Machine learning assisted coarse-grained molecular dynamics modeling of meso-scale interfacial fluids.
The Journal of chemical physics(2023)
摘要
A hallmark of meso-scale interfacial fluids is the multi-faceted, scale-dependent interfacial energy, which often manifests different characteristics across the molecular and continuum scale. The multi-scale nature imposes a challenge to construct reliable coarse-grained (CG) models, where the CG potential function needs to faithfully encode the many-body interactions arising from the unresolved atomistic interactions and account for the heterogeneous density distributions across the interface. We construct the CG models of both single- and two-component polymeric fluid systems based on the recently developed deep coarse-grained potential [Zhang et al., J. Chem. Phys. 149, 034101 (2018)] scheme, where each polymer molecule is modeled as a CG particle. By only using the training samples of the instantaneous force under the thermal equilibrium state, the constructed CG models can accurately reproduce both the probability density function of the void formation in bulk and the spectrum of the capillary wave across the fluid interface. More importantly, the CG models accurately predict the volume-to-area scaling transition for the apolar solvation energy, illustrating the effectiveness to probe the meso-scale collective behaviors encoded with molecular-level fidelity.
更多查看译文
关键词
molecular dynamics modeling,interfacial fluids,coarse-grained,meso-scale
AI 理解论文
溯源树
样例
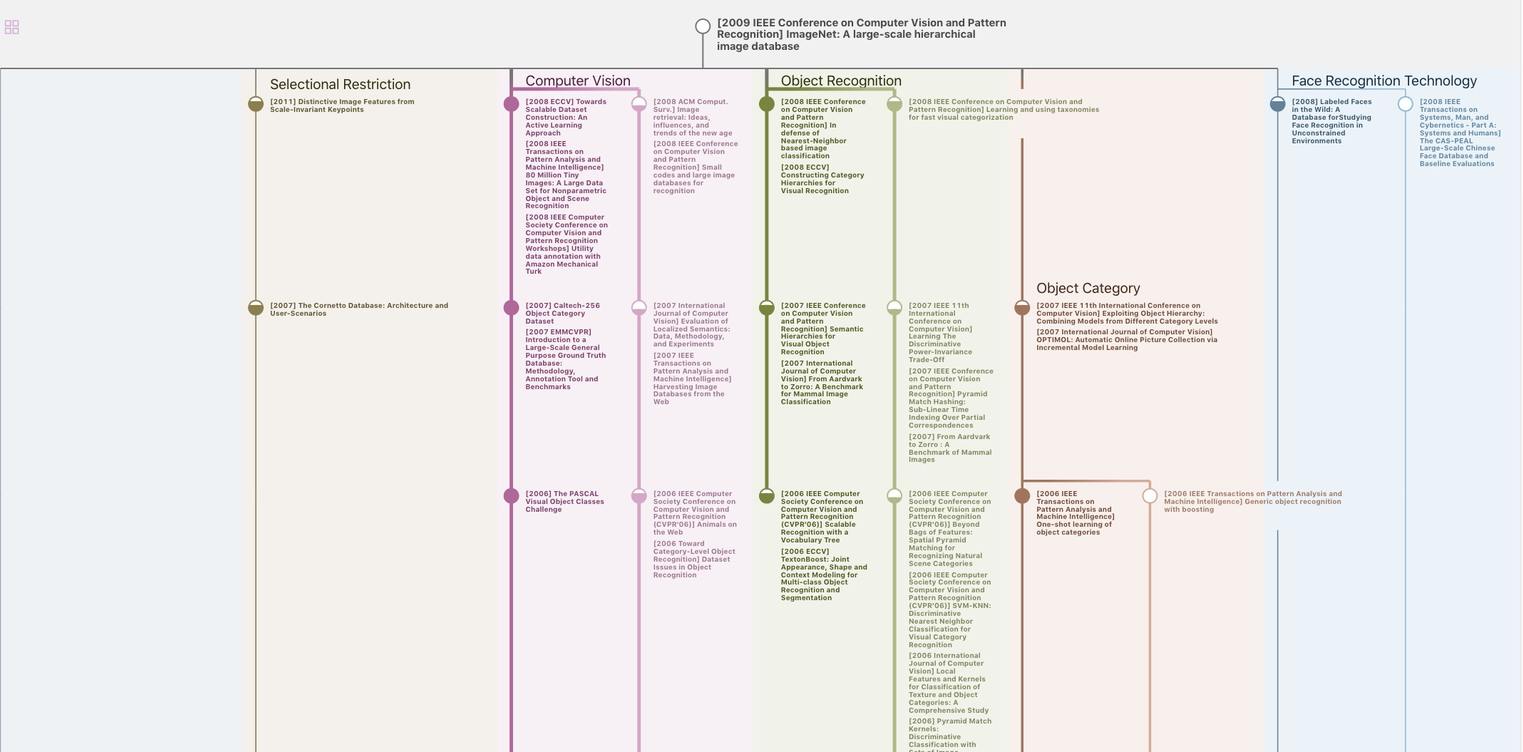
生成溯源树,研究论文发展脉络
Chat Paper
正在生成论文摘要