SpikeSim: An End-to-End Compute-in-Memory Hardware Evaluation Tool for Benchmarking Spiking Neural Networks
arxiv(2023)
摘要
Spiking neural networks (SNNs) are an active research domain toward energy-efficient machine intelligence. Compared to conventional artificial neural networks (ANNs), SNNs use temporal spike data and bio-plausible neuronal activation functions such as leaky-integrate fire/integrate fire (LIF/IF) for data processing. However, SNNs incur significant dot-product operations causing high memory and computation overhead in standard von-Neumann computing platforms. To this end, in memory computing (IMC) architectures have been proposed to alleviate the "memory-wall bottleneck" prevalent in von Neumann architectures. Although recent works have proposed IMC-based SNN hardware accelerators, the following key implementation aspects have been overlooked: 1) the adverse effects of crossbar nonideality on SNN performance due to repeated analog dot-product operations over multiple time-steps and 2) hardware overheads of essential SNN-specific components, such as the LIF/IF and data communication modules. To this end, we propose SpikeSim, a tool that can perform realistic performance, energy, latency and area evaluation of IMC-mapped SNNs. SpikeSim consists of a practical monolithic IMC architecture called SpikeFlow for mapping SNNs. Additionally, the nonideality computation engine (NICE) and energy-latency-area (ELA) engine performs hardware-realistic evaluation of SpikeFlow-mapped SNNs. Based on 65nm CMOS implementation and experiments on CIFAR10, CIFAR100 and TinyImagenet datasets, we find that the LIF/IF neuronal module has significant area contribution (> 11% of the total hardware area). To this end, we propose SNN topological modifications that leads to 1.24x and 10x reduction in the neuronal module's area and the overall energy-delay-product value, respectively. Furthermore, in this work, we perform a holistic comparison between IMC implemented ANN and SNNs and conclude that lower number of time-steps are the key to achieve higher throughput and energy-efficiency for SNNs compared to 4-bit ANNs. The code repository for the SpikeSim tool is available at Github link.
更多查看译文
关键词
spiking neural networks,hardware,end-to-end,compute-in-memory
AI 理解论文
溯源树
样例
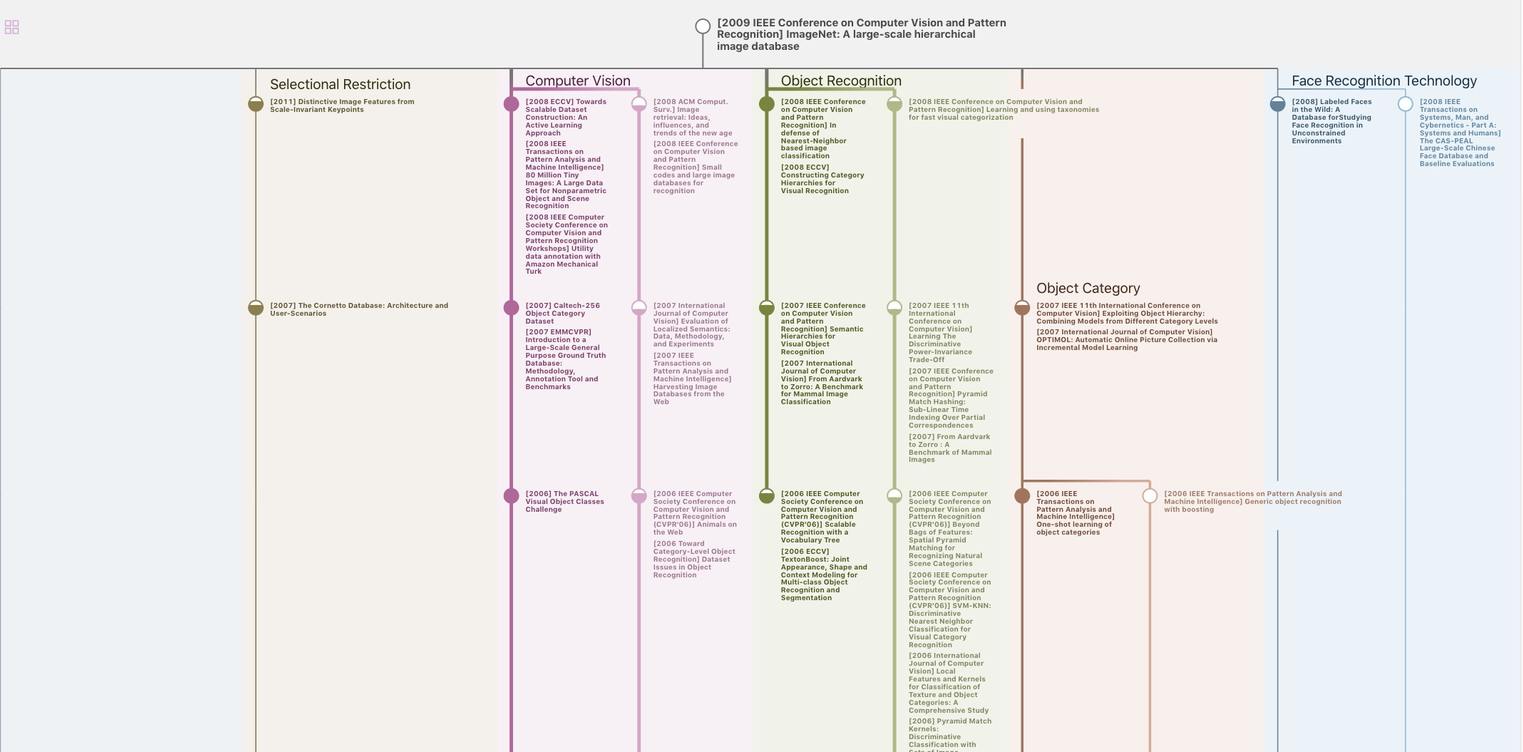
生成溯源树,研究论文发展脉络
Chat Paper
正在生成论文摘要