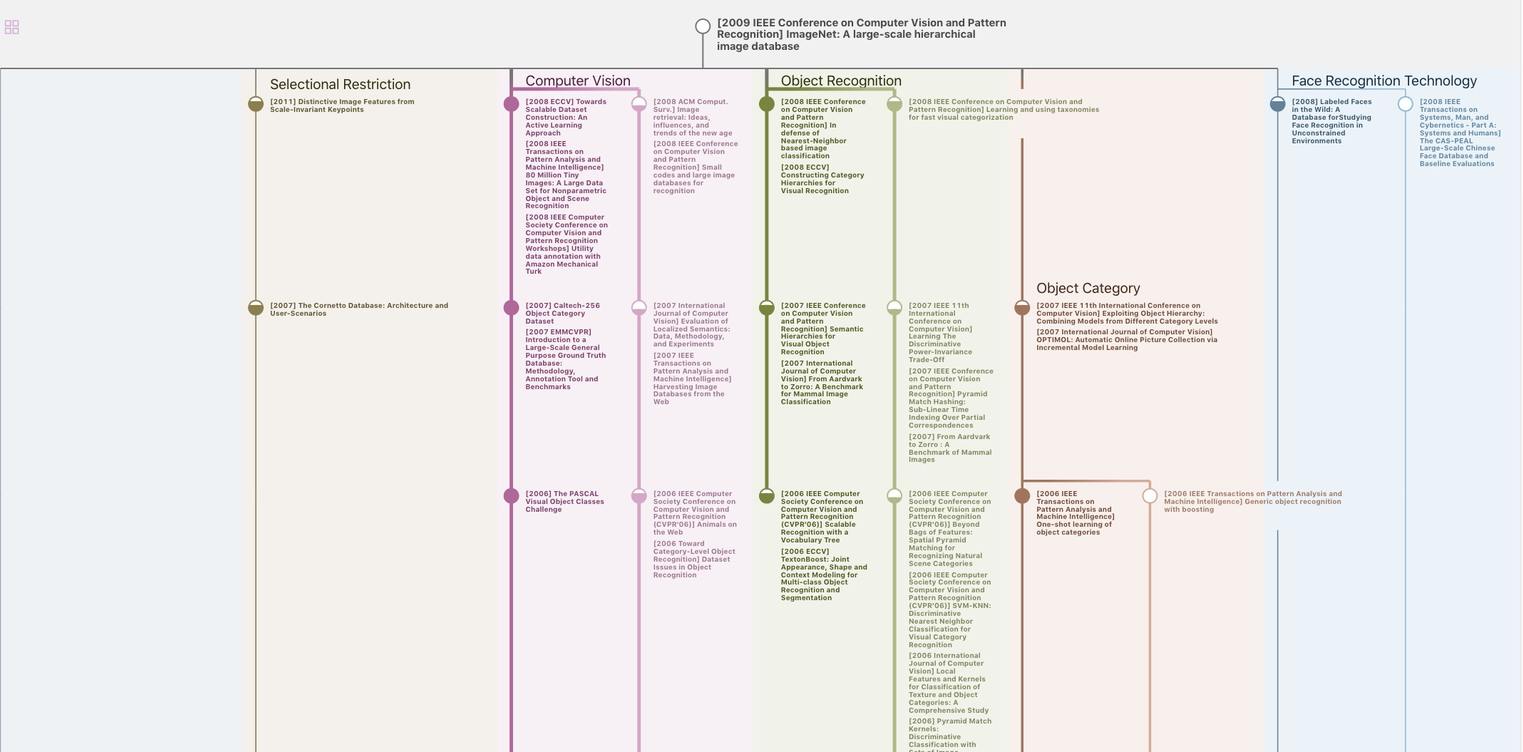
生成溯源树,研究论文发展脉络
Chat Paper
正在生成论文摘要