Data-Driven Stabilizing and Robust Control of Discrete-Time Linear Systems with Error in Variables
arxiv(2023)
摘要
This work presents a sum-of-squares (SOS) based framework to perform data-driven stabilization and robust control tasks on discrete-time linear systems where the full-state observations are corrupted by L-infinity bounded input, measurement, and process noise (error in variable setting). Certificates of state-feedback superstability, quadratic stability or positive stability of all plants in a consistency set are provided by solving a feasibility program formed by polynomial nonnegativity constraints. Under mild compactness and data-collection assumptions, SOS tightenings in rising degree will converge to recover the true superstabilizing or positive stabilizing controller, with some conservatism introduced for quadratic stabilizability. The performance of this SOS method is improved through the application of a theorem of alternatives while retaining tightness, in which the unknown noise variables are eliminated from the consistency set description. This SOS feasibility method is extended to provide worst-case-optimal robust controllers under H2 control costs. The consistency set description may be broadened to include cases where the data and process are affected by a combination of L-infinity bounded measurement, process, and input noise.
更多查看译文
关键词
robust control,linear
AI 理解论文
溯源树
样例
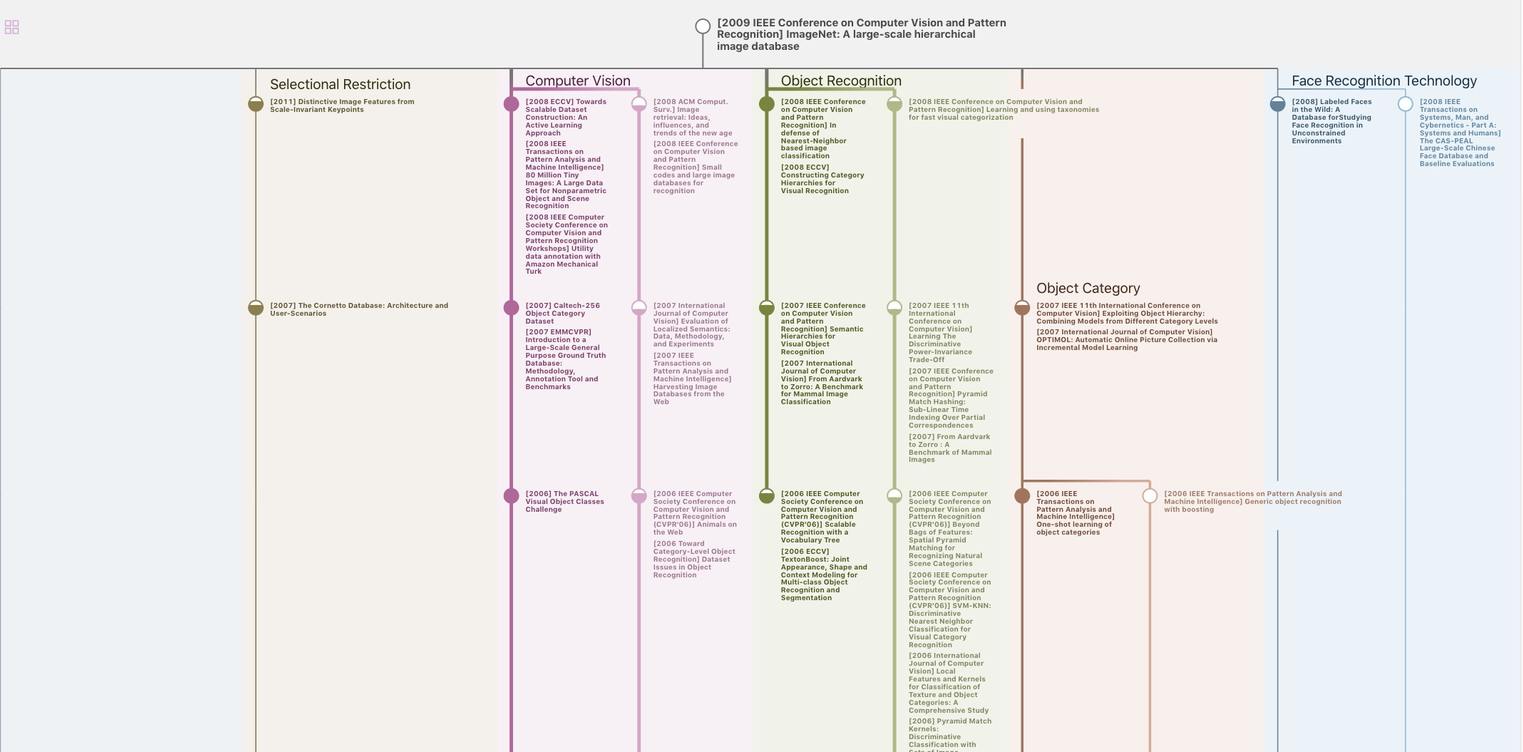
生成溯源树,研究论文发展脉络
Chat Paper
正在生成论文摘要