Road Extraction Based on Improved Convolutional Neural Networks with Satellite Images
Applied Sciences(2022)
摘要
Deep learning has been applied in various fields for its effective and accurate feature learning capabilities in recent years. Currently, information extracted from remote sensing images with the learning methods has become the most relevant research area for its developed precision. In terms of developing segmentation precision and reducing calculation power consumption, the improved deep learning methods have received more attention, and the improvement of semantic segmentation architectures has been a popular solution. This research presents a learning method named D-DenseNet with a new structure for road extraction. The methods for the improvement are divided into two stages: (1) alternate the consecutive dilated convolutions number in the structure of the network (2) the stem block is arranged as the initial block. So, dilated convolution can obtain more global context information through the whole network. Further, the D-DenseNet restructures D-LinkNet by taking DenseNet as its backbone instead of ResNet, which can expand the receptive field and accept more feature information. The D-DenseNet is effective because of its 119M model size and 57.96% IoU on the processing test data and 99.3M modes size and 66.26% on the public dataset, which achieved the research objective for reducing model size and developing segmentation precision—IoU. The experiment indicates that the D-Dense block and the stem block are effective for developing road extraction, and the appropriate number of convolution layers is also essential for model evaluation.
更多查看译文
关键词
semantic segmentation,low-level road,information extraction,deep learning
AI 理解论文
溯源树
样例
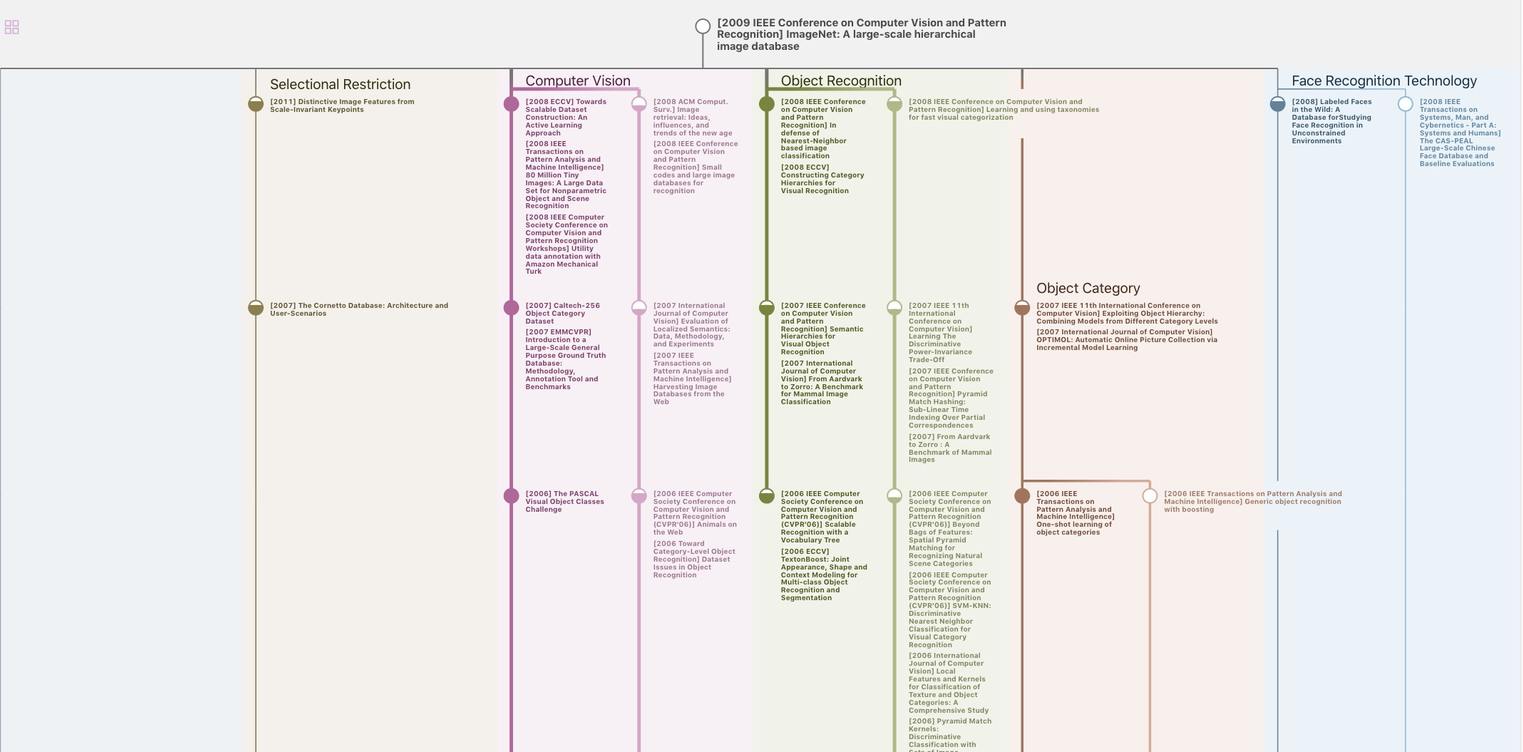
生成溯源树,研究论文发展脉络
Chat Paper
正在生成论文摘要