SCRDN: Residual dense network with self-calibrated convolutions for low dose CT image denoising
Nuclear Instruments and Methods in Physics Research Section A: Accelerators, Spectrometers, Detectors and Associated Equipment(2022)
摘要
Low-dose computed tomography (LDCT) can reduce the X-ray radiation dose that the patients receive, up to 86%, which decreases the potential hazards and expands its application scope. However, LDCT images contain a lot of noise and artifacts, which brings great difficulties to doctors’ diagnosis. Recently, methods based on deep learning have obtained great success in noise reducing of LDCT images. In this paper, we propose a novel residual dense network with self-calibrated convolution (SCRDN) for LDCT images denoising. Compared with the basic CNN, SCRDN includes jump connection, dense connection and self-calibrated convolution instead of traditional convolution. It makes full use of the hierarchical features of original images to obtain the reconstructed images with more details. It also obtains a larger receptive field without introducing new parameters. The experimental results show that the proposed method can achieve performance improvements over most state-of-the-art methods used in CT denoising.
更多查看译文
关键词
Dense network,Image denoising,Low-dose computed tomography (LDCT),Self-calibrated convolution
AI 理解论文
溯源树
样例
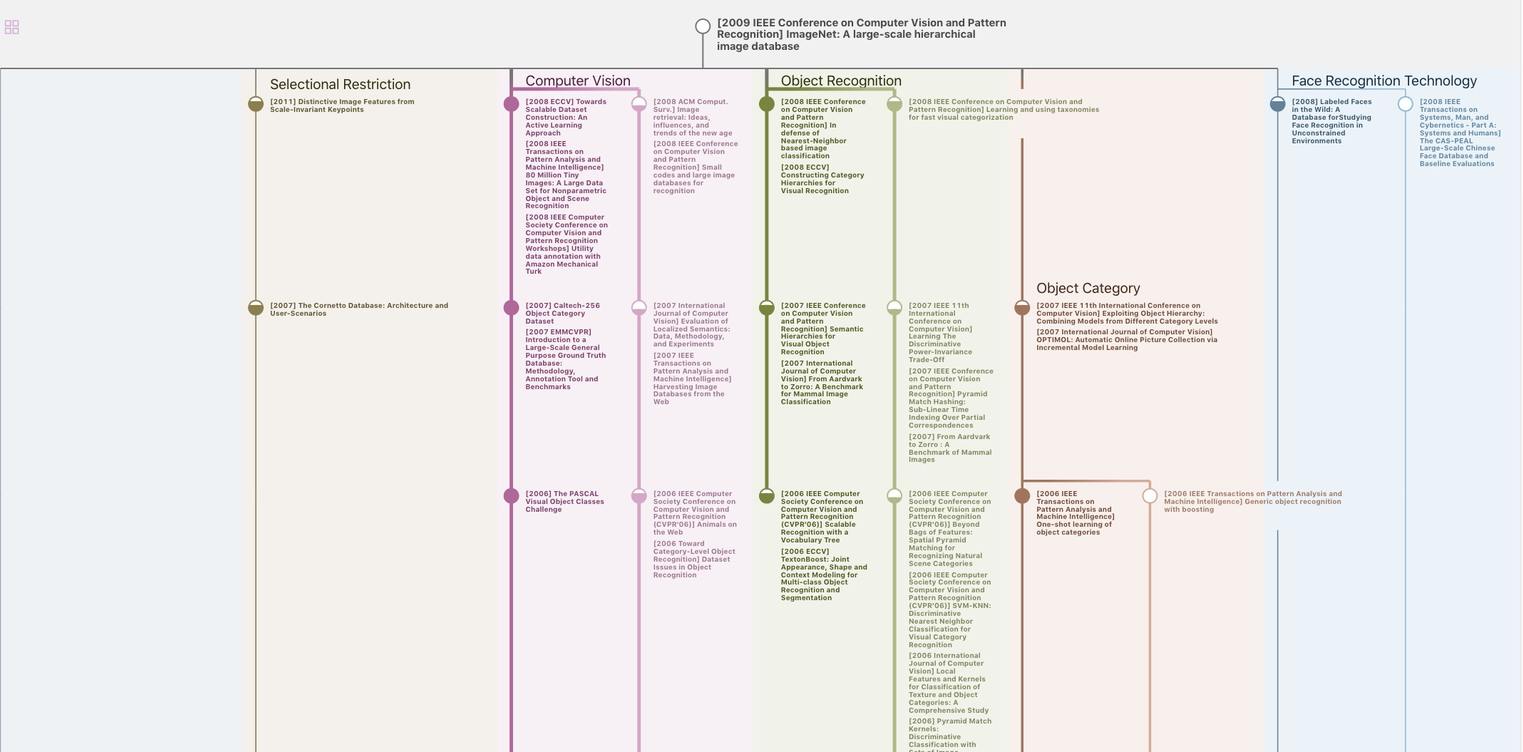
生成溯源树,研究论文发展脉络
Chat Paper
正在生成论文摘要