Hybrid sampling-based contrastive learning for imbalanced node classification
Int. J. Mach. Learn. Cybern.(2022)
摘要
Imbalanced node classification is a vital task because it widely exists in many real-world applications, such as financial fraud detection, anti-money laundering, drug reaction prediction and so on. However, many recent methods are for balanced graph-structured datasets, and do not perform well on imbalanced data. Therefore, we propose a hybrid sampling-based contrastive learning method (HSCL) for imbalanced node classification to alleviate this problem. The core of our method is to adopt the hybrid sampling method in contrastive learning, that is, undersampling majority classes and oversampling minority classes, to achieve a balance of samples from different classes in contrastive learning and thus obtain a discriminative representation. HSCL has been evaluated extensively on five real-world data sets. Experimental results show that the proposed method obtains better performance than other state-of-the-art methods.
更多查看译文
关键词
Imbalanced node classification,Contrastive learning,Hybrid sampling,Graph convolutional network
AI 理解论文
溯源树
样例
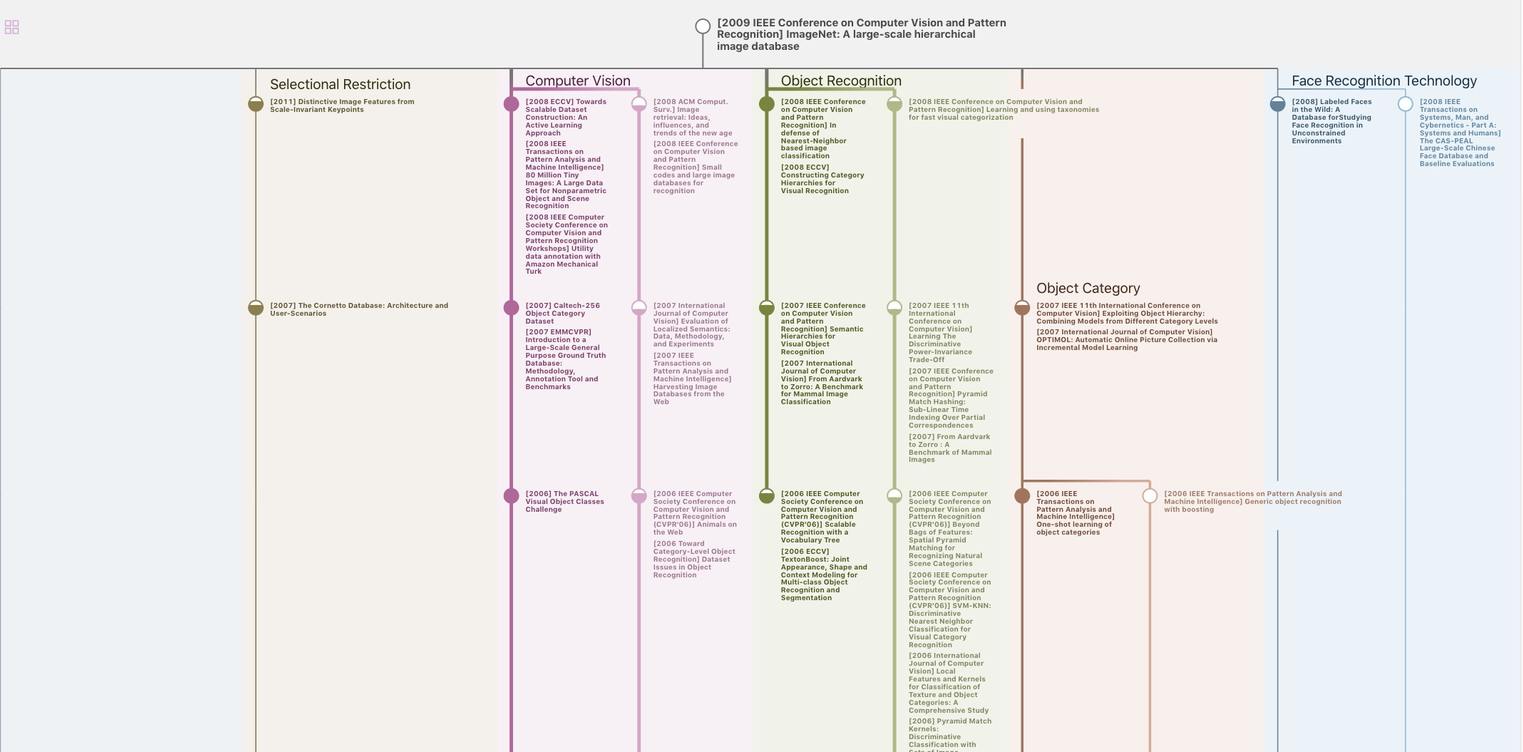
生成溯源树,研究论文发展脉络
Chat Paper
正在生成论文摘要