A Regularized Conditional GAN for Posterior Sampling in Inverse Problems
arxiv(2023)
摘要
In inverse problems, one seeks to reconstruct an image from incomplete and/or degraded measurements. Such problems arise in magnetic resonance imaging (MRI), computed tomography, deblurring, super-resolution, inpainting, and other applications. Given a training set of signal/measurement pairs, we design a method to generate posterior samples rapidly and accurately. In particular, we propose a regularized conditional Wasserstein GAN that generates dozens of high-quality posterior samples per second. Our regularization comprises an L1 penalty and an adaptively weighted standard-deviation reward. Using quantitative evaluation metrics like conditional Frechet inception distance, we demonstrate that our method produces state-of-the-art posterior samples in both multicoil MRI and large-scale inpainting applications.
更多查看译文
AI 理解论文
溯源树
样例
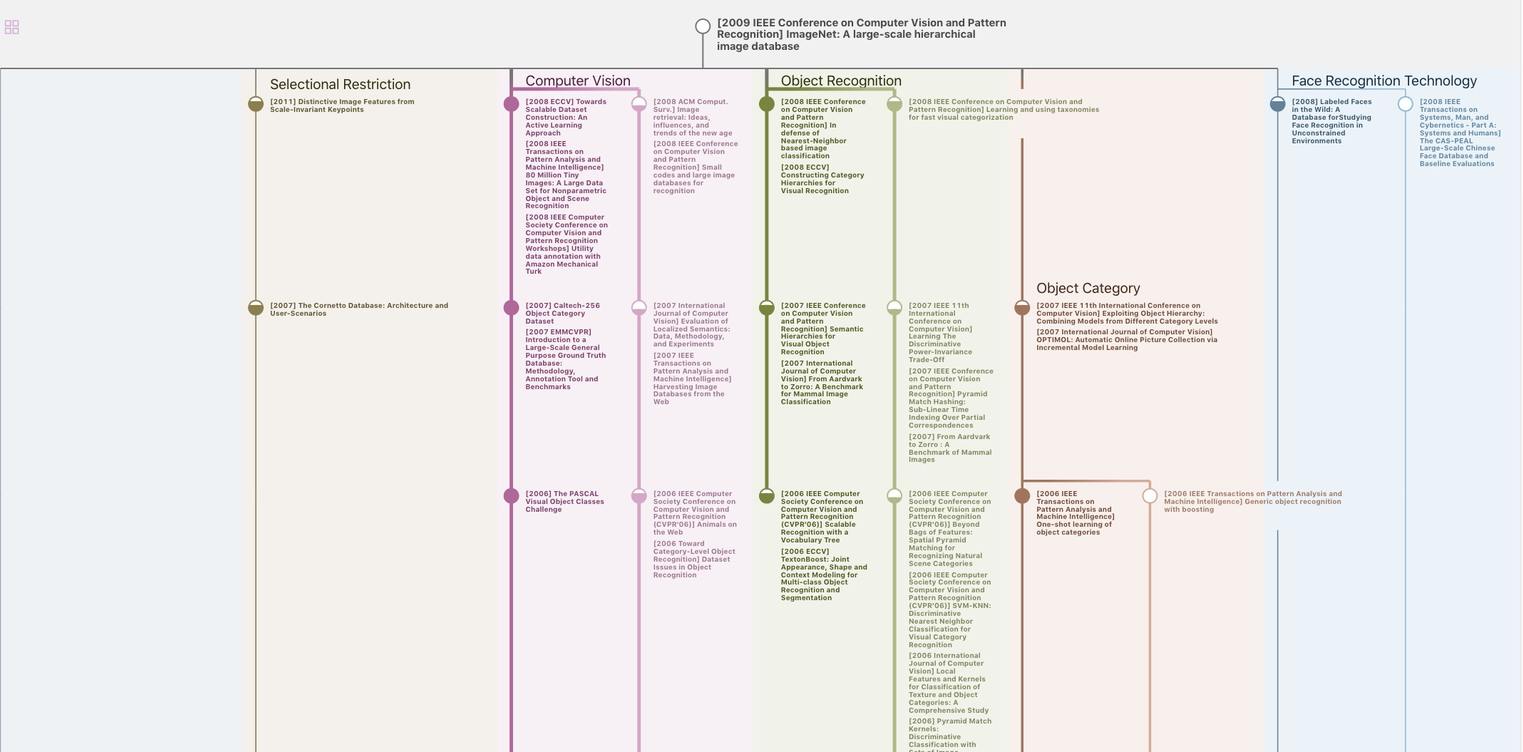
生成溯源树,研究论文发展脉络
Chat Paper
正在生成论文摘要