Deep Learning Enabled Label-free Cell Force Computation in Deformable Fibrous Environments
biorxiv(2022)
摘要
Through force exertion, cells actively engage with their immediate fibrous extracellular matrix (ECM) environment, causing dynamic remodeling of the environment and influencing cellular shape and contractility changes in a feedforward loop. Controlling cell shapes and quantifying the force-driven dynamic reciprocal interactions in a label-free setting is vital to understand cell behavior in fibrous environments but currently unavailable. Here, we introduce a force measurement platform termed crosshatch nanonet force microscopy (cNFM) that reveals new insights into cell shape-force coupling. Using a suspended crosshatch network of fibers capable of recovering in vivo cell shapes, we utilize deep learning methods to circumvent the fiduciary fluorescent markers required to recognize fiber intersections. Our method provides high fidelity computer reconstruction of different fiber architectures by automatically translating phase-contrast time-lapse images into synthetic fluorescent images. An inverse problem based on the nonlinear mechanics of fiber networks is formulated to match the network deformation and deformed fiber shapes to estimate the forces. We reveal an order-of-magnitude force changes associated with cell shape changes during migration, forces during cell-cell interactions and force changes as single mesenchymal stem cells undergo differentiation. Overall, deep learning methods are employed in detecting and tracking highly compliant backgrounds to develop an automatic and label-free force measurement platform to describe cell shape-force coupling in fibrous environments that cells would likely interact with in vivo.
### Competing Interest Statement
The authors have declared no competing interest.
更多查看译文
关键词
force,cell,deep learning,label-free
AI 理解论文
溯源树
样例
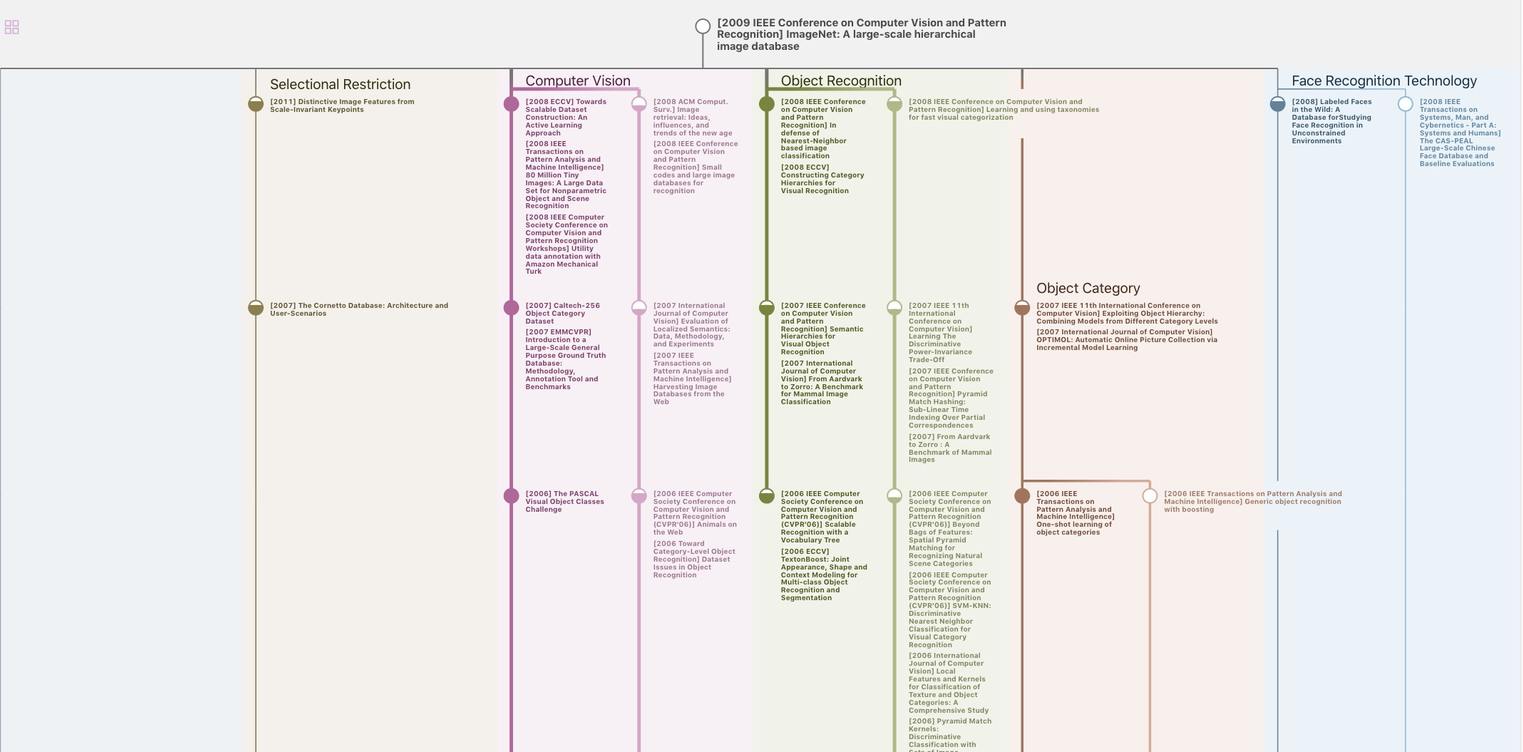
生成溯源树,研究论文发展脉络
Chat Paper
正在生成论文摘要